Thread: Using quicksort for every external sort run
I'll start a new thread for this, since my external sorting patch has now evolved well past the original "quicksort with spillover" idea...although not quite how I anticipated it would. It seems like I've reached a good point to get some feedback. I attach a patch series featuring a new, more comprehensive approach to quicksorting runs during external sorts. What I have now still includes "quicksort with spillover", but it's just a part of a larger project. I am quite happy with the improvements in performance shown by my testing, which I go into below. Controversy ========= A few weeks ago, I did not anticipate that I'd propose that replacement selection sort be used far less (only somewhat less, since I was only somewhat doubtful about the algorithm at the time). I had originally planned on continuing to *always* use it for the first run, to make "quicksort with spillover" possible (thereby sometimes avoiding significant I/O by not spilling most tuples), but also to make cases always considered sympathetic to replacement selection continue to happen. I thought that second or subsequent runs could still be quicksorted, but that I must still care about this latter category, the traditional sympathetic cases. This latter category is mostly just one important property of replacement selection: even without a strong logical/physical correlation, the algorithm tends to produce runs that are about twice the size of work_mem. (It's also notable that replacement selection only produces one run with mostly presorted input, even where input far exceeds work_mem, which is a neat trick.) I wanted to avoid controversy, but the case for the controversy is too strong for me to ignore: despite these upsides, replacement selection is obsolete, and should usually be avoided. Replacement selection sort still has a role to play in making "quicksort with spillover" possible (when a sympathetic case is *anticipated*), but other than that it seems generally inferior to a simple hybrid sort-merge strategy on modern hardware. By modern hardware, I mean anything manufactured in at least the last 20 years. We've already seen that the algorithm's use of a heap works badly with modern CPU caches, but that is just one factor contributing to its obsolescence. The big selling point of replacement selection sort in the 20th century was that it sometimes avoided multi-pass sorts as compared to a simple sort-merge strategy (remember when tuplesort.c always used 7 tapes? When you need to use 7 actual magnetic tapes, rewinding is expensive and in general this matters a lot!). We all know that memory capacity has grown enormously since then, but we must also consider another factor: At the same time, a simple hybrid sort-merge strategy's capacity to more or less get the important detail here right -- to avoid a multi-pass sort -- has increased quadratically (relative to work_mem/memory capacity). As an example, testing shows that for a datum tuplesort that requires about 2300MB of work_mem to be completed as a simple internal sort this patch only needs 30MB to just do one pass (see benchmark query below). I've mostly regressed that particular property of tuplesort (it used to be less than 30MB), but that's clearly the wrong thing to worry about for all kinds of reasons, probably even in the unimportant cases now forced to do multiple passes. Multi-pass sorts --------------------- I believe, in general, that we should consider a multi-pass sort to be a kind of inherently suspect thing these days, in the same way that checkpoints occurring 5 seconds apart are: not actually abnormal, but something that we should regard suspiciously. Can you really not afford enough work_mem to only do one pass? Does it really make sense to add far more I/O and CPU costs to avoid that other tiny memory capacity cost? In theory, the answer could be "yes", but it seems highly unlikely. Not only is very little memory required to avoid a multi-pass merge step, but as described above the amount required grows very slowly relative to linear growth in input. I propose to add a checkpoint_warning style warning (with a checkpoint_warning style GUC to control it). ISTM that these days, multi-pass merges are like saving $2 on replacing a stairwell light bulb, at the expense of regularly stumbling down the stairs in the dark. It shouldn't matter if you have a 50 terabyte decision support database or if you're paying Heroku a small monthly fee to run a database backing your web app: simply avoiding multi-pass merges is probably always the most economical solution, and by a wide margin. Note that I am not skeptical of polyphase merging itself, even though it is generally considered to be a complimentary technique to replacement selection (some less formal writing on external sorting seemingly fails to draw a sharp distinction). Nothing has changed there. Patch, performance =============== Let's focus on a multi-run sort, that does not use "quicksort with spillover", since that is all new, and is probably the most compelling case for very large databases with hundreds of gigabytes of data to sort. I think that this patch requires a machine with more I/O bandwidth than my laptop to get a proper sense of the improvement made. I've been using a tmpfs temp_tablespace for testing, to simulate this. That may leave me slightly optimistic about I/O costs, but you can usually get significantly more sequential I/O bandwidth by adding additional disks, whereas you cannot really buy new hardware to improve the situation with excessive CPU cache misses. Benchmark --------------- -- Setup, 100 million tuple table with high cardinality int4 column (2 billion possible int4 values) create table big_high_cardinality_int4 as select (random() * 2000000000)::int4 s, 'abcdefghijlmn'::text junk from generate_series(1, 100000000); -- Make cost model hinting accurate: analyze big_high_cardinality_int4; checkpoint; Let's start by comparing an external sort that uses 1/3 the memory of an internal sort against the master branch. That's completely unfair on the patch, of course, but it is a useful indicator of how well external sorts do overall. Although an external sort surely cannot be as fast as an internal sort, it might be able to approach an internal sort's speed when there is plenty of I/O bandwidth. That's a good thing to aim for, I think. -- Master (just enough memory for internal sort): set work_mem = '2300MB'; select count(distinct(s)) from big_high_cardinality; ***** Runtime after stabilization: ~33.6 seconds ***** -- Patch series, but with just over 1/3 the memory: set work_mem = '800MB'; select count(distinct(s)) from big_high_cardinality; ***** Runtime after stabilization: ~37.1 seconds ***** The patch only takes ~10% more time to execute this query, which seems very good considering that ~1/3 the work_mem has been put to use. trace_sort output for patch during execution of this case: LOG: begin datum sort: workMem = 819200, randomAccess = f LOG: switching to external sort with 2926 tapes: CPU 0.39s/2.66u sec elapsed 3.06 sec LOG: replacement selection avg tuple size 24.00 crossover: 0.85 LOG: hybrid sort-merge in use from row 34952532 with 100000000.00 total rows LOG: finished quicksorting run 1: CPU 0.39s/8.84u sec elapsed 9.24 sec LOG: finished writing quicksorted run 1 to tape 0: CPU 0.60s/9.61u sec elapsed 10.22 sec LOG: finished quicksorting run 2: CPU 0.87s/18.61u sec elapsed 19.50 sec LOG: finished writing quicksorted run 2 to tape 1: CPU 1.07s/19.38u sec elapsed 20.46 sec LOG: performsort starting: CPU 1.27s/21.79u sec elapsed 23.07 sec LOG: finished quicksorting run 3: CPU 1.27s/27.07u sec elapsed 28.35 sec LOG: finished writing quicksorted run 3 to tape 2: CPU 1.47s/27.69u sec elapsed 29.18 sec LOG: performsort done (except 3-way final merge): CPU 1.51s/28.54u sec elapsed 30.07 sec LOG: external sort ended, 146625 disk blocks used: CPU 1.76s/35.32u sec elapsed 37.10 sec Note that the on-tape runs are small relative to CPU costs, so this query is a bit sympathetic (consider the time spent writing batches that trace_sort indicates here). CREATE INDEX would not compare so well with an internal sort, for example, especially if it was a composite index or something. I've sized work_mem here in a deliberate way, to make sure there are 3 runs of similar size by the time the merge step is reached, which makes a small difference in the patch's favor. All told, this seems like a very significant overall improvement. Now, consider master's performance with the same work_mem setting (a fair test with comparable resource usage for master and patch): -- Master set work_mem = '800MB'; select count(distinct(s)) from big_high_cardinality; ***** Runtime after stabilization: ~120.9 seconds ***** The patch is ~3.25x faster than master here, which also seems like a significant improvement. That's pretty close to the improvement previously seen for good "quicksort with spillover" cases, but suitable for every external sort case that doesn't use "quicksort with spillover". In other words, no variety of external sort is not significantly improved by the patch. I think it's safe to suppose that there are also big benefits when multiple concurrent sort operations run on the same system. For example, when pg_restore has multiple jobs. Worst case --------------- Even with a traditionally sympathetic case for replacement selection sort, the patch beats replacement selection with multiple on-tape runs. When experimenting here, I did not forget to account for our qsort()'s behavior in the event of *perfectly* presorted input ("Bubble sort best case" behavior [1]). Other than that, I have a hard time thinking of an unsympathetic case for the patch, and could not find any actual regressions with a fair amount of effort. Abbreviated keys are not used when merging, but that doesn't seem to be something that notably counts against the new approach (which will have shorter runs on average). After all, the reason why abbreviated keys aren't saved on disk for merging is that they're probably not very useful when merging. They would resolve far fewer comparisons if they were used during merging, and having somewhat smaller runs does not result in significantly more non-abbreviated comparisons, even when sorting random noise strings. Avoiding replacement selection *altogether* ================================= Assuming you agree with my conclusions on replacement selection sort mostly not being worth it, we need to avoid replacement selection except when it'll probably allow a "quicksort with spillover". In my mind, that's now the *only* reason to use replacement selection. Callers pass a hint to tuplesort indicating how many tuples it is estimated will ultimately be passed before a sort is performed. (Typically, this comes from a scan plan node's row estimate, or more directly from the relcache for things like CREATE INDEX.) Cost model -- details ---------------------------- Second or subsequent runs *never* use replacement selection -- it is only *considered* for the first run, right before the possible point of initial heapification within inittapes(). The cost model is contained within the new function useselection(). See the second patch in the series for full details. That's where this is added. I have a fairly high bar for even using replacement selection for the first run -- several factors can result in a simple hybrid sort-merge strategy being used instead of a "quicksort with spillover", because in general most of the benefit seems to be around CPU cache misses rather than savings in I/O. Consider my benchmark query above once more -- with replacement selection used for the first run in the benchmark case above (e.g., with just the first patch in the series applied, or setting the "optimize_avoid_selection" debug GUC to "off"), I found that it took over twice as long to execute, even though the second-or-subsequent (now smaller) runs were quicksorted just the same, and were all merged just the same. The numbers should make it obvious why I gave in to the temptation of adding an ad-hoc, tuplesort-private cost model. At this point, I'd rather scrap "quicksort with spillover" (and the use of replacement selection under all possible circumstances) than scrap the idea of a cost model. That would make more sense, even though it would give up on the idea of saving most I/O where the work_mem threshold is only crossed by a small amount. Future work ========= I anticipate a number of other things within the first patch in the series, some of which are already worked out to some degree. Asynchronous I/O ------------------------- This patch leaves open the possibility of using something like libaio/librt for sorting. That would probably use half of memtuples as scratch space, while the other half is quicksorted. Memory prefetching --------------------------- To test what role memory prefetching is likely to have here, I attach a custom version of my tuplesort/tuplestore prefetch patch, with prefetching added to the "quicksort with spillover" and batch dumping runs WRITETUP()-calling code. This seems to help performance measurably. However, I guess it shouldn't really be considered as part of this patch. It can follow the initial commit of the big, base patch (or will becomes part of the base patch if and when prefetching is committed first). cost_sort() changes -------------------------- I had every intention of making cost_sort() a continuous cost function as part of this work. This could be justified by "quicksort with spillover" allowing tuplesort to "blend" from internal to external sorting as input size is gradually increased. This seemed like something that would have significant non-obvious benefits in several other areas. However, I've put off dealing with making any change to cost_sort() because of concerns about the complexity of overlaying such changes on top of the tuplesort-private cost model. I think that this will need to be discussed in a lot more detail. As a further matter, materialization of sort nodes will probably also require tweaks to the costing for "quicksort with spillover". Recall that "quicksort with spillover" can only work for !randomAccess tuplesort callers. Run size ------------ This patch continues to have tuplesort determine run size based on the availability of work_mem only. It does not entirely fix the problem of having work_mem sizing impact performance in counter-intuitive ways. In other words, smaller work_mem sizes can still be faster. It does make that general situation much better, though, because quicksort is a cache oblivious algorithm. Smaller work_mem sizes are sometimes a bit faster, but never dramatically faster. In general, the whole idea of making run size as big as possible is bogus, unless that enables or is likely to enable a "quicksort with spillover". The caller-supplied row count hint I've added may in the future be extended to determine optimal run size ahead of time, when it's perfectly clear (leaving aside misestimation) that a fully internal sort (or "quicksort with spillover") will not occur. This will result in faster external sorts where additional work_mem cannot be put to good use. As a side benefit, external sorts will not be effectively wasting a large amount of memory. The cost model we eventually come up with to determine optimal run size ought to balance certain things. Assuming a one-pass merge step, then we should balance the time lost waiting on the first run and time quicksorting the last run with the gradual increase in the cost during the merge step. Maybe the non-use of abbreviated keys during the merge step should also be considered. Alternatively, the run size may be determined by a GUC that is typically sized at drive controller cache size (e.g. 1GB) when any kind of I/O avoidance for the sort appears impossible. [1] Commit a3f0b3d6 -- Peter Geoghegan
Attachment
On Thu, Aug 20, 2015 at 3:24 AM, Peter Geoghegan <pg@heroku.com> wrote: > I believe, in general, that we should consider a multi-pass sort to be > a kind of inherently suspect thing these days, in the same way that > checkpoints occurring 5 seconds apart are: not actually abnormal, but > something that we should regard suspiciously. Can you really not > afford enough work_mem to only do one pass? Does it really make sense > to add far more I/O and CPU costs to avoid that other tiny memory > capacity cost? I think this is the crux of the argument. And I think you're basically, but not entirely, right. The key metric there is not how cheap memory has gotten but rather what the ratio is between the system's memory and disk storage. The use case I think you're leaving out is the classic "data warehouse" with huge disk arrays attached to a single host running massive queries for hours. In that case reducing run size will reduce I/O requirements directly and halving the amount of I/O sort takes will halve the time it takes regardless of cpu efficiency. And I have a suspicion typical data distributions get much better than a 2x speedup. But I think you're basically right that this is the wrong use case to worry about for most users. Even those users that do have large batch queries are probably not processing so much that they should be doing multiple passes. The ones that do are are probably more interested in parallel query, federated databases, column stores, and so on rather than worrying about just how many hours it takes to sort their multiple terabytes on a single processor. I am quite suspicious of quicksort though. It has O(n^2) worst case and I think it's only a matter of time before people start worrying about DOS attacks from users able to influence the data ordering. It's also not very suitable for GPU processing. Quicksort gets most of its advantage from cache efficiency, it isn't a super efficient algorithm otherwise, are there not other cache efficient algorithms to consider? Alternately, has anyone tested whether Timsort would work well? -- greg
Greg Stark <stark@mit.edu> writes: > Alternately, has anyone tested whether Timsort would work well? I think that was proposed a few years ago and did not look so good in simple testing. regards, tom lane
On 20 August 2015 at 03:24, Peter Geoghegan <pg@heroku.com> wrote:
Can you summarize what this patch does? I understand clearly what it doesn't do...
--
The patch is ~3.25x faster than master
I've tried to read this post twice and both times my work_mem overflowed. ;-)
Simon Riggs http://www.2ndQuadrant.com/
PostgreSQL Development, 24x7 Support, Remote DBA, Training & Services
PostgreSQL Development, 24x7 Support, Remote DBA, Training & Services
On Thu, Aug 20, 2015 at 6:54 AM, Tom Lane <tgl@sss.pgh.pa.us> wrote: > Greg Stark <stark@mit.edu> writes: >> Alternately, has anyone tested whether Timsort would work well? > > I think that was proposed a few years ago and did not look so good > in simple testing. I tested it in 2012. I got as far as writing a patch. Timsort is very good where comparisons are expensive -- that's why it's especially compelling when your comparator is written in Python. However, when testing it with text, even though there were significantly fewer comparisons, it was still slower than quicksort. Quicksort is cache oblivious, and that's an enormous advantage. This was before abbreviated keys; these days, the difference must be larger. -- Peter Geoghegan
On Thu, Aug 20, 2015 at 8:15 AM, Simon Riggs <simon@2ndquadrant.com> wrote: > On 20 August 2015 at 03:24, Peter Geoghegan <pg@heroku.com> wrote: >> >> >> The patch is ~3.25x faster than master > > > I've tried to read this post twice and both times my work_mem overflowed. > ;-) > > Can you summarize what this patch does? I understand clearly what it doesn't > do... The most important thing that it does is always quicksort runs, that are formed by simply filling work_mem with tuples in no particular order, rather than trying to make runs that are twice as large as work_mem on average. That's what the ~3.25x improvement concerned. That's actually a significantly simpler algorithm than replacement selection, and appears to be much faster. You might even say that it's a dumb algorithm, because it is less sophisticated than replacement selection. However, replacement selection tends to use CPU caches very poorly, while its traditional advantages have become dramatically less important due to large main memory sizes in particular. Also, it hurts that we don't currently dump tuples in batches, for several reasons. Better to do memory intense operations in batch, rather than having a huge inner loop, in order to minimize or prevent instruction cache misses. And we can better take advantage of asynchronous I/O. The complicated aspect of considering the patch is whether or not it's okay to not use replacement selection anymore -- is that an appropriate trade-off? The reason that the code has not actually been simplified by this patch is that I still want to use replacement selection for one specific case: when it is anticipated that a "quicksort with spillover" can occur, which is only possible with incremental spilling. That may avoid most I/O, by spilling just a few tuples using a heap/priority queue, and quicksorting everything else. That's compelling when you can manage it, but no reason to always use replacement selection for the first run in the common case where there well be several runs in total. Is that any clearer? To borrow a phrase from the processor architecture community, from a high level this is a "Brainiac versus Speed Demon" [1] trade-off. (I wish that there was a widely accepted name for this trade-off.) [1] http://www.lighterra.com/papers/modernmicroprocessors/#thebrainiacdebate -- Peter Geoghegan
On Thu, Aug 20, 2015 at 10:41 AM, Peter Geoghegan <pg@heroku.com> wrote:
On Thu, Aug 20, 2015 at 8:15 AM, Simon Riggs <simon@2ndquadrant.com> wrote:
> On 20 August 2015 at 03:24, Peter Geoghegan <pg@heroku.com> wrote:
>>
>>
>> The patch is ~3.25x faster than master
>
>
> I've tried to read this post twice and both times my work_mem overflowed.
> ;-)
>
> Can you summarize what this patch does? I understand clearly what it doesn't
> do...
The most important thing that it does is always quicksort runs, that
are formed by simply filling work_mem with tuples in no particular
order, rather than trying to make runs that are twice as large as
work_mem on average. That's what the ~3.25x improvement concerned.
That's actually a significantly simpler algorithm than replacement
selection, and appears to be much faster. You might even say that it's
a dumb algorithm, because it is less sophisticated than replacement
selection. However, replacement selection tends to use CPU caches very
poorly, while its traditional advantages have become dramatically less
important due to large main memory sizes in particular. Also, it hurts
that we don't currently dump tuples in batches, for several reasons.
Better to do memory intense operations in batch, rather than having a
huge inner loop, in order to minimize or prevent instruction cache
misses. And we can better take advantage of asynchronous I/O.
The complicated aspect of considering the patch is whether or not it's
okay to not use replacement selection anymore -- is that an
appropriate trade-off?
The reason that the code has not actually been simplified by this
patch is that I still want to use replacement selection for one
specific case: when it is anticipated that a "quicksort with
spillover" can occur, which is only possible with incremental
spilling. That may avoid most I/O, by spilling just a few tuples using
a heap/priority queue, and quicksorting everything else. That's
compelling when you can manage it, but no reason to always use
replacement selection for the first run in the common case where there
well be several runs in total.
Is that any clearer? To borrow a phrase from the processor
architecture community, from a high level this is a "Brainiac versus
Speed Demon" [1] trade-off. (I wish that there was a widely accepted
name for this trade-off.)
[1] http://www.lighterra.com/papers/modernmicroprocessors/#thebrainiacdebate--
Peter Geoghegan
--
Sent via pgsql-hackers mailing list (pgsql-hackers@postgresql.org)
To make changes to your subscription:
http://www.postgresql.org/mailpref/pgsql-hackers
Hi, Peter,
Just a quick anecdotal evidence. I did similar experiment about three years ago. The conclusion was that if you have SSD, just do quick sort and forget the longer runs, but if you are using hard drives, longer runs is the winner (and safer, to avoid cliffs). I did not experiment with RAID0/5 on many spindles though.
Not limited to sort, more generally, SSD is different enough from HDD, therefore it may worth the effort for backend to "guess" what storage device it has, then choose the right thing to do.
Cheers.
On Thu, Aug 20, 2015 at 12:42 PM, Feng Tian <ftian@vitessedata.com> wrote: > Just a quick anecdotal evidence. I did similar experiment about three years > ago. The conclusion was that if you have SSD, just do quick sort and > forget the longer runs, but if you are using hard drives, longer runs is the > winner (and safer, to avoid cliffs). I did not experiment with RAID0/5 on > many spindles though. > > Not limited to sort, more generally, SSD is different enough from HDD, > therefore it may worth the effort for backend to "guess" what storage device > it has, then choose the right thing to do. The devil is in the details. I cannot really comment on such a general statement. I would be willing to believe that that's true under unrealistic/unrepresentative conditions. Specifically, when multiple passes are required with a sort-merge strategy where that isn't the case with replacement selection. This could happen with a tiny work_mem setting (tiny in an absolute sense more than a relative sense). With an HDD, where sequential I/O is so much faster, this could be enough to make replacement selection win, just as it would have in the 1970s with magnetic tapes. As I've said, the solution is to simply avoid multiple passes, which should be possible in virtually all cases because of the quadratic growth in a classic hybrid sort-merge strategy's capacity to avoid multiple passes (growth relative to work_mem's growth). Once you ensure that, then you probably have a mostly I/O bound workload, which can be made faster by adding sequential I/O capacity (or, on the Postgres internals side, adding asynchronous I/O, or with memory prefetching). You cannot really buy a faster CPU to make a degenerate heapsort faster. -- Peter Geoghegan
On Thu, Aug 20, 2015 at 1:16 PM, Peter Geoghegan <pg@heroku.com> wrote:
On Thu, Aug 20, 2015 at 12:42 PM, Feng Tian <ftian@vitessedata.com> wrote:
> Just a quick anecdotal evidence. I did similar experiment about three years
> ago. The conclusion was that if you have SSD, just do quick sort and
> forget the longer runs, but if you are using hard drives, longer runs is the
> winner (and safer, to avoid cliffs). I did not experiment with RAID0/5 on
> many spindles though.
>
> Not limited to sort, more generally, SSD is different enough from HDD,
> therefore it may worth the effort for backend to "guess" what storage device
> it has, then choose the right thing to do.
The devil is in the details. I cannot really comment on such a general
statement.
I would be willing to believe that that's true under
unrealistic/unrepresentative conditions. Specifically, when multiple
passes are required with a sort-merge strategy where that isn't the
case with replacement selection. This could happen with a tiny
work_mem setting (tiny in an absolute sense more than a relative
sense). With an HDD, where sequential I/O is so much faster, this
could be enough to make replacement selection win, just as it would
have in the 1970s with magnetic tapes.
As I've said, the solution is to simply avoid multiple passes, which
should be possible in virtually all cases because of the quadratic
growth in a classic hybrid sort-merge strategy's capacity to avoid
multiple passes (growth relative to work_mem's growth). Once you
ensure that, then you probably have a mostly I/O bound workload, which
can be made faster by adding sequential I/O capacity (or, on the
Postgres internals side, adding asynchronous I/O, or with memory
prefetching). You cannot really buy a faster CPU to make a degenerate
heapsort faster.
--
Peter Geoghegan
Agree everything in principal,except one thing -- no, random IO on HDD in 2010s (relative to CPU/Memory/SSD), is not any faster than tape in 1970s. :-)
On Thu, Aug 20, 2015 at 1:28 PM, Feng Tian <ftian@vitessedata.com> wrote: > Agree everything in principal,except one thing -- no, random IO on HDD in > 2010s (relative to CPU/Memory/SSD), is not any faster than tape in 1970s. > :-) Sure. The advantage of replacement selection could be a deciding factor in unrepresentative cases, as I mentioned, but even then it's not going to be a dramatic difference as it would have been in the past. By the way, please don't top-post. -- Peter Geoghegan
On Thu, Aug 20, 2015 at 6:05 AM, Greg Stark <stark@mit.edu> wrote: > On Thu, Aug 20, 2015 at 3:24 AM, Peter Geoghegan <pg@heroku.com> wrote: >> I believe, in general, that we should consider a multi-pass sort to be >> a kind of inherently suspect thing these days, in the same way that >> checkpoints occurring 5 seconds apart are: not actually abnormal, but >> something that we should regard suspiciously. Can you really not >> afford enough work_mem to only do one pass? Does it really make sense >> to add far more I/O and CPU costs to avoid that other tiny memory >> capacity cost? > > I think this is the crux of the argument. And I think you're > basically, but not entirely, right. I agree that that's the crux of my argument. I disagree about my not being entirely right. :-) > The key metric there is not how cheap memory has gotten but rather > what the ratio is between the system's memory and disk storage. The > use case I think you're leaving out is the classic "data warehouse" > with huge disk arrays attached to a single host running massive > queries for hours. In that case reducing run size will reduce I/O > requirements directly and halving the amount of I/O sort takes will > halve the time it takes regardless of cpu efficiency. And I have a > suspicion typical data distributions get much better than a 2x > speedup. It could reduce seek time, which might be the dominant cost (but not I/O as such). I do accept that my argument did not really apply to this case, but you seem to be making an additional non-conflicting argument that certain data warehousing cases would be helped in another way by my patch. My argument was only about multi-gigabyte cases that I tested that were significantly improved, primarily due to CPU caching effects. If this helps with extremely large sorts that do require multiple passes by reducing seek time -- I think that they'd have to be multi-terabyte sorts, which I am ill-equipped to test -- then so much the better, I suppose. In any case, as I've said the way we allow run size to be dictated only by available memory (plus whatever replacement selection can do to make on-tape runs longer) is bogus. In the future there should be a cost model for an optimal run size, too. > But I think you're basically right that this is the wrong use case to > worry about for most users. Even those users that do have large batch > queries are probably not processing so much that they should be doing > multiple passes. The ones that do are are probably more interested in > parallel query, federated databases, column stores, and so on rather > than worrying about just how many hours it takes to sort their > multiple terabytes on a single processor. I suppose so. If you can afford multiple terabytes of storage, you can probably still afford gigabytes of memory to do a single pass. My laptop is almost 3 years old, weighs about 1.5 Kg, and has 16 GiB of memory. It's usually always that simple, and not really because we assume that Postgres doesn't have to deal with multi-terabyte sorts. Maybe I lack perspective, having never really dealt with a real data warehouse. I didn't mean to imply that in no circumstances could anyone profit from a multi-pass sort. If you're using Hadoop or something, I imagine that it still makes sense. In general, I think you'll agree that we should strongly leverage the fact that a multi-pass sort just isn't going to be needed when things are set up correctly under standard operating conditions nowadays. > I am quite suspicious of quicksort though. It has O(n^2) worst case > and I think it's only a matter of time before people start worrying > about DOS attacks from users able to influence the data ordering. It's > also not very suitable for GPU processing. Quicksort gets most of its > advantage from cache efficiency, it isn't a super efficient algorithm > otherwise, are there not other cache efficient algorithms to consider? I think that high quality quicksort implementations [1] will continue to be the way to go for sorting integers internally at the very least. Practically speaking, problems with the worst case performance have been completely ironed out since the early 1990s. I think it's possible to DOS Postgres by artificially introducing a worst-case, but it's very unlikely to be the easiest way of doing that in practice. I admit that it's probably the coolest way, though. I think that the benefits of offloading sorting to the GPU are not in evidence today. This may be especially true of a "street legal" implementation that takes into account all of the edge cases, as opposed to a hand customized thing for sorting uniformly distributed random integers. GPU sorts tend to use radix sort, and I just can't see that catching on. [1] https://www.cs.princeton.edu/~rs/talks/QuicksortIsOptimal.pdf -- Peter Geoghegan
On Thu, Aug 20, 2015 at 11:16 PM, Peter Geoghegan <pg@heroku.com> wrote: > It could reduce seek time, which might be the dominant cost (but not > I/O as such). No I didn't quite follow the argument to completion. Increasing the run size is a win if it reduces the number of passes. In the single-pass case it has to read all the data once, write it all out to tapes, then read it all back in again.So 3x the data. If it's still not sorted it needs to write it all back out yet again and read it all back in again. So 5x the data. If the tapes are larger it can avoid that 66% increase in total I/O. In large data sets it can need 3, 4, or maybe more passes through the data and saving one pass would be a smaller incremental difference. I haven't thought through the exponential growth carefully enough to tell if doubling the run size should decrease the number of passes linearly or by a constant number. But you're right that seems to be less and less a realistic scenario. Times when users are really processing data sets that large nowadays they'll just throw it into Hadoop or Biigquery or whatever to get the parallelism of many cpus. Or maybe Citus and the like. The main case where I expect people actually run into this is in building indexes, especially for larger data types (which come to think of it might be exactly where the comparison is expensive enough that quicksort's cache efficiency isn't helpful). But to do fair tests I would suggest you configure work_mem smaller (since running tests on multi-terabyte data sets is a pain) and sort some slower data types that don't fit in memory. Maybe arrays of text or json? -- greg
On Thu, Aug 20, 2015 at 5:02 PM, Greg Stark <stark@mit.edu> wrote: > I haven't thought through the exponential > growth carefully enough to tell if doubling the run size should > decrease the number of passes linearly or by a constant number. It seems that with 5 times the data that previously required ~30MB to avoid a multi-pass sort (where ~2300MB is required for an internal sort -- the benchmark query), it took ~60MB to avoid a multi-pass sort. I guess I just didn't exactly determine either threshold due to that taking too long, and that as predicted, every time the input size quadruples, the required amount of work_mem to avoid multiple passes only doubles. That will need to be verified more vigorously, but it looks that way. > But you're right that seems to be less and less a realistic scenario. > Times when users are really processing data sets that large nowadays > they'll just throw it into Hadoop or Biigquery or whatever to get the > parallelism of many cpus. Or maybe Citus and the like. I'm not sure that even that's generally true, simply because sorting a huge amount of data is very expensive -- it's not really a "big data" thing, so to speak. Look at recent results on this site: http://sortbenchmark.org Last year's winning "Gray" entrant, TritonSort, uses a huge parallel cluster of 186 machines, but only sorts 100TB. That's just over 500GB per node. Each node is a 32 core Intel Xeon EC2 instance with 244GB memory, and lots of SSDs. It seems like the point of the 100TB minimum rule in the "Gray" contest category is that that's practically impossible to fit entirely in memory (to avoid merging). Eventually, linearithmic growth becomes extremely painful, not matter how much processing power you have. It takes a while, though. -- Peter Geoghegan
On 20 August 2015 at 18:41, Peter Geoghegan <pg@heroku.com> wrote:
--
On Thu, Aug 20, 2015 at 8:15 AM, Simon Riggs <simon@2ndquadrant.com> wrote:
> On 20 August 2015 at 03:24, Peter Geoghegan <pg@heroku.com> wrote:
>>
>>
>> The patch is ~3.25x faster than master
>
>
> I've tried to read this post twice and both times my work_mem overflowed.
> ;-)
>
> Can you summarize what this patch does? I understand clearly what it doesn't
> do...
The most important thing that it does is always quicksort runs, that
are formed by simply filling work_mem with tuples in no particular
order, rather than trying to make runs that are twice as large as
work_mem on average. That's what the ~3.25x improvement concerned.
That's actually a significantly simpler algorithm than replacement
selection, and appears to be much faster.
Then I think this is fine, not least because it seems like a first step towards parallel sort.
This will give more runs, so merging those needs some thought. It will also give a more predictable number of runs, so we'll be able to predict any merging issues ahead of time. We can more easily find out the min/max tuple in each run, so we only merge overlapping runs.
You might even say that it's
a dumb algorithm, because it is less sophisticated than replacement
selection. However, replacement selection tends to use CPU caches very
poorly, while its traditional advantages have become dramatically less
important due to large main memory sizes in particular. Also, it hurts
that we don't currently dump tuples in batches, for several reasons.
Better to do memory intense operations in batch, rather than having a
huge inner loop, in order to minimize or prevent instruction cache
misses. And we can better take advantage of asynchronous I/O.
The complicated aspect of considering the patch is whether or not it's
okay to not use replacement selection anymore -- is that an
appropriate trade-off?
Using a heapsort is known to be poor for large heaps. We previously discussed the idea of quicksorting the first chunk of memory, then reallocating the heap as a smaller chunk for the rest of the sort. That would solve the cache miss problem.
I'd like to see some discussion of how we might integrate aggregation and sorting. A heap might work quite well for that, whereas quicksort doesn't sound like it would work as well.
The reason that the code has not actually been simplified by this
patch is that I still want to use replacement selection for one
specific case: when it is anticipated that a "quicksort with
spillover" can occur, which is only possible with incremental
spilling. That may avoid most I/O, by spilling just a few tuples using
a heap/priority queue, and quicksorting everything else. That's
compelling when you can manage it, but no reason to always use
replacement selection for the first run in the common case where there
well be several runs in total.
I think its premature to retire that algorithm - I think we should keep it for a while yet. I suspect it may serve well in cases where we have low memory, though I accept that is no longer the case for larger servers that we would now call typical.
This could cause particular issues in optimization, since heap sort is wonderfully predictable. We'd need a cost_sort() that was slightly pessimistic to cover the risk that a quicksort might not be as fast as we hope.
Is that any clearer?
Yes, thank you.
I'd like to see a more general and concise plan for how sorting evolves. We are close to having the infrastructure to perform intermediate aggregation, which would allow that to happen during sorting when required (aggregation, sort distinct). We also agreed some time back that parallel sorting would be the first incarnation of parallel operations, so we need to consider that also.
Simon Riggs http://www.2ndQuadrant.com/
PostgreSQL Development, 24x7 Support, Remote DBA, Training & Services
PostgreSQL Development, 24x7 Support, Remote DBA, Training & Services
On Thu, Aug 20, 2015 at 11:56 PM, Simon Riggs <simon@2ndquadrant.com> wrote: > This will give more runs, so merging those needs some thought. It will also > give a more predictable number of runs, so we'll be able to predict any > merging issues ahead of time. We can more easily find out the min/max tuple > in each run, so we only merge overlapping runs. I think that merging runs can be optimized to reduce the number of cache misses. Poul-Henning Kamp, the FreeBSD guy, has described problems with binary heaps and cache misses [1], and I think we could use his solution for merging. But we should definitely still quicksort runs. > Using a heapsort is known to be poor for large heaps. We previously > discussed the idea of quicksorting the first chunk of memory, then > reallocating the heap as a smaller chunk for the rest of the sort. That > would solve the cache miss problem. > > I'd like to see some discussion of how we might integrate aggregation and > sorting. A heap might work quite well for that, whereas quicksort doesn't > sound like it would work as well. If you're talking about deduplicating within tuplesort, then there are techniques. I don't know that that needs to be an up-front priority of this work. > I think its premature to retire that algorithm - I think we should keep it > for a while yet. I suspect it may serve well in cases where we have low > memory, though I accept that is no longer the case for larger servers that > we would now call typical. I have given one case where I think the first run should still use replacement selection: where that enables a "quicksort with spillover". For that reason, I would consider that I have not actually proposed to retire the algorithm. In principle, I agree with also using it under any other circumstances where it is likely to be appreciably faster, but it's just not in evidence that there is any other such case. I did look at all the traditionally sympathetic cases, as I went into, and it still seemed to not be worth it at all. But by all means, if you think I missed something, please show me a test case. > This could cause particular issues in optimization, since heap sort is > wonderfully predictable. We'd need a cost_sort() that was slightly > pessimistic to cover the risk that a quicksort might not be as fast as we > hope. Wonderfully predictable? Really? It's totally sensitive to CPU cache characteristics. I wouldn't say that at all. If you're alluding to the quicksort worst case, that seems like the wrong thing to worry about. The risk around that is often overstated, or based on experience from third-rate implementations that don't follow various widely accepted recommendations from the research community. > I'd like to see a more general and concise plan for how sorting evolves. We > are close to having the infrastructure to perform intermediate aggregation, > which would allow that to happen during sorting when required (aggregation, > sort distinct). We also agreed some time back that parallel sorting would be > the first incarnation of parallel operations, so we need to consider that > also. I agree with everything you say here, I think. I think it's appropriate that this work anticipate adding a number of other optimizations in the future, at least including: * Parallel sort using worker processes. * Memory prefetching. * Offset-value coding of runs, a compression technique that was used in System R, IIRC. This can speed up merging a lot, and will save I/O bandwidth on dumping out runs. * Asynchronous I/O. There should be an integrated approach to applying every possible optimization, or at least leaving the possibility open. A lot of these techniques are complementary. For example, there are significant benefits where the "onlyKey" optimization is now used with external sorts, which you get for free by using quicksort for runs. In short, I am absolutely on-board with the idea that these things need to be anticipated at the very least. For another speculative example, offset coding makes the merge step cheaper, but the work of doing the offset coding can be offloaded to worker processes, whereas the merge step proper cannot really be effectively parallelized -- those two techniques together are greater than the sum of their parts. One big problem that I see with replacement selection is that it makes most of these things impossible. In general, I think that parallel sort should be an external sort technique first and foremost. If you can only parallelize an internal sort, then running out of road when there isn't enough memory to do the sort in memory becomes a serious issue. Besides, you need to partition the input anyway, and external sorting naturally needs to do that while not precluding runs not actually being dumped to disk. [1] http://queue.acm.org/detail.cfm?id=1814327 -- Peter Geoghegan
On Wed, Aug 19, 2015 at 7:24 PM, Peter Geoghegan <pg@heroku.com> wrote: > Let's start by comparing an external sort that uses 1/3 the memory of > an internal sort against the master branch. That's completely unfair > on the patch, of course, but it is a useful indicator of how well > external sorts do overall. Although an external sort surely cannot be > as fast as an internal sort, it might be able to approach an internal > sort's speed when there is plenty of I/O bandwidth. That's a good > thing to aim for, I think. > The patch only takes ~10% more time to execute this query, which seems > very good considering that ~1/3 the work_mem has been put to use. > Note that the on-tape runs are small relative to CPU costs, so this > query is a bit sympathetic (consider the time spent writing batches > that trace_sort indicates here). CREATE INDEX would not compare so > well with an internal sort, for example, especially if it was a > composite index or something. This is something that I've made great progress on (see "concrete example" below for numbers). The differences in the amount of I/O required between these two cases (due to per-case variability in the width of tuples written to tape for datum sorts and index sorts) did not significantly factor in to the differences in performance, it turns out. The big issue was that while a pass-by-value datum sort accidentally has good cache characteristics during the merge step, that is not generally true. I figured out a way of making it generally true, though. I attach a revised patch series with a new commit that adds an optimization to the merge step, relieving what was a big remaining bottleneck in the CREATE INDEX case (and *every* external sort case that isn't a pass-by-value datum sort, which is most things). There are a few tweaks to earlier commits including, but nothing very interesting. All of my benchmarks suggests that this most recent revision puts external sorting within a fairly small margin of a fully internal sort on the master branch in many common cases. This difference is seen when the implementation only makes use of a fraction of the memory required for an internal sort, provided the system is reasonably well balanced. For a single backend, there is an overhead of about 5% - 20% against master's internal sort performance. This speedup appears to be fairly robust across a variety of different cases. I particularly care about CREATE INDEX, since that is where most pain is felt in the real world, and I'm happy that I found a way to make CREATE INDEX external sort reasonably comparable in run time to internal sorts that consume much more memory. I think it's time to stop talking about this as performance work, and start talking about it as scalability work. With that in mind, I'm mostly going to compare the performance of the new, optimized external sort implementation with the existing internal sort implementation from now on. New patch -- Sequential memory access =============================== The trick I hit upon for relieving the merge bottleneck was fairly simple. Prefetching works for internal sorts, but isn't practical for external sorts while merging. OTOH, I can arrange to have runs allocate their "tuple proper" contents into a memory pool, partitioned by final on-the-fly tape number. Today, runs/tapes are slurped from disk sequentially in a staggered fashion, based on the availability of in-memory tuples from each tape while merging. The new patch is very effective in reducing cache misses by simply making sure that each tape's "tuple proper" (e.g. each IndexTuple) is accessed in memory in the natural, predictable order (the sorted order that runs on tape always have). Unlike with internal sorts (where explicit memory prefetching of each "tuple proper" may be advisable), the final order in which the caller must consume a tape's "tuple proper" is predictable well in advance. A little rearrangement is required to make what were previously retail palloc() calls during prereading (a palloc() for each "tuple proper", within each READTUP() routine) consume space from the memory pool instead. The pool (a big, once-off memory allocation) is reused in a circular fashion per tape partition. This saves a lot of palloc() overhead. Under this scheme, each tape's next few IndexTuples are all in one cacheline. This patch has the merge step make better use of available memory bandwidth, rather than attempting to conceal memory latency. Explicit prefetch instructions (that we may independently end up using to do something similar with internal sorts when fetching tuples following sorting proper) are all about hiding latency. Concrete example -- performance --------------------------------------------- I attach a text file describing a practical, reproducible example CREATE INDEX. It shows how CREATE INDEX now compares fairly well with an equivalent operation that has enough maintenance_work_mem to complete its sort internally. I'll just summarize it here: A CREATE INDEX on a single int4 attribute on an unlogged table takes only ~18% longer. This is a 100 million row table that is 4977 MB on disk. On master, CREATE INDEX takes 66.6 seconds in total with an *internal* sort. With the patch series applied, an *external* sort involving a final on-the-fly merge of 6 runs takes 78.5 seconds. Obviously, since there are 6 runs to merge, work_mem is only approximately 1/6 of what is required for a fully internal sort. High watermark memory usage ------------------------------------------ One concern about the patch may be that it increases the high watermark memory usage by any on-the-fly final merge step. It takes full advantage of the availMem allowance at a point where every "tuple proper" is freed, and availMem has only had SortTuple/memtuples array "slot" memory subtracted (plus overhead). Memory is allocated in bulk once, and partitioned among active tapes, with no particular effort towards limiting memory usage beyond enforcing that we always !LACKMEM(). A lot of the overhead of many retail palloc() calls is removed by simply using one big memory allocation. In practice, LACKMEM() will rarely become true, because the availability of slots now tends to be the limiting factor. This is partially explained by the number of slots being established when palloc() overhead was in play, prior to the final merge step. However, I have concerns about the memory usage of this new approach. With the int4 CREATE INDEX case above, which has a uniform distribution, I noticed that about 40% of each tape's memory space remains unused when slots are exhausted. Ideally, we'd only have allocated enough memory to run out at about the same time that slots are exhausted, since the two would be balanced. This might be possible for fixed-sized tuples. I have not allocated each final on-the-fly merge step's active tape's pool individually, because while this waste of memory is large enough to be annoying, it's not large enough to be significantly helped by managing a bunch of per-tape buffers and enlarging them as needed geometrically (e.g. starting small, and doubling each time the buffer size is hit until the per-tape limit is finally reached). The main reason that the high watermark is increased is not because of this, though. It's mostly just that "tuple proper" memory is not freed until the sort is done, whereas before there were many small pfree() calls to match the many palloc() calls -- calls that occurred early and often. Note that the availability of "slots" (i.e. the size of the memtuples array, minus one element for each tape's heap item) is currently determined by whatever size it happened to be at when memtuples stopped growing, which isn't particularly well principled (hopefully this is no worse now). Optimal memory usage ------------------------------- In the absence of any clear thing to care about most beyond making sorting faster while still enforcing !LACKMEM(), for now I've kept it simple. I am saving a lot of memory by clawing back palloc() overhead, but may be wasting more than that in another way now, to say nothing of the new high watermark itself. If we're entirely I/O bound, maybe we should not waste memory by simply not allocating as much anyway (i.e. the extra memory may only theoretically help even when it is written to). But what does it really mean to be I/O bound? The OS cache probably consumes plenty of memory, too. Finally, let us not forget that it's clearly still the case that even following this work, run size needs to be optimized using a cost model, rather than simply being determined by how much memory can be made available (work_mem). If we get a faster sort using far less work_mem, then the DBA is probably accidentally wasting huge amounts of memory due to failing to do that. As an implementor, it's really hard to balance all of these concerns, or to say that one in particular is most urgent. Parallel sorting =========== Simon rightly emphasized the need for joined-up thinking in relation to applying important tuplesort optimizations. We must at least consider parallelism as part of this work. I'm glad that the first consumer of parallel infrastructure is set to be parallel sequential scans, not internal parallel sorts. That's because it seems that overall, a significant cost is actually reading tuples into memtuples to sort -- heap scanning and related costs in the buffer manager (even assuming everything is in shared_buffers), COPYTUP() palloc() calls, and so on. Taken together, they can be a bigger overall cost than sorting proper, even assuming abbreviated keys are not used. The third bucket that I tend to categorize costs into, "time spent actually writing out finished runs", is small on a well balanced system. Surprisingly small, I would say. I will sketch a simple implementation of parallel sorting based on the patch series that may be workable, and requires relatively little implementation effort compare to other ideas that were raised at various times: * Establish an optimal run size ahead of time using a cost model. We need this for serial external sorts anyway, to relieve the DBA of having to worry about sizing maintenance_work_mem according to obscure considerations around cache efficiency within tuplesort. Parallelism probably doesn't add much complexity to the cost model, which is not especially complicated to begin with. Note that I have not added this cost model yet (just the ad-hoc, tuplesort-private cost model for using replacement selection to get a "quicksort with spillover"). It may be best if this cost model lives in the optimizer. * Have parallel workers do a parallel heap scan of the relation until they fill this optimal run size. Use local memory to sort within workers. Write runs out in the usual way. Then, the worker picks up the next run scheduled. If there are no more runs to build, there is no more work for the parallel workers. * Shut down workers. Do an on-the-fly merge in the parent process. This is the same as with a serial merge, but with a little coordination with worker processes to make sure every run is available, etc. In general, coordination is kept to an absolute minimum. I tend to think that this really simple approach would get much of the gain of something more complicated -- no need to write shared memory management code, minimal need to handle coordination between workers, and no real changes to the algorithms used for each sub-problem. This makes merging more of a bottleneck again, but that is a bottleneck on I/O and especially memory bandwidth. Parallelism cannot help much with that anyway (except by compressing runs with offset coding, perhaps, but that isn't specific to parallelism and won't always help). Writing out runs in bulk is very fast here -- certainly much faster than I thought it would be when I started thinking about external sorting. And if that turns out to be a problem for cases that have sufficient memory to do everything internally, that can later be worked on non-invasively. As I've said in the past, I think parallel sorting only makes sense when memory latency and bandwidth are not huge bottlenecks, which we should bend over backwards to avoid. In a sense, you can't really make use of parallel workers for sorting until you fix that problem first. I am not suggesting that we do this because it's easier than other approaches. I think it's actually most effective to not make parallel sorting too divergent from serial sorting, because making things cumulative makes speed-ups from localized optimizations cumulative, while at the same time, AFAICT there isn't anything to recommend extensive specialization for parallel sort. If what I've sketched is also a significantly easier approach, then that's a bonus. -- Peter Geoghegan
Attachment
- quicksort_external_test.txt
- 0005-Use-tuple-proper-memory-pool-in-tuplesort.patch
- 0004-Prefetch-from-memtuples-array-in-tuplesort.patch
- 0003-Log-requirement-for-multiple-external-sort-passes.patch
- 0002-Further-diminish-role-of-replacement-selection.patch
- 0001-Quicksort-when-performing-external-sorts.patch
>I will sketch a simple implementation of parallel sorting based on the >patch series that may be workable, and requires relatively little >implementation effort compare to other ideas that were raised at >various times: Hello, I've only a very superficial understanding on your work, please apologize if this is off topic or if this was already discussed... Have you considered performances for cases where multiple CREATE INDEX are running in parallel? One of our typical use case are large daily tables (50-300 Mio rows) with up to 6 index creations that start simultaneously. Our servers have 40-60 GB RAM , ca. 12 CPUs and we set maintenance mem to 1-2 GB for this. If the create index themselves start using parallelism, I guess that we might need to review our workflow... best regards, Marc Mamin
On Sun, Sep 6, 2015 at 1:51 AM, Marc Mamin <M.Mamin@intershop.de> wrote: > Have you considered performances for cases where multiple CREATE INDEX are running in parallel? > One of our typical use case are large daily tables (50-300 Mio rows) with up to 6 index creations > that start simultaneously. > Our servers have 40-60 GB RAM , ca. 12 CPUs and we set maintenance mem to 1-2 GB for this. > If the create index themselves start using parallelism, I guess that we might need to review our workflow... Not particularly. I imagine that that case would be helped a lot here (probably more than a simpler case involving only one CREATE INDEX), because each core would be require fewer main memory accesses overall. Maybe you can test it and let us know how it goes. -- Peter Geoghegan
On Wed, Aug 19, 2015 at 7:24 PM, Peter Geoghegan <pg@heroku.com> wrote: > I'll start a new thread for this, since my external sorting patch has > now evolved well past the original "quicksort with spillover" > idea...although not quite how I anticipated it would. It seems like > I've reached a good point to get some feedback. Corey Huinker has once again assisted me with this work, by doing some benchmarking on an AWS instance of his: 32 cores (c3.8xlarge, I suppose) MemTotal: 251902912 kB I believe it had one EBS volume. This testing included 2 data sets: * A data set that he happens to have that is representative of his production use-case. Corey had some complaints about the sort performance of PostgreSQL, particularly prior to 9.5, and I like to link any particular performance optimization to an improvement in an actual production workload, if at all possible. * A tool that I wrote, that works on top of sortbenchmark.org's "gensort" [1] data generation tool. It seems reasonable to me to drive this work in part with a benchmark devised by Jim Gray. He did after all receive a Turing award for this contribution to transaction processing. I'm certainly a fan of his work. A key practical advantage of that is that is has reasonable guarantees about determinism, making these results relatively easy to recreate independently. The modified "gensort" is available from https://github.com/petergeoghegan/gensort The python script postgres_load.py, which performs bulk-loading for Postgres using COPY FREEZE. It ought to be fairly self-documenting: $:~/gensort$ ./postgres_load.py --help usage: postgres_load.py [-h] [-w WORKERS] [-m MILLION] [-s] [-l] [-c] optional arguments: -h, --help show this help message and exit -w WORKERS, --workers WORKERS Number of gensort workers (default: 4) -m MILLION, --million MILLION Generate n million tuples(default: 100) -s, --skew Skew distribution of output keys (default: False) -l, --logged Use loggedPostgreSQL table (default: False) -c, --collate Use default collation rather than C collation (default: False) For this initial report to the list, I'm going to focus on a case involving 16 billion non-skewed tuples generated using the gensort tool. I wanted to see how a sort of a ~1TB table (1017GB as reported by psql, actually) could be improved, as compared to relatively small volumes of data (in the multiple gigabyte range) that were so improved by sorts on my laptop, which has enough memory to avoid blocking on physical I/O much of the time. How the new approach deals with hundreds of runs that are actually reasonably sized is also of interest. This server does have a lot of memory, and many CPU cores. It was kind of underpowered on I/O, though. The initial load of 16 billion tuples (with a sortkey that is "C" locale text) took about 10 hours. My tool supports parallel generation of COPY format files, but serial performance of that stage isn't especially fast. Further, in order to support COPY FREEZE, and in order to ensure perfect determinism, the COPY operations occur serially in a single transaction that creates the table that we performed a CREATE INDEX on. Patch, with 3GB maintenance_work_mem: ... LOG: performsort done (except 411-way final merge): CPU 1017.95s/17615.74u sec elapsed 23910.99 sec STATEMENT: create index on sort_test (sortkey ); LOG: external sort ended, 54740802 disk blocks used: CPU 2001.81s/31395.96u sec elapsed 41648.05 sec STATEMENT: create index on sort_test (sortkey ); So just over 11 hours (11:34:08), then. The initial sorting for 411 runs took 06:38:30.99, as you can see. Master branch: ... LOG: finished writing run 202 to tape 201: CPU 1224.68s/31060.15u sec elapsed 34409.16 sec LOG: finished writing run 203 to tape 202: CPU 1230.48s/31213.55u sec elapsed 34580.41 sec LOG: finished writing run 204 to tape 203: CPU 1236.74s/31366.63u sec elapsed 34750.28 sec LOG: performsort starting: CPU 1241.70s/31501.61u sec elapsed 34898.63 sec LOG: finished writing run 205 to tape 204: CPU 1242.19s/31516.52u sec elapsed 34914.17 sec LOG: finished writing final run 206 to tape 205: CPU 1243.23s/31564.23u sec elapsed 34963.03 sec LOG: performsort done (except 206-way final merge): CPU 1243.86s/31570.58u sec elapsed 34974.08 sec LOG: external sort ended, 54740731 disk blocks used: CPU 2026.98s/48448.13u sec elapsed 55299.24 sec CREATE INDEX Time: 55299315.220 ms So 15:21:39 for master -- it's much improved, but this was still disappointing given the huge improvements on relatively small cases. Finished index was fairly large, which can be seen here by working back from "total relation size": postgres=# select pg_size_pretty(pg_total_relation_size('sort_test'));pg_size_pretty ----------------1487 GB (1 row) I think that this is probably due to the relatively slow I/O on this server, and because the merge step is more of a bottleneck. As we increase maintenance_work_mem, we're likely to then suffer from the lack of explicit asynchronous I/O here. It helps, still, but not dramatically. With with maintenance_work_mem = 30GB, patch is somewhat faster (no reason to think that this would help master at all, so that was untested): ... LOG: starting quicksort of run 40: CPU 1815.99s/19339.80u sec elapsed 24910.38 sec LOG: finished quicksorting run 40: CPU 1820.09s/19565.94u sec elapsed 25140.69 sec LOG: finished writing run 40 to tape 39: CPU 1833.76s/19642.11u sec elapsed 25234.44 sec LOG: performsort starting: CPU 1849.46s/19803.28u sec elapsed 25499.98 sec LOG: starting quicksort of run 41: CPU 1849.46s/19803.28u sec elapsed 25499.98 sec LOG: finished quicksorting run 41: CPU 1852.37s/20000.73u sec elapsed 25700.43 sec LOG: finished writing run 41 to tape 40: CPU 1864.89s/20069.09u sec elapsed 25782.93 sec LOG: performsort done (except 41-way final merge): CPU 1965.43s/20086.28u sec elapsed 25980.80 sec LOG: external sort ended, 54740909 disk blocks used: CPU 3270.57s/31595.37u sec elapsed 40376.43 sec CREATE INDEX Time: 40383174.977 ms So that takes 11:13:03 in total -- we only managed to shave about 20 minutes off the total time taken, despite a 10x increase in maintenance_work_mem. Still, at least it gets moderately better, not worse, which is certainly what I'd expect from the master branch. 60GB was half way between 3GB and 30GB in terms of performance, so it doesn't continue to help, but, again, at least things don't get much worse. Thoughts on these results: * I'd really like to know the role of I/O here. Better, low-overhead instrumentation is required to see when and how we are I/O bound. I've been doing much of that on a more-or-less ad hoc basis so far, using iotop. I'm looking into a way to usefully graph the I/O activity over many hours, to correlate with the trace_sort output that I'll also show. I'm open to suggestions on the easiest way of doing that. Having used the "perf" tool for instrumenting I/O at all in the past. * Parallelism would probably help us here *a lot*. * As I said, I think we suffer from the lack of asynchronous I/O much more at this scale. Will need to confirm that theory. * It seems kind of ill-advised to make run size (which is always in linear proportion to maintenance_work_mem with this new approach to sorting) larger, because it probably will hurt writing runs more than it will help in making merging cheaper (perhaps mostly due to the lack of asynchronous I/O to hide the latency of writes -- Linux might not do so well at this scale). * Maybe adding actual I/O bandwidth is the way to go to get a better picture. I wouldn't be surprised if we were very bottlenecked on I/O here. Might be worth using many parallel EBS volumes here, for example. [1] http://sortbenchmark.org/FAQ-2015.html -- Peter Geoghegan
<div dir="ltr"><div class="gmail_extra"><div class="gmail_quote">On Fri, Nov 6, 2015 at 8:08 PM, Peter Geoghegan <span dir="ltr"><<ahref="mailto:pg@heroku.com" target="_blank">pg@heroku.com</a>></span> wrote:<br /><blockquote class="gmail_quote"style="margin:0 0 0 .8ex;border-left:1px #ccc solid;padding-left:1ex"><span class="">On Wed, Aug 19, 2015at 7:24 PM, Peter Geoghegan <<a href="mailto:pg@heroku.com">pg@heroku.com</a>> wrote:<br /></span><span class="">>I'll start a new thread for this, since my external sorting patch has<br /> > now evolved well past the original"quicksort with spillover"<br /> > idea...although not quite how I anticipated it would. It seems like<br /> >I've reached a good point to get some feedback.<br /><br /></span>Corey Huinker has once again assisted me with thiswork, by doing some<br /> benchmarking on an AWS instance of his:<br /><br /> 32 cores (c3.8xlarge, I suppose)<br />MemTotal: 251902912 kB<br /><br /> I believe it had one EBS volume.<br /><br /> This testing included 2 data sets:<br/><br /> * A data set that he happens to have that is representative of his<br /> production use-case. Corey hadsome complaints about the sort<br /> performance of PostgreSQL, particularly prior to 9.5, and I like to<br /> link anyparticular performance optimization to an improvement in an<br /> actual production workload, if at all possible.<br /><br/> * A tool that I wrote, that works on top of <a href="http://sortbenchmark.org" rel="noreferrer" target="_blank">sortbenchmark.org</a>'s<br/> "gensort" [1] data generation tool. It seems reasonable to me to drive<br />this work in part with a benchmark devised by Jim Gray. He did after<br /> all receive a Turing award for this contributionto transaction<br /> processing. I'm certainly a fan of his work. A key practical advantage<br /> of that isthat is has reasonable guarantees about determinism, making<br /> these results relatively easy to recreate independently.<br/><br /> The modified "gensort" is available from<br /><a href="https://github.com/petergeoghegan/gensort"rel="noreferrer" target="_blank">https://github.com/petergeoghegan/gensort</a><br/><br /> The python script postgres_load.py, which performsbulk-loading for<br /> Postgres using COPY FREEZE. It ought to be fairly self-documenting:<br /><br /> $:~/gensort$./postgres_load.py --help<br /> usage: postgres_load.py [-h] [-w WORKERS] [-m MILLION] [-s] [-l] [-c]<br /><br/> optional arguments:<br /> -h, --help show this help message and exit<br /> -w WORKERS, --workers WORKERS<br/> Number of gensort workers (default: 4)<br /> -m MILLION, --million MILLION<br /> Generate n million tuples (default: 100)<br /> -s, --skew Skew distribution of outputkeys (default: False)<br /> -l, --logged Use logged PostgreSQL table (default: False)<br /> -c, --collate Use default collation rather than C collation<br /> (default: False)<br /><br />For this initial report to the list, I'm going to focus on a case<br /> involving 16 billion non-skewed tuples generatedusing the gensort<br /> tool. I wanted to see how a sort of a ~1TB table (1017GB as reported<br /> by psql, actually)could be improved, as compared to relatively small<br /> volumes of data (in the multiple gigabyte range) that wereso improved<br /> by sorts on my laptop, which has enough memory to avoid blocking on<br /> physical I/O much of thetime. How the new approach deals with<br /> hundreds of runs that are actually reasonably sized is also of<br /> interest.This server does have a lot of memory, and many CPU cores.<br /> It was kind of underpowered on I/O, though.<br/><br /> The initial load of 16 billion tuples (with a sortkey that is "C"<br /> locale text) took about 10 hours.My tool supports parallel generation<br /> of COPY format files, but serial performance of that stage isn't<br /> especiallyfast. Further, in order to support COPY FREEZE, and in<br /> order to ensure perfect determinism, the COPY operationsoccur<br /> serially in a single transaction that creates the table that we<br /> performed a CREATE INDEX on.<br/><br /> Patch, with 3GB maintenance_work_mem:<br /><br /> ...<br /> LOG: performsort done (except 411-way final merge):CPU<br /> 1017.95s/17615.74u sec elapsed 23910.99 sec<br /> STATEMENT: create index on sort_test (sortkey );<br />LOG: external sort ended, 54740802 disk blocks used: CPU<br /> 2001.81s/31395.96u sec elapsed 41648.05 sec<br /> STATEMENT: create index on sort_test (sortkey );<br /><br /> So just over 11 hours (11:34:08), then. The initial sortingfor 411<br /> runs took 06:38:30.99, as you can see.<br /><br /> Master branch:<br /><br /> ...<br /> LOG: finishedwriting run 202 to tape 201: CPU 1224.68s/31060.15u sec<br /> elapsed 34409.16 sec<br /> LOG: finished writing run203 to tape 202: CPU 1230.48s/31213.55u sec<br /> elapsed 34580.41 sec<br /> LOG: finished writing run 204 to tape 203:CPU 1236.74s/31366.63u sec<br /> elapsed 34750.28 sec<br /> LOG: performsort starting: CPU 1241.70s/31501.61u sec elapsed34898.63 sec<br /> LOG: finished writing run 205 to tape 204: CPU 1242.19s/31516.52u sec<br /> elapsed 34914.17 sec<br/> LOG: finished writing final run 206 to tape 205: CPU<br /> 1243.23s/31564.23u sec elapsed 34963.03 sec<br /> LOG: performsort done (except 206-way final merge): CPU<br /> 1243.86s/31570.58u sec elapsed 34974.08 sec<br /> LOG: externalsort ended, 54740731 disk blocks used: CPU<br /> 2026.98s/48448.13u sec elapsed 55299.24 sec<br /> CREATE INDEX<br/> Time: 55299315.220 ms<br /><br /> So 15:21:39 for master -- it's much improved, but this was still<br /> disappointinggiven the huge improvements on relatively small cases.<br /><br /> Finished index was fairly large, which canbe seen here by working<br /> back from "total relation size":<br /><br /> postgres=# select pg_size_pretty(pg_total_relation_size('sort_test'));<br/> pg_size_pretty<br /> ----------------<br /> 1487 GB<br /> (1row)<br /><br /> I think that this is probably due to the relatively slow I/O on this<br /> server, and because the mergestep is more of a bottleneck. As we<br /> increase maintenance_work_mem, we're likely to then suffer from the<br />lack of explicit asynchronous I/O here. It helps, still, but not<br /> dramatically. With with maintenance_work_mem = 30GB,patch is somewhat<br /> faster (no reason to think that this would help master at all, so that<br /> was untested):<br/><br /> ...<br /> LOG: starting quicksort of run 40: CPU 1815.99s/19339.80u sec elapsed<br /> 24910.38 sec<br/> LOG: finished quicksorting run 40: CPU 1820.09s/19565.94u sec elapsed<br /> 25140.69 sec<br /> LOG: finished writingrun 40 to tape 39: CPU 1833.76s/19642.11u sec<br /> elapsed 25234.44 sec<br /> LOG: performsort starting: CPU 1849.46s/19803.28usec elapsed 25499.98 sec<br /> LOG: starting quicksort of run 41: CPU 1849.46s/19803.28u sec elapsed<br/> 25499.98 sec<br /> LOG: finished quicksorting run 41: CPU 1852.37s/20000.73u sec elapsed<br /> 25700.43 sec<br/> LOG: finished writing run 41 to tape 40: CPU 1864.89s/20069.09u sec<br /> elapsed 25782.93 sec<br /> LOG: performsortdone (except 41-way final merge): CPU<br /> 1965.43s/20086.28u sec elapsed 25980.80 sec<br /> LOG: external sortended, 54740909 disk blocks used: CPU<br /> 3270.57s/31595.37u sec elapsed 40376.43 sec<br /> CREATE INDEX<br /> Time:40383174.977 ms<br /><br /> So that takes 11:13:03 in total -- we only managed to shave about 20<br /> minutes off thetotal time taken, despite a 10x increase in<br /> maintenance_work_mem. Still, at least it gets moderately better, not<br/> worse, which is certainly what I'd expect from the master branch. 60GB<br /> was half way between 3GB and 30GB interms of performance, so it<br /> doesn't continue to help, but, again, at least things don't get much<br /> worse.<br/><br /> Thoughts on these results:<br /><br /> * I'd really like to know the role of I/O here. Better, low-overhead<br/> instrumentation is required to see when and how we are I/O bound. I've<br /> been doing much of that ona more-or-less ad hoc basis so far, using<br /> iotop. I'm looking into a way to usefully graph the I/O activity over<br/> many hours, to correlate with the trace_sort output that I'll also<br /> show. I'm open to suggestions on the easiestway of doing that. Having<br /> used the "perf" tool for instrumenting I/O at all in the past.<br /><br /> * Parallelismwould probably help us here *a lot*.<br /><br /> * As I said, I think we suffer from the lack of asynchronousI/O much<br /> more at this scale. Will need to confirm that theory.<br /><br /> * It seems kind of ill-advisedto make run size (which is always in<br /> linear proportion to maintenance_work_mem with this new approach to<br/> sorting) larger, because it probably will hurt writing runs more than<br /> it will help in making merging cheaper(perhaps mostly due to the lack<br /> of asynchronous I/O to hide the latency of writes -- Linux might not<br /> doso well at this scale).<br /><br /> * Maybe adding actual I/O bandwidth is the way to go to get a better<br /> picture.I wouldn't be surprised if we were very bottlenecked on I/O<br /> here. Might be worth using many parallel EBS volumeshere, for<br /> example.<br /><br /> [1] <a href="http://sortbenchmark.org/FAQ-2015.html" rel="noreferrer" target="_blank">http://sortbenchmark.org/FAQ-2015.html</a><br/><span class="HOEnZb"><font color="#888888">--<br /> PeterGeoghegan<br /></font></span></blockquote></div><br /></div><div class="gmail_extra">The machine in question still exists,so if you have questions about it, commands you'd like me to run to give you insight as to the I/O capabilities ofthe machine, let me know. I can't guarantee we'll keep the machine much longer.</div><div class="gmail_extra"><br /></div><divclass="gmail_extra"><br /></div><div class="gmail_extra"><br /></div><div class="gmail_extra"><br /></div></div>
On Wed, Aug 19, 2015 at 7:24 PM, Peter Geoghegan <pg@heroku.com> wrote: Hi Peter, Your most recent versions of this patch series (not the ones on the email I am replying to) give a compiler warning: tuplesort.c: In function 'mergeruns': tuplesort.c:2741: warning: unused variable 'memNowUsed' > Multi-pass sorts > --------------------- > > I believe, in general, that we should consider a multi-pass sort to be > a kind of inherently suspect thing these days, in the same way that > checkpoints occurring 5 seconds apart are: not actually abnormal, but > something that we should regard suspiciously. Can you really not > afford enough work_mem to only do one pass? I don't think it is really about the cost of RAM. What people can't afford is spending all of their time personally supervising all the sorts on the system. It is pretty easy for a transient excursion in workload to make a server swap itself to death and fall over. Not just the PostgreSQL server, but the entire OS. Since we can't let that happen, we have to be defensive about work_mem. Yes, we have far more RAM than we used to. We also have far more things demanding access to it at the same time. I agree we don't want to optimize for low memory, but I don't think we should throw it under the bus, either. Right now we are effectively saying the CPU-cache problems with the heap start exceeding the larger run size benefits at 64kb (the smallest allowed setting for work_mem). While any number we pick is going to be a guess that won't apply to all hardware, surely we can come up with a guess better than 64kb. Like, 8 MB, say. If available memory for the sort is 8MB or smaller and the predicted size anticipates a multipass merge, then we can use the heap method rather than the quicksort method. Would a rule like that complicate things much? It doesn't matter to me personally at the moment, because the smallest work_mem I run on a production system is 24MB. But if for some reason I had to increase max_connections, or had to worry about plans with many more possible concurrent work_mem allocations (like some partitioning), then I might not need to rethink that setting downward. > > In theory, the answer could be "yes", but it seems highly unlikely. > Not only is very little memory required to avoid a multi-pass merge > step, but as described above the amount required grows very slowly > relative to linear growth in input. I propose to add a > checkpoint_warning style warning (with a checkpoint_warning style GUC > to control it). I'm skeptical about a warning for this. I think it is rather unlike checkpointing, because checkpointing is done in a background process, which greatly limits its visibility, while sorting is a foreground thing. I know if my sorts are slow, without having to go look in the log file. If we do have the warning, shouldn't it use a log-level that gets sent to the front end where the person running the sort can see it and locally change work_mem? And if we have a GUC, I think it should be a dial, not a binary. If I have a sort that takes a 2-way merge and then a final 29-way merge, I don't think that that is worth reporting. So maybe, if the maximum number of runs on a tape exceeds 2 (rather than exceeds 1, which is the current behavior with the patch) would be the setting I would want to use, if I were to use it at all. ... > This patch continues to have tuplesort determine run size based on the > availability of work_mem only. It does not entirely fix the problem of > having work_mem sizing impact performance in counter-intuitive ways. > In other words, smaller work_mem sizes can still be faster. It does > make that general situation much better, though, because quicksort is > a cache oblivious algorithm. Smaller work_mem sizes are sometimes a > bit faster, but never dramatically faster. Yes, that is what I found as well. I think the main reason it is even that small bit slower at large memory is because writing and sorting are not finely interleaved, like they are with heap selection. Once you sit down to qsort 3GB of data, you are not going to write any more tuples until that qsort is entirely done. I didn't do any testing beyond 3GB of maintenance_work_mem, but I imagine this could get more important if people used dozens or hundreds of GB. One idea would be to stop and write out a just-sorted partition whenever that partition is contiguous to the already-written portion. If the qsort is tweaked to recurse preferentially into the left partition first, this would result in tuples being written out at a pretty study pace. If the qsort was unbalanced and the left partition was always the larger of the two, then that approach would have to be abandoned at some point. But I think there are already defenses against that, and at worst you would give up and revert to the sort-them-all then write-them-all behavior. Overall this is very nice. Doing some real world index builds of short text (~20 bytes ascii) identifiers, I could easily get speed ups of 40% with your patch if I followed the philosophy of "give it as much maintenance_work_mem as I can afford". If I fine-tuned the maintenance_work_mem so that it was optimal for each sort method, then the speed up quite a bit less, only 22%. But 22% is still very worthwhile, and who wants to spend their time fine-tuning the memory use for every index build? Cheers, Jeff
Hi Jeff, On Wed, Nov 18, 2015 at 10:31 AM, Jeff Janes <jeff.janes@gmail.com> wrote: > tuplesort.c: In function 'mergeruns': > tuplesort.c:2741: warning: unused variable 'memNowUsed' That was caused by a last-minute change to the mulitpass warning message. I forgot to build at -O2, and missed this. >> I believe, in general, that we should consider a multi-pass sort to be >> a kind of inherently suspect thing these days, in the same way that >> checkpoints occurring 5 seconds apart are: not actually abnormal, but >> something that we should regard suspiciously. Can you really not >> afford enough work_mem to only do one pass? > > I don't think it is really about the cost of RAM. What people can't > afford is spending all of their time personally supervising all the > sorts on the system. It is pretty easy for a transient excursion in > workload to make a server swap itself to death and fall over. Not just > the PostgreSQL server, but the entire OS. Since we can't let that > happen, we have to be defensive about work_mem. Yes, we have far more > RAM than we used to. We also have far more things demanding access to > it at the same time. I agree with you, but I'm not sure that I've been completely clear on what I mean. Even as the demand on memory has grown, the competitive advantage of replacement selection in avoiding a multi-pass merge has diminished far faster. You should simply not allow it to happen as a DBA -- that's the advice that other systems' documentation makes. Avoiding a multi-pass merge was always the appeal of replacement selection, even in the 1970s, but it will rarely if ever make that critical difference these days. As I said, as the volume of data to be sorted in memory increases linearly, the point at which a multi-pass merge phase happens increases quadratically with my patch. The advantage of replacement selection is therefore almost irrelevant. That is why, in general, interest in replacement selection is far far lower today than it was in the past. The poor CPU cache characteristics of the heap (priority queue) are only half the story about why replacement selection is more or less obsolete these days. > I agree we don't want to optimize for low memory, but I don't think we > should throw it under the bus, either. Right now we are effectively > saying the CPU-cache problems with the heap start exceeding the larger > run size benefits at 64kb (the smallest allowed setting for work_mem). > While any number we pick is going to be a guess that won't apply to > all hardware, surely we can come up with a guess better than 64kb. > Like, 8 MB, say. If available memory for the sort is 8MB or smaller > and the predicted size anticipates a multipass merge, then we can use > the heap method rather than the quicksort method. Would a rule like > that complicate things much? I'm already using replacement selection for the first run when it is predicted by my new ad-hoc cost model that we can get away with a "quicksort with spillover", avoiding almost all I/O. We only incrementally spill as many tuples as needed right now, but it would be pretty easy to not quicksort the remaining tuples, but continue to incrementally spill everything. So no, it wouldn't be too hard to hang on to the old behavior sometimes, if it looked worthwhile. In principle, I have no problem with doing that. Through testing, I cannot see any actual upside, though. Perhaps I just missed something. Even 8MB is enough to avoid the multipass merge in the event of a surprisingly high volume of data (my work laptop is elsewhere, so I don't have my notes on this in front of me, but I figured out the crossover point for a couple of cases). >> In theory, the answer could be "yes", but it seems highly unlikely. >> Not only is very little memory required to avoid a multi-pass merge >> step, but as described above the amount required grows very slowly >> relative to linear growth in input. I propose to add a >> checkpoint_warning style warning (with a checkpoint_warning style GUC >> to control it). > > I'm skeptical about a warning for this. Other systems expose this explicitly, and, as I said, say in an unqualified way that a multi-pass merge should be avoided. Maybe the warning isn't the right way of communicating that message to the DBA in detail, but I am confident that it ought to be communicated to the DBA fairly clearly. > One idea would be to stop and write out a just-sorted partition > whenever that partition is contiguous to the already-written portion. > If the qsort is tweaked to recurse preferentially into the left > partition first, this would result in tuples being written out at a > pretty study pace. If the qsort was unbalanced and the left partition > was always the larger of the two, then that approach would have to be > abandoned at some point. But I think there are already defenses > against that, and at worst you would give up and revert to the > sort-them-all then write-them-all behavior. Seems kind of invasive. > Overall this is very nice. Doing some real world index builds of > short text (~20 bytes ascii) identifiers, I could easily get speed ups > of 40% with your patch if I followed the philosophy of "give it as > much maintenance_work_mem as I can afford". If I fine-tuned the > maintenance_work_mem so that it was optimal for each sort method, then > the speed up quite a bit less, only 22%. But 22% is still very > worthwhile, and who wants to spend their time fine-tuning the memory > use for every index build? Thanks, but I expected better than that. Was it a collated text column? The C collation will put the patch in a much better light (more strcoll() calls are needed with this new approach -- it's still well worth it, but it is a downside that makes collated text not especially sympathetic). Just sorting on an integer attribute is also a good sympathetic case, FWIW. How much time did the sort take in each case? How many runs? How much time was spent merging? trace_sort output is very interesting here. -- Peter Geoghegan
On Wed, Nov 18, 2015 at 11:29 PM, Peter Geoghegan <pg@heroku.com> wrote: > Other systems expose this explicitly, and, as I said, say in an > unqualified way that a multi-pass merge should be avoided. Maybe the > warning isn't the right way of communicating that message to the DBA > in detail, but I am confident that it ought to be communicated to the > DBA fairly clearly. I'm pretty convinced warnings from DML are a categorically bad idea. In any OLTP load they're effectively fatal errors since they'll fill up log files or client output or cause other havoc. Or they'll cause no problem because nothing is reading them. Neither behaviour is useful. Perhaps the right thing to do is report a statistic to pg_stats so DBAs can see how often sorts are in memory, how often they're on disk, and how often the on disk sort requires n passes. That would put them in the same category as "sequential scans" for DBAs that expect the application to only run index-based OLTP queries for example. The problem with this is that sorts are not tied to a particular relation and without something to group on the stat will be pretty hard to act on. -- greg
On Wed, Nov 18, 2015 at 6:29 PM, Peter Geoghegan <pg@heroku.com> wrote: > In principle, I have no problem with doing that. Through testing, I > cannot see any actual upside, though. Perhaps I just missed something. > Even 8MB is enough to avoid the multipass merge in the event of a > surprisingly high volume of data (my work laptop is elsewhere, so I > don't have my notes on this in front of me, but I figured out the > crossover point for a couple of cases). I'd be interested in seeing this analysis in some detail. -- Robert Haas EnterpriseDB: http://www.enterprisedb.com The Enterprise PostgreSQL Company
On Wed, Nov 18, 2015 at 5:22 PM, Greg Stark <stark@mit.edu> wrote: > On Wed, Nov 18, 2015 at 11:29 PM, Peter Geoghegan <pg@heroku.com> wrote: >> Other systems expose this explicitly, and, as I said, say in an >> unqualified way that a multi-pass merge should be avoided. Maybe the >> warning isn't the right way of communicating that message to the DBA >> in detail, but I am confident that it ought to be communicated to the >> DBA fairly clearly. > > I'm pretty convinced warnings from DML are a categorically bad idea. > In any OLTP load they're effectively fatal errors since they'll fill > up log files or client output or cause other havoc. Or they'll cause > no problem because nothing is reading them. Neither behaviour is > useful. To be clear, this is a LOG level message, not a WARNING. I think that if the DBA ever sees the multipass_warning message, he or she does not have an OLTP workload. If you experience what might be considered log spam due to multipass_warning, then the log spam is the least of your problems. Besides, log_temp_files is a very similar setting (albeit one that is not enabled by default), so I tend to doubt that your view that that style of log message is categorically bad is widely shared. Having said that, I'm not especially attached to the idea of communicating the concern to the DBA using the mechanism of a checkpoint_warning-style LOG message (multipass_warning). Yes, I really do mean it when I say that the DBA is not supposed to see this message, no matter how much or how little memory or data is involved. There is no nuance intended here; it isn't sensible to allow a multi-pass sort, just as it isn't sensible to allow checkpoints every 5 seconds. Both of those things can be thought of as thrashing. > Perhaps the right thing to do is report a statistic to pg_stats so > DBAs can see how often sorts are in memory, how often they're on disk, > and how often the on disk sort requires n passes. That might be better than what I came up with, but I hesitate to track more things using the statistics collector in the absence of a clear consensus to do so. I'd be more worried about the overhead of what you suggest than the overhead of a LOG message, seen only in the case of something that's really not supposed to happen. -- Peter Geoghegan
On 19 November 2015 at 01:22, Greg Stark <stark@mit.edu> wrote:
--
Perhaps the right thing to do is report a statistic to pg_stats so
DBAs can see how often sorts are in memory, how often they're on disk,
and how often the on disk sort requires n passes. That would put them
in the same category as "sequential scans" for DBAs that expect the
application to only run index-based OLTP queries for example. The
problem with this is that sorts are not tied to a particular relation
and without something to group on the stat will be pretty hard to act
on.
+1
We don't have a message appear when hash joins use go weird, and we definitely don't want anything like that for sorts either.
Simon Riggs http://www.2ndQuadrant.com/
PostgreSQL Development, 24x7 Support, Remote DBA, Training & Services
PostgreSQL Development, 24x7 Support, Remote DBA, Training & Services
On Thu, Nov 19, 2015 at 6:56 PM, Peter Geoghegan <pg@heroku.com> wrote: > Yes, I really do mean it when I say that the DBA is not supposed to > see this message, no matter how much or how little memory or data is > involved. There is no nuance intended here; it isn't sensible to allow > a multi-pass sort, just as it isn't sensible to allow checkpoints > every 5 seconds. Both of those things can be thought of as thrashing. Hm. So a bit of back-of-envelope calculation. If we have want to buffer at least 1MB for each run -- I think we currently do more actually -- and say that a 1GB work_mem ought to be enough to run reasonably (that's per sort after all and there might be multiple sorts to say nothing of other users on the system). That means we can merge about 1,000 runs in the final merge. Each run will be about 2GB currently but 1GB if we quicksort the runs. So the largest table we can sort in a single pass is 1-2 TB. If we go above those limits we have the choice of buffering less per run or doing a whole second pass through the data. I suspect we would get more horsepower out of buffering less though I'm not sure where the break-even point is. Certainly if we did random I/O for every I/O that's much more expensive than a factor of 2 over sequential I/O. We could probably do the math based on random_page_cost and sequential_page_cost to calculate the minimum amount of buffering before it's worth doing an extra pass. So I think you're kind of right and kind of wrong. The vast majority of use cases are either sub 1TB or are in work environments designed specifically for data warehouse queries where a user can obtain much more memory for their queries. However I think it's within the intended use cases that Postgres should be able to handle a few terabytes of data on a moderately sized machine in a shared environment too. Our current defaults are particularly bad for this though. If you initdb a new Postgres database today and create a table even a few gigabytes and try to build an index on it it takes forever. The last time I did a test I canceled it after it had run for hours, raised maintenance_work_mem and built the index in a few minutes. The problem is that if we just raise those limits then people will use more resources when they don't need it. If it were safer for to have those limits be much higher then we could make the defaults reflect what people want when they do bigger jobs rather than just what they want for normal queries or indexes. > I think that if the DBA ever sees the multipass_warning message, he or she does not have an OLTP workload. Hm, that's pretty convincing. I guess this isn't the usual sort of warning due to the time it would take to trigger. -- greg
On Wed, Nov 18, 2015 at 6:19 PM, Robert Haas <robertmhaas@gmail.com> wrote: > On Wed, Nov 18, 2015 at 6:29 PM, Peter Geoghegan <pg@heroku.com> wrote: >> In principle, I have no problem with doing that. Through testing, I >> cannot see any actual upside, though. Perhaps I just missed something. >> Even 8MB is enough to avoid the multipass merge in the event of a >> surprisingly high volume of data (my work laptop is elsewhere, so I >> don't have my notes on this in front of me, but I figured out the >> crossover point for a couple of cases). > > I'd be interested in seeing this analysis in some detail. Sure. Jeff mentioned 8MB as a work_mem setting, so let's examine a case where that's the work_mem setting, and see experimentally where the crossover point for a multi-pass sort ends up. If this table is created: postgres=# create unlogged table bar as select (random() * 1e9)::int4 idx, 'payload xyz'::text payload from generate_series(1, 10100000) i; SELECT 10100000 Then, on my system, a work_mem setting of 8MB *just about* avoids seeing the multipass_warning message with this query: postgres=# select count(distinct idx) from bar ; count ------------10,047,433 (1 row) A work_mem setting of 235MB is just enough to make the query's sort fully internal. Let's see how things change with a higher work_mem setting of 16MB. I mentioned quadratic growth: Having doubled work_mem, let's *quadruple* the number of tuples, to see where this leaves a 16MB setting WRT a multi-pass merge: postgres=# drop table bar ; DROP TABLE postgres=# create unlogged table bar as select (random() * 1e9)::int4 idx, 'payload xyz'::text payload from generate_series(1, 10100000 * 4) i; SELECT 40400000 Further experiments show that this is the exact point at which the 16MB work_mem setting similarly narrowly avoids a multi-pass warning. This should be the dominant consideration, because now a fully internal sort requires 4X the work_mem of my original 16MB work_mem example table/query. The quadratic growth in a simple hybrid sort-merge strategy's ability to avoid a multi-pass merge phase (growth relative to linear increases in work_mem) can be demonstrated with simple experiments. -- Peter Geoghegan
On Thu, Nov 19, 2015 at 8:35 PM, Greg Stark <stark@mit.edu> wrote: > Hm. So a bit of back-of-envelope calculation. If we have want to > buffer at least 1MB for each run -- I think we currently do more > actually -- and say that a 1GB work_mem ought to be enough to run > reasonably (that's per sort after all and there might be multiple > sorts to say nothing of other users on the system). That means we can > merge about 1,000 runs in the final merge. Each run will be about 2GB > currently but 1GB if we quicksort the runs. So the largest table we > can sort in a single pass is 1-2 TB. For the sake of pedantry I fact checked myself. We calculate the number of tapes based on wanting to buffer 32 blocks plus overhead so about 256kB. So the actual maximum you can handle with 1GB of sort_mem without multiple merges is on the order 4-8TB. -- greg
On Thu, Nov 19, 2015 at 3:43 PM, Peter Geoghegan <pg@heroku.com> wrote: >> I'd be interested in seeing this analysis in some detail. > > Sure. Jeff mentioned 8MB as a work_mem setting, so let's examine a > case where that's the work_mem setting, and see experimentally where > the crossover point for a multi-pass sort ends up. > > If this table is created: > > postgres=# create unlogged table bar as select (random() * 1e9)::int4 > idx, 'payload xyz'::text payload from generate_series(1, 10100000) i; > SELECT 10100000 > > Then, on my system, a work_mem setting of 8MB *just about* avoids > seeing the multipass_warning message with this query: > > postgres=# select count(distinct idx) from bar ; > > count > ------------ > 10,047,433 > (1 row) > > A work_mem setting of 235MB is just enough to make the query's sort > fully internal. > > Let's see how things change with a higher work_mem setting of 16MB. I > mentioned quadratic growth: Having doubled work_mem, let's *quadruple* > the number of tuples, to see where this leaves a 16MB setting WRT a > multi-pass merge: > > postgres=# drop table bar ; > DROP TABLE > postgres=# create unlogged table bar as select (random() * 1e9)::int4 > idx, 'payload xyz'::text payload from generate_series(1, 10100000 * 4) > i; > SELECT 40400000 > > Further experiments show that this is the exact point at which the > 16MB work_mem setting similarly narrowly avoids a multi-pass warning. > This should be the dominant consideration, because now a fully > internal sort requires 4X the work_mem of my original 16MB work_mem > example table/query. > > The quadratic growth in a simple hybrid sort-merge strategy's ability > to avoid a multi-pass merge phase (growth relative to linear increases > in work_mem) can be demonstrated with simple experiments. OK, so reversing this analysis, with the default work_mem of 4MB, we'd need a multi-pass merge for more than 235MB/4 = 58MB of data. That is very, very far from being a can't-happen scenario, and I would not at all think it would be acceptable to ignore such a case. Even ignoring the possibility that someone with work_mem = 8MB will try to sort 235MB of data strikes me as out of the question. Those seem like entirely reasonable things for users to do. Greg's example of someone with work_mem = 1GB trying to sort 4TB does not seem like a crazy thing to me. Yeah, in all of those cases you might think that users should set work_mem higher, but that doesn't mean that they actually do. Most systems have to set work_mem very conservatively to make sure they don't start swapping under heavily load. I think you need to revisit your assumptions here. -- Robert Haas EnterpriseDB: http://www.enterprisedb.com The Enterprise PostgreSQL Company
On Thu, Nov 19, 2015 at 12:35 PM, Greg Stark <stark@mit.edu> wrote: > So I think you're kind of right and kind of wrong. The vast majority > of use cases are either sub 1TB or are in work environments designed > specifically for data warehouse queries where a user can obtain much > more memory for their queries. However I think it's within the > intended use cases that Postgres should be able to handle a few > terabytes of data on a moderately sized machine in a shared > environment too. Maybe I've made this more complicated than it needs to be. The fact is that my recent 16MB example is still faster than the master branch when a multiple pass merge is performed (e.g. when work_mem is 15MB, or even 12MB). More on that later. > Our current defaults are particularly bad for this though. If you > initdb a new Postgres database today and create a table even a few > gigabytes and try to build an index on it it takes forever. The last > time I did a test I canceled it after it had run for hours, raised > maintenance_work_mem and built the index in a few minutes. The problem > is that if we just raise those limits then people will use more > resources when they don't need it. I think that the bigger problems are: * There is a harsh discontinuity in the cost function -- performance suddenly falls off a cliff when a sort must be performed externally. * Replacement selection is obsolete. It's very slow on machines from the last 20 years. > If it were safer for to have those > limits be much higher then we could make the defaults reflect what > people want when they do bigger jobs rather than just what they want > for normal queries or indexes. Or better yet, make it so that it doesn't really matter that much, even while you're still using the same amount of memory as before. If you're saying that the whole work_mem model isn't a very good one, then I happen to agree. It would be very nice to have some fancy admission control feature, but I'd still appreciate a cost model that dynamically sets work_mem. The model avoids an excessively high setting where there is only about half the memory available for a 10GB sort. You should probably have 5 runs sized 2GB, rather than 2 runs sized 5GB, even if you can afford the memory for the latter. It would still make sense to have very high work_mem settings when you can dynamically set it so high that the sort does complete internally, though. >> I think that if the DBA ever sees the multipass_warning message, he or she does not have an OLTP workload. > > Hm, that's pretty convincing. I guess this isn't the usual sort of > warning due to the time it would take to trigger. I would like more opinions on the multipass_warning message. I can write a patch that creates a new system view, detailing how sort were completed, if there is demand. -- Peter Geoghegan
On Thu, Nov 19, 2015 at 2:35 PM, Robert Haas <robertmhaas@gmail.com> wrote: > OK, so reversing this analysis, with the default work_mem of 4MB, we'd > need a multi-pass merge for more than 235MB/4 = 58MB of data. That is > very, very far from being a can't-happen scenario, and I would not at > all think it would be acceptable to ignore such a case. > I think you need to revisit your assumptions here. Which assumption? Are we talking about multipass_warning, or my patch series in general? Obviously those are two very differently things. As I've said, we could address the visibility aspect of this differently. I'm fine with that. I'll now talk about my patch series in general -- the actual consequences of not avoiding a single pass merge phase when the master branch would have done so. The latter 16MB work_mem example query/table is still faster with a 12MB work_mem than master, even with multiple passes. Quite a bit faster, in fact: about 37 seconds on master, to about 24.7 seconds with the patches (same for higher settings short of 16MB). Now, that's probably slightly unfair on the master branch, because the patches still have the benefit of the memory pooling during the merge phase, which is nothing to do with what we're talking about, and because my laptop still has plenty of ram. I should point out that there is no evidence that any case has been regressed, let alone written off entirely or ignored. I looked. I probably have not been completely exhaustive, and I'd be willing to believe there is something that I've missed, but it's still quite possible that there is no downside to any of this. -- Peter Geoghegan
On Thu, Nov 19, 2015 at 2:53 PM, Peter Geoghegan <pg@heroku.com> wrote: > The latter 16MB work_mem example query/table is still faster with a > 12MB work_mem than master, even with multiple passes. Quite a bit > faster, in fact: about 37 seconds on master, to about 24.7 seconds > with the patches (same for higher settings short of 16MB). I made the same comparison with work_mem sizes of 2MB and 6MB for master/patch, and the patch *still* came out ahead, often by over 10%. This was more than fair, though, because sometimes the final on-the-fly merge for the master branch starting at a point at which the patch series has already completed its sort. (Of course, I don't believe that any user would ever be well served with such a low work_mem setting for these queries -- I'm looking for a bad case, though). I guess this is a theoretical downside of my approach, that is more than made up for elsewhere (even leaving aside the final, unrelated patch in the series, addressing the merge bottleneck directly). So, to summarize such downsides (downsides of a hybrid sort-merge strategy as compared to replacement selection): * As mentioned just now, the fact that there are more runs -- merging can be slower (although tuples can be returned earlier, which could also help with CREATE INDEX). This is more of a problem when random I/O is expensive, and less of a problem when the OS cache buffers things nicely. * One run can be created with replacement selection, where a hyrbid-sort merge strategy needs to create and then merge many runs. When I started work on this patch, I was pretty sure that case would be noticeably regressed. I was wrong. * Abbreviated key comparisons are used less because runs are smaller. This is why sorts of types like numeric are not especially sympathetic to the patch. Still, we manage to come out well ahead overall. You can perhaps show the patch to be almost as slow as the master branch with a very unsympathetic case involving the union of all these 3. I couldn't regress a case with integers with just the first two, though. -- Peter Geoghegan
On Fri, Nov 20, 2015 at 12:54 AM, Peter Geoghegan <pg@heroku.com> wrote: > * One run can be created with replacement selection, where a > hyrbid-sort merge strategy needs to create and then merge many runs. > When I started work on this patch, I was pretty sure that case would > be noticeably regressed. I was wrong. Hm. Have you tested a nearly-sorted input set around 1.5x the size of work_mem? That should produce a single run using the heap to generate runs but generate two runs if, AIUI, you're just filling work_mem, running quicksort, dumping that run entirely and starting fresh. I don't mean to say it's representative but if you're looking for a worst case... -- greg
On Thu, Nov 19, 2015 at 5:32 PM, Greg Stark <stark@mit.edu> wrote: > Hm. Have you tested a nearly-sorted input set around 1.5x the size of > work_mem? That should produce a single run using the heap to generate > runs but generate two runs if, AIUI, you're just filling work_mem, > running quicksort, dumping that run entirely and starting fresh. Yes. Actually, even with a random ordering, on average replacement selection sort will produce runs twice as long as the patch series. With nearly ordered input, there is no limit to how log runs can be -- you could definitely have cases where *no* merge step is required. We just return tuples from one long run. And yet, it isn't worth it in cases that I tested. Please don't take my word for it -- try yourself. -- Peter Geoghegan
On Thu, Nov 19, 2015 at 5:42 PM, Peter Geoghegan <pg@heroku.com> wrote: > I would like more opinions on the multipass_warning message. I can > write a patch that creates a new system view, detailing how sort were > completed, if there is demand. I think a warning message is a terrible idea, and a system view is a needless complication. If the patch is as fast or faster than what we have now in all cases, then we should adopt it (assuming it's also correct and well-commented and all that other good stuff). If it's not, then we need to analyze the cases where it's slower and decide whether they are significant enough to care about. -- Robert Haas EnterpriseDB: http://www.enterprisedb.com The Enterprise PostgreSQL Company
On Thu, Nov 19, 2015 at 5:53 PM, Peter Geoghegan <pg@heroku.com> wrote: > I'll now talk about my patch series in general -- the actual > consequences of not avoiding a single pass merge phase when the master > branch would have done so. That's what I was asking about. It seemed to me that you were saying we could ignore those cases, which doesn't seem to me to be true. > The latter 16MB work_mem example query/table is still faster with a > 12MB work_mem than master, even with multiple passes. Quite a bit > faster, in fact: about 37 seconds on master, to about 24.7 seconds > with the patches (same for higher settings short of 16MB). Is this because we save enough by quicksorting rather than heapsorting to cover the cost of the additional merge phase? If not, then why is it happening like this? > I should point out that there is no evidence that any case has been > regressed, let alone written off entirely or ignored. I looked. I > probably have not been completely exhaustive, and I'd be willing to > believe there is something that I've missed, but it's still quite > possible that there is no downside to any of this. If that's so, it's excellent news. -- Robert Haas EnterpriseDB: http://www.enterprisedb.com The Enterprise PostgreSQL Company
On Fri, Nov 20, 2015 at 12:50 PM, Robert Haas <robertmhaas@gmail.com> wrote: > On Thu, Nov 19, 2015 at 5:42 PM, Peter Geoghegan <pg@heroku.com> wrote: >> I would like more opinions on the multipass_warning message. I can >> write a patch that creates a new system view, detailing how sort were >> completed, if there is demand. > > I think a warning message is a terrible idea, and a system view is a > needless complication. If the patch is as fast or faster than what we > have now in all cases, then we should adopt it (assuming it's also > correct and well-commented and all that other good stuff). If it's > not, then we need to analyze the cases where it's slower and decide > whether they are significant enough to care about. Maybe I was mistaken to link the idea to this patch, but I think it (or something involving a view) is a good idea. I linked it to the patch because the patch makes it slightly more important than before. -- Peter Geoghegan
On Fri, Nov 20, 2015 at 12:52 PM, Robert Haas <robertmhaas@gmail.com> wrote: > That's what I was asking about. It seemed to me that you were saying > we could ignore those cases, which doesn't seem to me to be true. I've been around for long enough to know that there are very few cases that can be ignored. :-) >> The latter 16MB work_mem example query/table is still faster with a >> 12MB work_mem than master, even with multiple passes. Quite a bit >> faster, in fact: about 37 seconds on master, to about 24.7 seconds >> with the patches (same for higher settings short of 16MB). > > Is this because we save enough by quicksorting rather than heapsorting > to cover the cost of the additional merge phase? > > If not, then why is it happening like this? I think it's because of caching effects alone, but I am not 100% sure of that. I concede that it might not be enough to make up for the additional I/O on some systems or platforms. The fact remains, however, that the patch was faster on the unsympathetic case I ran on the machine I had available (which has an SSD), and that I really have not managed to find a case that is regressed after some effort. >> I should point out that there is no evidence that any case has been >> regressed, let alone written off entirely or ignored. I looked. I >> probably have not been completely exhaustive, and I'd be willing to >> believe there is something that I've missed, but it's still quite >> possible that there is no downside to any of this. > > If that's so, it's excellent news. As I mentioned up-thread, maybe I shouldn't have brought all the theoretical justifications for killing replacement selection into the discussion so early. Those observations on replacement selection (which are not my own original insights) happen to be what spurred this work. I spent so much time talking about how irrelevant multi-pass merging was that people imagined that that was severely regressed, when it really was not. That just happened to be the way I came at the problem. The numbers speak for themselves here. I just want to be clear about the disadvantages of what I propose, even if it's well worth it overall in most (all?) cases. -- Peter Geoghegan
On Fri, Nov 20, 2015 at 2:58 PM, Peter Geoghegan <pg@heroku.com> wrote: > The numbers speak for themselves here. I just want to be clear about > the disadvantages of what I propose, even if it's well worth it > overall in most (all?) cases. There is a paper called "Critical Evaluation of Existing External Sorting Methods in the Perspective of Modern Hardware": http://ceur-ws.org/Vol-1343/paper8.pdf This paper was not especially influential, and I don't agree with every detail, or I at least don't think that every recommendation should be adopted to Postgres. Even still, the paper is the best summary I have seen so far. It clearly explains why there is plenty to recommend a simple hybrid sort-merge strategy over replacement selection, despite the fact that replacement selection is faster when using 1970s hardware. -- Peter Geoghegan
On Wed, Nov 18, 2015 at 3:29 PM, Peter Geoghegan <pg@heroku.com> wrote: >> Overall this is very nice. Doing some real world index builds of >> short text (~20 bytes ascii) identifiers, I could easily get speed ups >> of 40% with your patch if I followed the philosophy of "give it as >> much maintenance_work_mem as I can afford". If I fine-tuned the >> maintenance_work_mem so that it was optimal for each sort method, then >> the speed up quite a bit less, only 22%. But 22% is still very >> worthwhile, and who wants to spend their time fine-tuning the memory >> use for every index build? > > Thanks, but I expected better than that. It also might have been that you used a "quicksort with spillover". That still uses a heap to some degree, in order to avoid most I/O, but with a single backend sorting that can often be slower than the (greatly overhauled) "external merge" sort method (both of these algorithms are what you'll see in EXPLAIN ANALYZE, which can be a little confusing because it isn't clear what the distinction is in some cases). You might also very occasionally see an "external sort" (this is also a description from EXPLAIN ANALYZE), which is generally slower (it's a case where we were unable to do a final on-the-fly merge, either because random access is requested by the caller, or because multiple passes were required -- thankfully this doesn't happen most of the time). -- Peter Geoghegan
On 20 November 2015 at 22:58, Peter Geoghegan <pg@heroku.com> wrote:
--
The numbers speak for themselves here. I just want to be clear about
the disadvantages of what I propose, even if it's well worth it
overall in most (all?) cases.
My feeling is that numbers rarely speak for themselves, without LSD. (Which numbers?)
How are we doing here? Keen to see this work get committed, so we can move onto parallel sort. What's the summary?
How about we commit it with a sort_algorithm = 'foo' parameter so we can compare things before release of 9.6?
Simon Riggs http://www.2ndQuadrant.com/
PostgreSQL Development, 24x7 Support, Remote DBA, Training & Services
PostgreSQL Development, 24x7 Support, Remote DBA, Training & Services
On Tue, Nov 24, 2015 at 3:32 PM, Simon Riggs <simon@2ndquadrant.com> wrote: > My feeling is that numbers rarely speak for themselves, without LSD. (Which > numbers?) Guffaw. > How are we doing here? Keen to see this work get committed, so we can move > onto parallel sort. What's the summary? I showed a test case where a CREATE INDEX sort involving 5 runs and a merge only took about 18% longer than an equivalent fully internal sort [1] using over 5 times the memory. That's about 2.5X faster than the 9.5 performance on the same system with the same amount of memory. Overall, the best cases I saw were the original "quicksort with spillover" cases [2]. They were just under 4X faster. I care about that less, though, because that will happen way less often, and won't help with larger sorts that are even more CPU bound. There is a theoretical possibility that this is slower on systems where multiple merge passes are required as a consequence of not having runs as long as possible (due to not using replacement selection heap). That will happen very infrequently [3], and is very probably still worth it. So, the bottom line is: This patch seems very good, is unlikely to have any notable downside (no case has been shown to be regressed), but has yet to receive code review. I am working on a new version with the first two commits consolidated, and better comments, but that will have the same code, unless I find bugs or am dissatisfied. It mostly needs thorough code review, and to a lesser extent some more performance testing. Parallel sort is very important. Robert, Amit and I had a call about this earlier today. We're all in agreement that this should be extended in that direction, and have a rough idea about how it ought to fit together with the parallelism primitives. Parallel sort in 9.6 could certainly happen -- that's what I'm aiming for. I haven't really done preliminary research yet; I'll know more in a little while. > How about we commit it with a sort_algorithm = 'foo' parameter so we can > compare things before release of 9.6? I had a debug GUC (like the existing one to disable top-N heapsorts) that disabled "quicksort with spillover". That's almost the opposite of what you're asking for, though, because that makes us never use a heap. You're asking for me to write a GUC to always use a heap. That's not a good way of testing this patch, because it's inconvenient to consider the need to use a heap beyond the first run (something that now exists solely for the benefit of "quicksort with spillover"; a heap will often never be used even for the first run). Besides, the merge optimization is a big though independent part of this, and doesn't make sense to control with the same GUC. If I haven't gotten this right, we should not commit the patch. If the patch isn't superior to the existing approach in virtually every way, then there is no point in making it possible for end-users to disable with messy GUCs -- it should be reverted. [1] Message: http://www.postgresql.org/message-id/CAM3SWZRiHaF7jdf923ZZ2qhDJiErqP5uU_+JPuMvUmeD0z9fFA@mail.gmail.com Attachment: http://www.postgresql.org/message-id/attachment/39660/quicksort_external_test.txt [2] http://www.postgresql.org/message-id/CAM3SWZTzLT5Y=VY320NznAyz2z_em3us6x=7rXMEUma9Z9yN6Q@mail.gmail.com [3] http://www.postgresql.org/message-id/CAM3SWZTX5=nHxPpogPirQsH4cR+BpQS6r7Ktax0HMQiNLf-1qA@mail.gmail.com -- Peter Geoghegan
On 25 November 2015 at 00:33, Peter Geoghegan <pg@heroku.com> wrote:
--
Parallel sort is very important. Robert, Amit and I had a call about
this earlier today. We're all in agreement that this should be
extended in that direction, and have a rough idea about how it ought
to fit together with the parallelism primitives. Parallel sort in 9.6
could certainly happen -- that's what I'm aiming for. I haven't really
done preliminary research yet; I'll know more in a little while.
Glad to hear it, I was hoping to see that.
> How about we commit it with a sort_algorithm = 'foo' parameter so we can
> compare things before release of 9.6?
I had a debug GUC (like the existing one to disable top-N heapsorts)
that disabled "quicksort with spillover". That's almost the opposite
of what you're asking for, though, because that makes us never use a
heap. You're asking for me to write a GUC to always use a heap.
I'm asking for a parameter to confirm results from various algorithms, so we can get many eyeballs to confirm your work across its breadth. This is similar to the original trace_sort parameter which we used to confirm earlier sort improvements. I trust it will show this is good and can be removed prior to release of 9.6.
Simon Riggs http://www.2ndQuadrant.com/
PostgreSQL Development, 24x7 Support, Remote DBA, Training & Services
PostgreSQL Development, 24x7 Support, Remote DBA, Training & Services
On Tue, Nov 24, 2015 at 4:46 PM, Simon Riggs <simon@2ndquadrant.com> wrote: >> I had a debug GUC (like the existing one to disable top-N heapsorts) >> that disabled "quicksort with spillover". That's almost the opposite >> of what you're asking for, though, because that makes us never use a >> heap. You're asking for me to write a GUC to always use a heap. > > > I'm asking for a parameter to confirm results from various algorithms, so we > can get many eyeballs to confirm your work across its breadth. This is > similar to the original trace_sort parameter which we used to confirm > earlier sort improvements. I trust it will show this is good and can be > removed prior to release of 9.6. My patch updates trace_sort messages. trace_sort doesn't change the behavior of anything. The only time we've ever done anything like this was for Top-N heap sorts. This is significantly more inconvenient than you think. See the comments in the new dumpbatch() function. -- Peter Geoghegan
On Wed, Nov 25, 2015 at 12:33 AM, Peter Geoghegan <pg@heroku.com> wrote: > On Tue, Nov 24, 2015 at 3:32 PM, Simon Riggs <simon@2ndquadrant.com> wrote: >> My feeling is that numbers rarely speak for themselves, without LSD. (Which >> numbers?) > > Guffaw. Actually I kind of agree. What I would like to see is a series of numbers for increasing sizes of sorts plotted against the same series for the existing algorithm. Specifically with the sort size varying to significantly more than the physical memory on the machine. For example on a 16GB machine sorting data ranging from 1GB to 128GB. There's a lot more information in a series of numbers than individual numbers. We'll be able to see whether all our pontificating about the rates of growth of costs of different algorithms or which costs dominate at which scales are actually borne out in reality. And see where the break points are where I/O overtakes memory costs. And it'll be clearer where to look for problematic cases where the new algorithm might not dominate the old one. -- greg
On Tue, Nov 24, 2015 at 5:42 PM, Greg Stark <stark@mit.edu> wrote: > Actually I kind of agree. What I would like to see is a series of > numbers for increasing sizes of sorts plotted against the same series > for the existing algorithm. Specifically with the sort size varying to > significantly more than the physical memory on the machine. For > example on a 16GB machine sorting data ranging from 1GB to 128GB. There already was a test case involving a 1TB/16 billion tuple sort [1] (well, a 1TB gensort Postgres table [2]). Granted, I don't have a large number of similar test cases across a variety of scales, but there are only so many hours in the day. Disappointingly, the results at that scale were merely good, not great, but there was probably various flaws in how representative the hardware used was. > There's a lot more information in a series of numbers than individual > numbers. We'll be able to see whether all our pontificating about the > rates of growth of costs of different algorithms or which costs > dominate at which scales are actually borne out in reality. You yourself said that 1GB is sufficient to get a single-pass merge phase for a sort of about 4TB - 8TB, so I think the discussion of the growth in costs tells us plenty about what can happen at the high end. My approach might help less overall, but it certainly won't falter. See the 1TB test case -- output from trace_sort is all there. > And see > where the break points are where I/O overtakes memory costs. And it'll > be clearer where to look for problematic cases where the new algorithm > might not dominate the old one. I/O doesn't really overtake memory cost -- if it does, then it should be worthwhile to throw more sequential I/O bandwidth at the problem, which is a realistic, economical solution with a mature implementation (unlike buying more memory bandwidth). I didn't do that with the 1TB test case. If you assume, as cost_sort() does, that it takes N log2(N) comparisons to sort some tuples, then it breaks down like this: 10 items require 33 comparisons, ratio 3.32192809489 100 items require 664 comparisons, ratio 6.64385618977 1,000 items require 9,965 comparisons, ratio 9.96578428466 1,000,000 items require 19,931,568 comparisons, ratio 19.9315685693 1,000,000,000 items require 29,897,352,853 comparisons, ratio 29.897352854 16,000,000,000 items require 542,357,645,663 comparisons, ratio 33.897352854 The cost of writing out and reading runs should be more or less in linear proportion to their size, which is a totally different story. That's the main reason why "quicksort with spillover" is aimed at relatively small sorts, which we expect more of overall. I think the big issue is that a non-parallel sort is significantly under-powered when you go to sort 16 billion tuples. It's probably not very sensible to do so if you have a choice of parallelizing the sort. There is no plausible way to do replacement selection in parallel, since you cannot know ahead of time with any accuracy where to partition workers, as runs can end up arbitrarily larger than memory with presorted inputs. That might be the single best argument for what I propose to do here. This is what Corey's case showed for the final run with 30GB maintenance_work_mem: LOG: starting quicksort of run 40: CPU 1815.99s/19339.80u sec elapsed 24910.38 sec LOG: finished quicksorting run 40: CPU 1820.09s/19565.94u sec elapsed 25140.69 sec LOG: finished writing run 40 to tape 39: CPU 1833.76s/19642.11u sec elapsed 25234.44 sec (Note that the time taken to copy tuples comprising the final run is not displayed or accounted for) This is the second last run, run 40, so it uses the full 30GB of maintenance_work_mem. We spend 00:01:33.75 writing the run. However, we spent 00:03:50.31 just sorting the run. That's roughly the same ratio that I see on my laptop with far smaller runs. I think the difference isn't wider because the server is quite I/O bound -- but we could fix that by adding more disks. [1] http://www.postgresql.org/message-id/CAM3SWZQtdd=Q+EF1xSZaYG1CiOYQJ7sZFcL08GYqChpJtGnKMg@mail.gmail.com [2] https://github.com/petergeoghegan/gensort -- Peter Geoghegan
On Tue, Nov 24, 2015 at 6:31 PM, Peter Geoghegan <pg@heroku.com> wrote: > (Note that the time taken to copy tuples comprising the final run is > not displayed or accounted for) I mean, comprising the second last run, the run shown, run 40. -- Peter Geoghegan
On Wed, Nov 25, 2015 at 2:31 AM, Peter Geoghegan <pg@heroku.com> wrote: > > There already was a test case involving a 1TB/16 billion tuple sort > [1] (well, a 1TB gensort Postgres table [2]). Granted, I don't have a > large number of similar test cases across a variety of scales, but > there are only so many hours in the day. Disappointingly, the results > at that scale were merely good, not great, but there was probably > various flaws in how representative the hardware used was. That's precisely why it's valuable to see a whole series of data points rather than just one. Often when you see the shape of the curve, especially any breaks or changes in the behaviour that helps understand the limitations of the model. Perhaps it would be handy to find a machine with a very small amount of physical memory so you could run more reasonably sized tests on it. A VM would be fine if you could be sure the storage layer isn't caching. In short, I think you're right in theory and I want to make sure you're right in practice. I'm afraid if we just look at a few data points we'll miss out on a bug or a factor we didn't anticipate that could have been addressed. Just to double check though. My understanding is that your quicksort algorithm is to fill work_mem with tuples, quicksort them, write out a run, and repeat. When the inputs are done read work_mem/runs worth of tuples from each run into memory and run a merge (using a heap?) like we do currently. Is that right? Incidentally one of the reasons abandoning the heap to generate runs is attractive is that it opens up other sorting algorithms for us. Instead of quicksort we might be able to plug in a GPU sort for example. -- greg
On Wed, Nov 25, 2015 at 4:10 AM, Greg Stark <stark@mit.edu> wrote: > That's precisely why it's valuable to see a whole series of data > points rather than just one. Often when you see the shape of the > curve, especially any breaks or changes in the behaviour that helps > understand the limitations of the model. Perhaps it would be handy to > find a machine with a very small amount of physical memory so you > could run more reasonably sized tests on it. A VM would be fine if you > could be sure the storage layer isn't caching. I have access to the Power7 system that Robert and others sometimes use for this stuff. I'll try to come up a variety of tests. > In short, I think you're right in theory and I want to make sure > you're right in practice. I'm afraid if we just look at a few data > points we'll miss out on a bug or a factor we didn't anticipate that > could have been addressed. I am in favor of being comprehensive. > Just to double check though. My understanding is that your quicksort > algorithm is to fill work_mem with tuples, quicksort them, write out a > run, and repeat. When the inputs are done read work_mem/runs worth of > tuples from each run into memory and run a merge (using a heap?) like > we do currently. Is that right? Yes, that's basically what I'm doing. There are basically two extra bits: * Without changing how merging actually works, I am clever about allocating memory for the final on-the-fly merge. Allocation is done once, in one huge batch. Importantly, I exploit locality by having every "tuple proper" (e.g. IndexTuple) in contiguous memory, in sorted (tape) order, per tape. This also greatly reduces palloc() overhead for the final on-the-fly merge step. * We do something special when we're just over work_mem, to avoid most I/O -- "quicksort with spillover". This is a nice trick, but it's certain way less important than the basic idea of simply always quicksorting runs. I could easily not do this. This is why the heap code was not significantly simplified to only cover the merge cases, though -- this uses essentially the same replacement selection style heap to incrementally spill to get us enough memory to mostly complete the sort internally. > Incidentally one of the reasons abandoning the heap to generate runs > is attractive is that it opens up other sorting algorithms for us. > Instead of quicksort we might be able to plug in a GPU sort for > example. Yes, it's true that we automatically benefit from optimizations for the internal sort case now. That's already happening with the patch, actually -- the "onlyKey" optimization (a more specialized quicksort specialization, used in the one attribute heap case, and datum case) is now automatically used. That was where the best 2012 numbers for SortSupport were seen, so that makes a significant difference. As you say, something like that could easily happen again. -- Peter Geoghegan
On Wed, Nov 18, 2015 at 3:29 PM, Peter Geoghegan <pg@heroku.com> wrote: > On Wed, Nov 18, 2015 at 10:31 AM, Jeff Janes <jeff.janes@gmail.com> wrote: > >> I agree we don't want to optimize for low memory, but I don't think we >> should throw it under the bus, either. Right now we are effectively >> saying the CPU-cache problems with the heap start exceeding the larger >> run size benefits at 64kb (the smallest allowed setting for work_mem). >> While any number we pick is going to be a guess that won't apply to >> all hardware, surely we can come up with a guess better than 64kb. >> Like, 8 MB, say. If available memory for the sort is 8MB or smaller >> and the predicted size anticipates a multipass merge, then we can use >> the heap method rather than the quicksort method. Would a rule like >> that complicate things much? > > I'm already using replacement selection for the first run when it is > predicted by my new ad-hoc cost model that we can get away with a > "quicksort with spillover", avoiding almost all I/O. We only > incrementally spill as many tuples as needed right now, but it would > be pretty easy to not quicksort the remaining tuples, but continue to > incrementally spill everything. So no, it wouldn't be too hard to hang > on to the old behavior sometimes, if it looked worthwhile. > > In principle, I have no problem with doing that. Through testing, I > cannot see any actual upside, though. Perhaps I just missed something. > Even 8MB is enough to avoid the multipass merge in the event of a > surprisingly high volume of data (my work laptop is elsewhere, so I > don't have my notes on this in front of me, but I figured out the > crossover point for a couple of cases). For me very large sorts (100,000,000 ints) with work_mem below 4MB do better with unpatched than with your patch series, by about 5%. Not a big deal, but also if it is easy to keep the old behavior then I think we should. Yes, it is dumb to do large sorts with work_mem below 4MB, but if you have canned apps which do a mixture of workloads it is not so easy to micromanage their work_mem. Especially as there are no easy tools that let me as the DBA say "if you connect from this IP address, you get this work_mem". I didn't collect trace_sort on those ones because of the high volume it would generate. > >>> In theory, the answer could be "yes", but it seems highly unlikely. >>> Not only is very little memory required to avoid a multi-pass merge >>> step, but as described above the amount required grows very slowly >>> relative to linear growth in input. I propose to add a >>> checkpoint_warning style warning (with a checkpoint_warning style GUC >>> to control it). >> >> I'm skeptical about a warning for this. > > Other systems expose this explicitly, and, as I said, say in an > unqualified way that a multi-pass merge should be avoided. Maybe the > warning isn't the right way of communicating that message to the DBA > in detail, but I am confident that it ought to be communicated to the > DBA fairly clearly. I thinking about how many other places in the code could justify a similar type of warning "If you just gave me 15% more memory, this hash join would be much faster", and what that would make the logs look like if future work went along with this precedence. If there were some mechanism to put the warning in a system view counter instead of the log file, that would be much cleaner. Or a way to separate the server log file into streams. But since we don't have those, I guess I can't really object much to the proposed behavior. > >> One idea would be to stop and write out a just-sorted partition >> whenever that partition is contiguous to the already-written portion. >> If the qsort is tweaked to recurse preferentially into the left >> partition first, this would result in tuples being written out at a >> pretty study pace. If the qsort was unbalanced and the left partition >> was always the larger of the two, then that approach would have to be >> abandoned at some point. But I think there are already defenses >> against that, and at worst you would give up and revert to the >> sort-them-all then write-them-all behavior. > > Seems kind of invasive. I agree, but I wonder if it won't become much more important at 30GB of work_mem. Of course if there is no reason to ever set work_mem that high, then it wouldn't matter--but there is always a reason to do so, if you have so much memory to spare. So better than that invasive work, I guess would be to make sort use less than work_mem if it gets no benefit from using all of it. Anyway, ideas for future work, either way. > >> Overall this is very nice. Doing some real world index builds of >> short text (~20 bytes ascii) identifiers, I could easily get speed ups >> of 40% with your patch if I followed the philosophy of "give it as >> much maintenance_work_mem as I can afford". If I fine-tuned the >> maintenance_work_mem so that it was optimal for each sort method, then >> the speed up quite a bit less, only 22%. But 22% is still very >> worthwhile, and who wants to spend their time fine-tuning the memory >> use for every index build? > > Thanks, but I expected better than that. Was it a collated text > column? The C collation will put the patch in a much better light > (more strcoll() calls are needed with this new approach -- it's still > well worth it, but it is a downside that makes collated text not > especially sympathetic). Just sorting on an integer attribute is also > a good sympathetic case, FWIW. It was UTF8 encoded (although all characters were actually ASCII), but C collated. I've never seen improvements of 3 fold or more like you saw, under any conditions, so I wonder if your test machine doesn't have unusually slow main memory. > > How much time did the sort take in each case? How many runs? How much > time was spent merging? trace_sort output is very interesting here. My largest test, which took my true table and extrapolated it out for a few years growth, had about 500,000,000 rows. At 3GB maintainance_work_mem, it took 13 runs patched and 7 runs unpatched to build the index, with timings of 3168.66 sec and 5713.07 sec. The final merging is intermixed with whatever other work goes on to build the actual index files out of the sorted data, so I don't know exactly what the timing of just the merge part was. But it was certainly a minority of the time, even if you assume the actual index build were free. For the patched code, the majority of the time goes to the quick sorting stages. When I test each version of the code at its own most efficient maintenance_work_mem, I get 3007.2 seconds at 1GB for patched and 3836.46 seconds at 64MB for unpatched. I'm attaching the trace_sort output from the client log for all 4 of those scenarios. "sort_0005" means all 5 of your patches were applied, "origin" means none of them were. Cheers, Jeff
Attachment
On Thu, Nov 19, 2015 at 12:35 PM, Greg Stark <stark@mit.edu> wrote: > On Thu, Nov 19, 2015 at 6:56 PM, Peter Geoghegan <pg@heroku.com> wrote: >> Yes, I really do mean it when I say that the DBA is not supposed to >> see this message, no matter how much or how little memory or data is >> involved. There is no nuance intended here; it isn't sensible to allow >> a multi-pass sort, just as it isn't sensible to allow checkpoints >> every 5 seconds. Both of those things can be thought of as thrashing. > > Hm. So a bit of back-of-envelope calculation. If we have want to > buffer at least 1MB for each run -- I think we currently do more > actually -- and say that a 1GB work_mem ought to be enough to run > reasonably (that's per sort after all and there might be multiple > sorts to say nothing of other users on the system). That means we can > merge about 1,000 runs in the final merge. Each run will be about 2GB > currently but 1GB if we quicksort the runs. So the largest table we > can sort in a single pass is 1-2 TB. > > If we go above those limits we have the choice of buffering less per > run or doing a whole second pass through the data. If we only go slightly above the limits, it is much more graceful. It will happily do a 3 way merge followed by a 1023 way final merge (or something like that) so only 0.3 percent of the data needs a second pass, not all of it. If course by the time you get a factor of 2 over the limit, you are making an entire second pass one way or another. Cheers, Jeff
On Sat, Nov 28, 2015 at 2:04 PM, Jeff Janes <jeff.janes@gmail.com> wrote: > For me very large sorts (100,000,000 ints) with work_mem below 4MB do > better with unpatched than with your patch series, by about 5%. Not a > big deal, but also if it is easy to keep the old behavior then I think > we should. Yes, it is dumb to do large sorts with work_mem below 4MB, > but if you have canned apps which do a mixture of workloads it is not > so easy to micromanage their work_mem. Especially as there are no > easy tools that let me as the DBA say "if you connect from this IP > address, you get this work_mem". I'm not very concerned about a regression that is only seen when work_mem is set below the (very conservative) postgresql.conf default value of 4MB when sorting 100 million integers. Thank you for characterizing the regression, though -- it's good to have a better idea of how much of a problem that is in practice. I can still preserve the old behavior with a GUC, but it isn't completely trivial, and I don't want to complicate things any further without a real benefit, which I still don't see. I'm still using a replacement selection style heap, and I think that there will be future uses for the heap (e.g. dynamic duplicate removal within tuplesort), though. >> Other systems expose this explicitly, and, as I said, say in an >> unqualified way that a multi-pass merge should be avoided. Maybe the >> warning isn't the right way of communicating that message to the DBA >> in detail, but I am confident that it ought to be communicated to the >> DBA fairly clearly. > > I thinking about how many other places in the code could justify a > similar type of warning "If you just gave me 15% more memory, this > hash join would be much faster", and what that would make the logs > look like if future work went along with this precedence. If there > were some mechanism to put the warning in a system view counter > instead of the log file, that would be much cleaner. Or a way to > separate the server log file into streams. But since we don't have > those, I guess I can't really object much to the proposed behavior. I'm going to let this go, actually. Not because I don't think that avoiding a multi-pass sort is a good goal for DBAs to have, but because a multi-pass sort doesn't appear to be a point at which performance tanks these days, with modern block devices. Also, I just don't have time to push something non-essential that there is resistance to. >>> One idea would be to stop and write out a just-sorted partition >>> whenever that partition is contiguous to the already-written portion. >>> If the qsort is tweaked to recurse preferentially into the left >>> partition first, this would result in tuples being written out at a >>> pretty study pace. If the qsort was unbalanced and the left partition >>> was always the larger of the two, then that approach would have to be >>> abandoned at some point. But I think there are already defenses >>> against that, and at worst you would give up and revert to the >>> sort-them-all then write-them-all behavior. >> >> Seems kind of invasive. > > I agree, but I wonder if it won't become much more important at 30GB > of work_mem. Of course if there is no reason to ever set work_mem > that high, then it wouldn't matter--but there is always a reason to do > so, if you have so much memory to spare. So better than that invasive > work, I guess would be to make sort use less than work_mem if it gets > no benefit from using all of it. Anyway, ideas for future work, > either way. I hope to come up with a fairly robust model for automatically sizing an "effective work_mem" in the context of external sorts. There should be a heuristic that balances fan-in against other considerations. I think that doing this with the existing external sort code would be completely hopeless. This is a problem that is well understood by the research community, although balances things well in the context of PostgreSQL is a little trickier. I also think it's a little arbitrary that the final on-the-fly merge step uses a work_mem-ish sized buffer, much like the sorting of runs, as if there is a good reason to be consistent. Maybe that's fine, though. There are advantages to returning tuples earlier in the context of parallelism, which recommends smaller effective work_mem sizes (provided they're above a certain threshold). For this reason, having larger runs may not be a useful goal in general, even without considering the cost in cache misses paid in the pursuit that goal. >> Thanks, but I expected better than that. Was it a collated text >> column? The C collation will put the patch in a much better light >> (more strcoll() calls are needed with this new approach -- it's still >> well worth it, but it is a downside that makes collated text not >> especially sympathetic). Just sorting on an integer attribute is also >> a good sympathetic case, FWIW. > > It was UTF8 encoded (although all characters were actually ASCII), but > C collated. I think that I should have considered that you'd hand-optimized the work_mem setting for each case in reacting here -- I was at a conference when I responded. You can show the existing code in a better light by doing that, as you have, but I think it's all but irrelevant. It isn't even practical for experts to do that, so the fact that it is possible is only really a footnote. My choice of work_mem for my tests tended to be round numbers, like 1GB, because that was the first thing I thought of. > I've never seen improvements of 3 fold or more like you saw, under any > conditions, so I wonder if your test machine doesn't have unusually > slow main memory. I think that there is a far simpler explanation. Any time I reported a figure over ~2.5x, it was for "quicksort with spillover", and with a temp tablespace on tmpfs to simulate lots of I/O bandwidth (but with hardly any actual writing to tape -- that's the whole point of that case). I also think that the heap structure does very badly with low cardinality sets, which is where the 3.25X - 4X numbers came from. You haven't tested "quicksort with spillover" here at all, which is fine, since it is less important. Finally, as I said, I did not give the master branch the benefit of fine-tuning work_mem (which I think is fair and representative). > My largest test, which took my true table and extrapolated it out for > a few years growth, had about 500,000,000 rows. Cool. > At 3GB maintainance_work_mem, it took 13 runs patched and 7 runs > unpatched to build the index, with timings of 3168.66 sec and 5713.07 > sec. > > The final merging is intermixed with whatever other work goes on to > build the actual index files out of the sorted data, so I don't know > exactly what the timing of just the merge part was. But it was > certainly a minority of the time, even if you assume the actual index > build were free. For the patched code, the majority of the time goes > to the quick sorting stages. I'm not sure what you mean here. I agree that the work of (say) inserting leaf tuples as part of an index build is kind of the same cost as the merge step itself, or doesn't vary markedly between the CREATE INDEX case, and other cases (where there is some analogous processing of final sorted output). I would generally expect that the merge phase takes significantly less than sorting runs, regardless of how we sort runs, unless parallelism is involved, where merging could dominate. The master branch has a faster merge step, at least proportionally, because it has larger runs. > When I test each version of the code at its own most efficient > maintenance_work_mem, I get > 3007.2 seconds at 1GB for patched and 3836.46 seconds at 64MB for unpatched. As I said, it seems a little bit unfair to hand-tune work_mem or maintenance_work_mem like that. Who can afford to do that? I think you agree that it's untenable to have DBAs allocate work_mem differently for cases where an internal sort or external sort is expected; workloads are just far too complicated and changeable. > I'm attaching the trace_sort output from the client log for all 4 of > those scenarios. "sort_0005" means all 5 of your patches were > applied, "origin" means none of them were. Thanks for looking at this. This is very helpful. It looks like the server you used here had fairly decent disks, and that we tended to be CPU bound more often than not. That's a useful testing ground. Consider run #7 (of 13 total) with 3GB maintenance_work_mem, for example (this run was picked at random): ... LOG: finished writing run 6 to tape 5: CPU 35.13s/1028.44u sec elapsed 1080.43 sec LOG: starting quicksort of run 7: CPU 38.15s/1051.68u sec elapsed 1108.19 sec LOG: finished quicksorting run 7: CPU 38.16s/1228.09u sec elapsed 1284.87 sec LOG: finished writing run 7 to tape 6: CPU 40.21s/1235.36u sec elapsed 1295.19 sec LOG: starting quicksort of run 8: CPU 42.73s/1257.59u sec elapsed 1321.09 sec ... So there was 27.76 seconds spent copying tuples into local memory ahead of the quicksort, 2 minutes 56.68 seconds spent actually quicksorting, and a trifling 10.32 seconds actually writing the run! I bet that the quicksort really didn't use up too much memory bandwidth on the system as a whole, since abbreviated keys are used with a cache oblivious internal sorting algorithm. This suggests that this case would benefit rather a lot from parallel workers doing this for each run at the same time (once my code is adopted to do that, of course). This is something I'm currently researching. I think that (roughly speaking) each core on this system is likely slower than the cores on a 4-core consumer desktop/laptop, which is very normal, particularly with x86_64 systems. That also makes it more representative than my previous tests. -- Peter Geoghegan
On Sat, Nov 28, 2015 at 4:05 PM, Peter Geoghegan <pg@heroku.com> wrote: > So there was 27.76 seconds spent copying tuples into local memory > ahead of the quicksort, 2 minutes 56.68 seconds spent actually > quicksorting, and a trifling 10.32 seconds actually writing the run! I > bet that the quicksort really didn't use up too much memory bandwidth > on the system as a whole, since abbreviated keys are used with a cache > oblivious internal sorting algorithm. Uh, actually, that isn't so: LOG: begin index sort: unique = f, workMem = 1048576, randomAccess = f LOG: bttext_abbrev: abbrev_distinct after 160: 1.000489 (key_distinct: 40.802210, norm_abbrev_card: 0.006253, prop_card: 0.200000) LOG: bttext_abbrev: aborted abbreviation at 160 (abbrev_distinct: 1.000489, key_distinct: 40.802210, prop_card: 0.200000) Abbreviation is aborted in all cases that you tested. Arguably this should happen significantly less frequently with the "C" locale, possibly almost never, but it makes this case less than representative of most people's workloads. I think that at least the first several hundred leading attribute tuples are duplicates. BTW, roughly what does this CREATE INDEX look like? Is it a composite index, for example? It would also be nice to see pg_stats entries for each column being indexed. Data distributions are certainly of interest here. Thanks -- Peter Geoghegan
On Sun, Nov 29, 2015 at 2:01 AM, Peter Geoghegan <pg@heroku.com> wrote: > I think that at least the first several > hundred leading attribute tuples are duplicates. I mean duplicate abbreviated keys. There are 40 distinct keys overall in the first 160 tuples, which is why abbreviation is aborted -- this can be seen from the trace_sort output, of course. -- Peter Geoghegan
On Sat, Nov 28, 2015 at 02:04:16PM -0800, Jeff Janes wrote: > On Wed, Nov 18, 2015 at 3:29 PM, Peter Geoghegan <pg@heroku.com> wrote: > > On Wed, Nov 18, 2015 at 10:31 AM, Jeff Janes <jeff.janes@gmail.com> wrote: > > > >> I agree we don't want to optimize for low memory, but I don't think we > >> should throw it under the bus, either. Right now we are effectively > >> saying the CPU-cache problems with the heap start exceeding the larger > >> run size benefits at 64kb (the smallest allowed setting for work_mem). > >> While any number we pick is going to be a guess that won't apply to > >> all hardware, surely we can come up with a guess better than 64kb. > >> Like, 8 MB, say. If available memory for the sort is 8MB or smaller > >> and the predicted size anticipates a multipass merge, then we can use > >> the heap method rather than the quicksort method. Would a rule like > >> that complicate things much? > > > > I'm already using replacement selection for the first run when it is > > predicted by my new ad-hoc cost model that we can get away with a > > "quicksort with spillover", avoiding almost all I/O. We only > > incrementally spill as many tuples as needed right now, but it would > > be pretty easy to not quicksort the remaining tuples, but continue to > > incrementally spill everything. So no, it wouldn't be too hard to hang > > on to the old behavior sometimes, if it looked worthwhile. > > > > In principle, I have no problem with doing that. Through testing, I > > cannot see any actual upside, though. Perhaps I just missed something. > > Even 8MB is enough to avoid the multipass merge in the event of a > > surprisingly high volume of data (my work laptop is elsewhere, so I > > don't have my notes on this in front of me, but I figured out the > > crossover point for a couple of cases). > > For me very large sorts (100,000,000 ints) with work_mem below 4MB do > better with unpatched than with your patch series, by about 5%. Not a > big deal, but also if it is easy to keep the old behavior then I think > we should. Yes, it is dumb to do large sorts with work_mem below 4MB, > but if you have canned apps which do a mixture of workloads it is not > so easy to micromanage their work_mem. Especially as there are no > easy tools that let me as the DBA say "if you connect from this IP > address, you get this work_mem". That's certainly doable with pgbouncer, for example. What would you have in mind for the more general capability? It seems to me that bloating up pg_hba.conf would be undesirable, but maybe I'm picturing this as bigger than it actually needs to be. Cheers, David. -- David Fetter <david@fetter.org> http://fetter.org/ Phone: +1 415 235 3778 AIM: dfetter666 Yahoo!: dfetter Skype: davidfetter XMPP: david.fetter@gmail.com Remember to vote! Consider donating to Postgres: http://www.postgresql.org/about/donate
On Sat, Nov 28, 2015 at 4:05 PM, Peter Geoghegan <pg@heroku.com> wrote: > On Sat, Nov 28, 2015 at 2:04 PM, Jeff Janes <jeff.janes@gmail.com> wrote: ... >> >> The final merging is intermixed with whatever other work goes on to >> build the actual index files out of the sorted data, so I don't know >> exactly what the timing of just the merge part was. But it was >> certainly a minority of the time, even if you assume the actual index >> build were free. For the patched code, the majority of the time goes >> to the quick sorting stages. > > I'm not sure what you mean here. I had no point to make here, I was just trying to answer one of your questions about how much time was spent merging. I don't know, because it is interleaved with and not separately instrumented from the index build. > > I would generally expect that the merge phase takes significantly less > than sorting runs, regardless of how we sort runs, unless parallelism > is involved, where merging could dominate. The master branch has a > faster merge step, at least proportionally, because it has larger > runs. > >> When I test each version of the code at its own most efficient >> maintenance_work_mem, I get >> 3007.2 seconds at 1GB for patched and 3836.46 seconds at 64MB for unpatched. > > As I said, it seems a little bit unfair to hand-tune work_mem or > maintenance_work_mem like that. Who can afford to do that? I think you > agree that it's untenable to have DBAs allocate work_mem differently > for cases where an internal sort or external sort is expected; > workloads are just far too complicated and changeable. Right, I agree with all that. But I think it is important to know where the benefits come from. It looks like about half comes from being more robust to overly-large memory usage, and half from absolute improvements which you get at each implementations own best setting. Also, if someone had previously restricted work_mem (or more likely maintenance_work_mem) simply to avoid the large memory penalty, they need to know to revisit that decision. Although they still don't get any actual benefit from using too much memory, just a reduced penalty. I'm kind of curious as to why the optimal for the patched code appears at 1GB and not lower. If I get a chance to rebuild the test, I will look into that more. > >> I'm attaching the trace_sort output from the client log for all 4 of >> those scenarios. "sort_0005" means all 5 of your patches were >> applied, "origin" means none of them were. > > Thanks for looking at this. This is very helpful. It looks like the > server you used here had fairly decent disks, and that we tended to be > CPU bound more often than not. That's a useful testing ground. It has a Perc H710 RAID controller with 15,000 RPM drives, but it is also a virtualized system that has other stuff going on. The disks are definitely better than your average household computer, but I don't think they are anything special as far as real database hardware goes. It is hard to saturate the disks for sequential reads. It will be interesting to see what parallel builds can do. What would be next in reviewing the patches? Digging into the C-level implementation? Cheers, Jeff
On Sun, Nov 29, 2015 at 8:02 PM, David Fetter <david@fetter.org> wrote: >> >> For me very large sorts (100,000,000 ints) with work_mem below 4MB do >> better with unpatched than with your patch series, by about 5%. Not a >> big deal, but also if it is easy to keep the old behavior then I think >> we should. Yes, it is dumb to do large sorts with work_mem below 4MB, >> but if you have canned apps which do a mixture of workloads it is not >> so easy to micromanage their work_mem. Especially as there are no >> easy tools that let me as the DBA say "if you connect from this IP >> address, you get this work_mem". > > That's certainly doable with pgbouncer, for example. I had not considered that. How would you do it with pgbouncer? The think I can think of would be to put it in server_reset_query, which doesn't seem correct. > What would you > have in mind for the more general capability? It seems to me that > bloating up pg_hba.conf would be undesirable, but maybe I'm picturing > this as bigger than it actually needs to be. I would envision something like "ALTER ROLE set ..." only for application_name and IP address instead of ROLE. I have no idea how I would implement that, it is just how I would like to use it as the end user. Cheers, Jeff
On Mon, Nov 30, 2015 at 9:51 AM, Jeff Janes <jeff.janes@gmail.com> wrote: >> As I said, it seems a little bit unfair to hand-tune work_mem or >> maintenance_work_mem like that. Who can afford to do that? I think you >> agree that it's untenable to have DBAs allocate work_mem differently >> for cases where an internal sort or external sort is expected; >> workloads are just far too complicated and changeable. > > Right, I agree with all that. But I think it is important to know > where the benefits come from. It looks like about half comes from > being more robust to overly-large memory usage, and half from absolute > improvements which you get at each implementations own best setting. > Also, if someone had previously restricted work_mem (or more likely > maintenance_work_mem) simply to avoid the large memory penalty, they > need to know to revisit that decision. Although they still don't get > any actual benefit from using too much memory, just a reduced penalty. Well, to be clear, they do get a benefit with much larger memory sizes. It's just that the benefit does not continue indefinitely. I agree with this assessment, though. > I'm kind of curious as to why the optimal for the patched code appears > at 1GB and not lower. If I get a chance to rebuild the test, I will > look into that more. I think that the availability of abbreviated keys (or something that allows most comparisons made by quicksort/the heap to be resolved at the SortTuple level) could make a big difference for things like this. Bear in mind that the merge phase has better cache characteristics when many attributes must be compared, and not mostly just leading attributes. Alphasort [1] merges in-memory runs (built with quicksort) to create on-disk runs for this reason. (I tried that, and it didn't help -- maybe I get that benefit from merging on-disk runs, since modern machines have so much more memory than in 1994). > It has a Perc H710 RAID controller with 15,000 RPM drives, but it is > also a virtualized system that has other stuff going on. The disks > are definitely better than your average household computer, but I > don't think they are anything special as far as real database hardware > goes. What I meant was that it's better than my laptop. :-) > What would be next in reviewing the patches? Digging into the C-level > implementation? Yes, certainly, but let me post a revised version first. I have improved the comments, and performed some consolidation of commits. Also, I am going to get a bunch of test results from the POWER7 system. I think I might see more benefits with higher maintenance_work_mem settings that you saw, primarily because my case can mostly just use abbreviated keys during the quicksort operations. Also, I find it very very useful that while (for example) your 3GB test case was slower than your 1GB test case, it was only 5% slower. I have a lot of hope that we can have a cost model for sizing an effective maintenance_work_mem for this reason -- the consequences of being wrong are really not that severe. It's unfortunate that we currently waste so much memory by blindly adhering to work_mem/maintenance_work_mem. This matters a lot more when we have parallel sort. [1] http://www.cs.berkeley.edu/~rxin/db-papers/alphasort.pdf -- Peter Geoghegan
On Mon, Nov 30, 2015 at 12:29 PM, Peter Geoghegan <pg@heroku.com> wrote: >> I'm kind of curious as to why the optimal for the patched code appears >> at 1GB and not lower. If I get a chance to rebuild the test, I will >> look into that more. > > I think that the availability of abbreviated keys (or something that > allows most comparisons made by quicksort/the heap to be resolved at > the SortTuple level) could make a big difference for things like this. Using the Hydra POWER7 server [1] + the gensort benchmark [2], which uses the C collation, and has abbreviated keys that have lots of entropy, I see benefits with higher and higher maintenance_work_mem settings. I will present a variety of cases, which seemed like something Greg Stark is particularly interested in. On the whole, I am quite pleased with how things are shown to be improved in a variety of different scenarios. Looking at CREATE INDEX build times on an (unlogged) gensort table with 50 million, 100 million, 250 million, and 500 million tuples, with maintenance_work_mem settings of 512MB, 1GB, 10GB, and 15GB, there are sustained improvements as more memory is made available. I'm not saying that that would be the case with low cardinality leading attribute tuples -- probably not -- but it seems pretty nice that this case can sustain improvements as more memory is made available. The server used here has reasonably good disks (Robert goes into this in his blogpost), but nothing spectacular. This is what a 500 million tuple gensort table looks like: postgres=# \dt+ List of relations Schema | Name | Type | Owner | Size | Description --------+-----------+-------+-------+-------+------------- public | sort_test | table | pg | 32 GB | (1 row) Results: 50 million tuple table (best of 3): ------------------------------------------ 512MB: (8-way final merge) external sort ended, 171058 disk blocks used: CPU 4.11s/79.30u sec elapsed 83.60 sec 1GB: (4-way final merge) external sort ended, 171063 disk blocks used: CPU 4.29s/71.34u sec elapsed 75.69 sec 10GB: N/A 15GB: N/A 1GB (master branch): (3-way final merge) external sort ended, 171064 disk blocks used: CPU 6.19s/163.00u sec elapsed 170.84 sec 100 million tuple table (best of 3): -------------------------------------------- 512MB: (16-way final merge) external sort ended, 342114 disk blocks used: CPU 8.61s/177.77u sec elapsed 187.03 sec 1GB: (8-way final merge) external sort ended, 342124 disk blocks used: CPU 8.07s/165.15u sec elapsed 173.70 sec 10GB: N/A 15GB: N/A 1GB (master branch): (5-way final merge) external sort ended, 342129 disk blocks used: CPU 11.68s/358.17u sec elapsed 376.41 sec 250 million tuple table (best of 3): -------------------------------------------- 512MB: (39-way final merge) external sort ended, 855284 disk blocks used: CPU 19.96s/486.57u sec elapsed 507.89 sec 1GB: (20-way final merge) external sort ended, 855306 disk blocks used: CPU 22.63s/475.33u sec elapsed 499.09 sec 10GB: (2-way final merge) external sort ended, 855326 disk blocks used: CPU 21.99s/341.34u sec elapsed 366.15 sec 15GB: (2-way final merge) external sort ended, 855326 disk blocks used: CPU 23.23s/322.18u sec elapsed 346.97 sec 1GB (master branch): (11-way final merge) external sort ended, 855315 disk blocks used: CPU 30.56s/973.00u sec elapsed 1015.63 sec 500 million tuple table (best of 3): -------------------------------------------- 512MB: (77-way final merge) external sort ended, 1710566 disk blocks used: CPU 45.70s/1016.70u sec elapsed 1069.02 sec 1GB: (39-way final merge) external sort ended, 1710613 disk blocks used: CPU 44.34s/1013.26u sec elapsed 1067.16 sec 10GB: (4-way final merge) external sort ended, 1710649 disk blocks used: CPU 46.46s/772.97u sec elapsed 841.35 sec 15GB: (3-way final merge) external sort ended, 1710652 disk blocks used: CPU 51.55s/729.88u sec elapsed 809.68 sec 1GB (master branch): (20-way final merge) external sort ended, 1710632 disk blocks used: CPU 69.35s/2013.21u sec elapsed 2113.82 sec I attached a detailed account of these benchmarks, for those that really want to see the nitty-gritty. This includes a 1GB case for patch without memory prefetching (which is not described in this message). [1] http://rhaas.blogspot.com/2012/03/performance-and-scalability-on-ibm.html [2] https://github.com/petergeoghegan/gensort -- Peter Geoghegan
Attachment
Hm. Here is a log-log chart of those results (sorry for html mail). I'm not really sure if log-log is the right tool to use for a O(nlog(n)) curve though.
I think the take-away is that this is outside the domain where any interesting break points occur. Maybe run more tests on the low end to find where the tapesort can generate a single tape and avoid the merge and see where the discontinuity is with quicksort for the various work_mem sizes.
And can you calculate an estimate where the domain would be where multiple passes would be needed for this table at these work_mem sizes? Is it feasible to test around there?
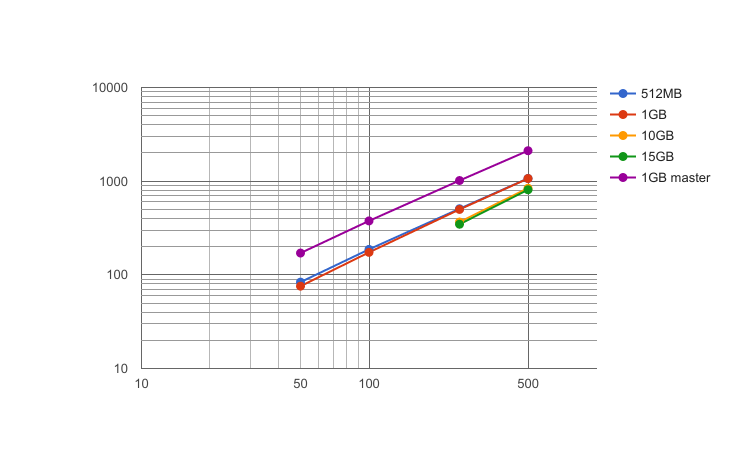
greg
Attachment
On Mon, Nov 30, 2015 at 5:12 PM, Greg Stark <stark@mit.edu> wrote: > I think the take-away is that this is outside the domain where any interesting break points occur. I think that these are representative of what people want to do with external sorts. We have already had Jeff look for a regression. He found one only with less than 4MB of work_mem (the default), with over 100 million tuples. What exactly are we looking for? > And can you calculate an estimate where the domain would be where multiple passes would be needed for this table at thesework_mem sizes? Is it feasible to test around there? Well, you said that 1GB of work_mem was enough to avoid that within about 4TB - 8TB of data. So, I believe the answer is "no": [pg@hydra ~]$ df -h Filesystem Size Used Avail Use% Mounted on rootfs 20G 19G 519M 98% / devtmpfs 31G 128K 31G 1% /dev tmpfs 31G 384K 31G 1% /dev/shm /dev/mapper/vg_hydra-root 20G 19G 519M 98% / tmpfs 31G 127M 31G 1% /run tmpfs 31G 0 31G 0% /sys/fs/cgroup tmpfs 31G 0 31G 0% /media /dev/md0 497M 145M 328M 31% /boot /dev/mapper/vg_hydra-data 1023G 322G 651G 34% /data -- Peter Geoghegan
On Sat, Nov 28, 2015 at 7:05 PM, Peter Geoghegan <pg@heroku.com> wrote: > On Sat, Nov 28, 2015 at 2:04 PM, Jeff Janes <jeff.janes@gmail.com> wrote: >> For me very large sorts (100,000,000 ints) with work_mem below 4MB do >> better with unpatched than with your patch series, by about 5%. Not a >> big deal, but also if it is easy to keep the old behavior then I think >> we should. Yes, it is dumb to do large sorts with work_mem below 4MB, >> but if you have canned apps which do a mixture of workloads it is not >> so easy to micromanage their work_mem. Especially as there are no >> easy tools that let me as the DBA say "if you connect from this IP >> address, you get this work_mem". > > I'm not very concerned about a regression that is only seen when > work_mem is set below the (very conservative) postgresql.conf default > value of 4MB when sorting 100 million integers. Perhaps surprisingly, I tend to agree. I'm cautious of regressions here, but large sorts in queries are relatively uncommon. You're certainly not going to want to return a 100 million tuples to the client. If you're trying to do a merge join with 100 million tuples, well, 100 million integers @ 32 bytes per tuple is 3.2GB, and that's the size of a tuple with a 4 byte integer and at most 4 bytes of other data being carried along with it. So in practice you'd probably need to have at least 5-10GB of data, which means you are trying to sort data over a million times larger than the amount of memory you allowed for the sort. With or without that patch, you should really consider raising work_mem. And maybe create some indexes so that the planner doesn't choose a merge join any more. The aggregate case is perhaps with a little more thought: maybe you are sorting 100 million tuples so that you can GroupAggregate them. But, there again, the benefits of raising work_mem are quite large with or without this patch. Heck, if you're lucky, a little more work_mem might switch you to a HashAggregate. I'm not sure it's worth complicating the code to cater to those cases. While large sorts are uncommon in queries, they are much more common in index builds. Therefore, I think we ought to be worrying more about regressions at 64MB than at 4MB, because we ship with maintenance_work_mem = 64MB and a lot of people probably don't change it before trying to build an index. If we make those index builds go faster, users will be happy. If we make them go slower, users will be sad. So I think it's worth asking the question "are there any CREATE INDEX commands that someone might type on a system on which they've done no other configuration that will be slower with this patch"? -- Robert Haas EnterpriseDB: http://www.enterprisedb.com The Enterprise PostgreSQL Company
On Wed, Dec 2, 2015 at 10:03 AM, Robert Haas <robertmhaas@gmail.com> wrote: >> I'm not very concerned about a regression that is only seen when >> work_mem is set below the (very conservative) postgresql.conf default >> value of 4MB when sorting 100 million integers. > > Perhaps surprisingly, I tend to agree. I'm cautious of regressions > here, but large sorts in queries are relatively uncommon. You're > certainly not going to want to return a 100 million tuples to the > client. Right. The fact that it was only a 5% regression is also a big part of what made me unconcerned. I am glad that we've characterized the regression that I assumed was there, though -- I certainly knew that Knuth and so on were not wrong to emphasize increasing run size in the 1970s. Volume 3 of The Art of Computer Programming literally has a pull-out chart showing the timing of external sorts. This includes the time it takes for a human operator to switch magnetic tapes, and rewind those tapes. The underlying technology has changed rather a lot since, of course. > While large sorts are uncommon in queries, they are much more common > in index builds. Therefore, I think we ought to be worrying more > about regressions at 64MB than at 4MB, because we ship with > maintenance_work_mem = 64MB and a lot of people probably don't change > it before trying to build an index. If we make those index builds go > faster, users will be happy. If we make them go slower, users will be > sad. So I think it's worth asking the question "are there any CREATE > INDEX commands that someone might type on a system on which they've > done no other configuration that will be slower with this patch"? I certainly agree that that's a good place to focus. I think that it's far, far less likely that anything will be slowed down when you take this as a cut-off point. I don't want to overemphasize it, but the analysis of how many more passes are needed because of lack of a replacement selection heap (the "quadratic growth" thing) gives me confidence. A case with less than 4MB of work_mem is where we actually saw *some* regression. -- Peter Geoghegan
On Sun, Nov 29, 2015 at 2:01 AM, Peter Geoghegan <pg@heroku.com> wrote: > On Sat, Nov 28, 2015 at 4:05 PM, Peter Geoghegan <pg@heroku.com> wrote: >> So there was 27.76 seconds spent copying tuples into local memory >> ahead of the quicksort, 2 minutes 56.68 seconds spent actually >> quicksorting, and a trifling 10.32 seconds actually writing the run! I >> bet that the quicksort really didn't use up too much memory bandwidth >> on the system as a whole, since abbreviated keys are used with a cache >> oblivious internal sorting algorithm. > > Uh, actually, that isn't so: > > LOG: begin index sort: unique = f, workMem = 1048576, randomAccess = f > LOG: bttext_abbrev: abbrev_distinct after 160: 1.000489 > (key_distinct: 40.802210, norm_abbrev_card: 0.006253, prop_card: > 0.200000) > LOG: bttext_abbrev: aborted abbreviation at 160 (abbrev_distinct: > 1.000489, key_distinct: 40.802210, prop_card: 0.200000) > > Abbreviation is aborted in all cases that you tested. Arguably this > should happen significantly less frequently with the "C" locale, > possibly almost never, but it makes this case less than representative > of most people's workloads. I think that at least the first several > hundred leading attribute tuples are duplicates. I guess I wasn't paying sufficient attention to that part of trace_sort, I was not familiar enough with the abbreviation feature to interpret what it meant. I had thought we used 16 bytes for abbreviation, but now I see it is only 8 bytes. My column has the format of ABC-123-456-789-0 The name-space identifier ("ABC-") is the same in 99.99% of the cases. And to date, as well as in my extrapolation, the first two digits of the numeric part are leading zeros and the third one is mostly 0,1,2. So the first 8 bytes really have less than 2 bits worth of information. So yeah, not surprising abbreviation was not useful. (When I created the system, I did tests that showed it doesn't make much difference whether I used the format natively, or stripped it to something more compact on input and reformatted it on output. That was before abbreviation features existed) > > BTW, roughly what does this CREATE INDEX look like? Is it a composite > index, for example? Nope, just a single column index. In the extrapolated data set, each distinct value shows up a couple hundred times on average. I'm thinking of converting it to a btree_gin index once I've tested them a bit more, as the compression benefits are substantial. Cheers, Jeff
On Sun, Dec 6, 2015 at 3:59 PM, Jeff Janes <jeff.janes@gmail.com> wrote: > My column has the format of ABC-123-456-789-0 > > The name-space identifier ("ABC-") is the same in 99.99% of the > cases. And to date, as well as in my extrapolation, the first two > digits of the numeric part are leading zeros and the third one is > mostly 0,1,2. So the first 8 bytes really have less than 2 bits worth > of information. So yeah, not surprising abbreviation was not useful. I think that given you're using the "C" collation, abbreviation should still go ahead. I posted a patch to do that, which I need to further justify per Robert's request (currently, we do nothing special based on collation). Abbreviation should help in surprisingly marginal cases, since far fewer memory accesses will be required in the early stages of the sort with only (say) 5 distinct abbreviated keys. Once abbreviated comparisons start to not help at all (with quicksort, at some partition), there's a good chance that the full keys can be reused to some extent, before being evicted from CPU caches. >> BTW, roughly what does this CREATE INDEX look like? Is it a composite >> index, for example? > > Nope, just a single column index. In the extrapolated data set, each > distinct value shows up a couple hundred times on average. I'm > thinking of converting it to a btree_gin index once I've tested them a > bit more, as the compression benefits are substantial. Unfortunately, that cannot use tuplesort.c at all. -- Peter Geoghegan
On Tue, Nov 24, 2015 at 4:33 PM, Peter Geoghegan <pg@heroku.com> wrote: > So, the bottom line is: This patch seems very good, is unlikely to > have any notable downside (no case has been shown to be regressed), > but has yet to receive code review. I am working on a new version with > the first two commits consolidated, and better comments, but that will > have the same code, unless I find bugs or am dissatisfied. It mostly > needs thorough code review, and to a lesser extent some more > performance testing. I'm currently spending a lot of time working on parallel CREATE INDEX. I should not delay posting a new version of my patch series any further, though. I hope to polish up parallel CREATE INDEX to be able to show people something in a couple of weeks. This version features consolidated commits, the removal of the multipass_warning parameter, and improved comments and commit messages. It has almost entirely unchanged functionality. The only functional changes are: * The function useselection() is taught to distrust an obviously bogus caller reltuples hint (when it's already less than half of what we know to be the minimum number of tuples that the sort must sort, immediately after LACKMEM() first becomes true -- this is probably a generic estimate). * Prefetching only occurs when writing tuples. Explicit prefetching appears to hurt in some cases, as David Rowley has shown over on the dedicated thread. But it might still be that writing tuples is a case that is simple enough to benefit consistently, due to the relatively uniform processing that memory latency can hide behind for that case (before, the same prefetching instructions were used for CREATE INDEX and for aggregates, for example). Maybe we should consider trying to get patch 0002 (the memory pool/merge patch) committed first, something Greg Stark suggested privately. That might actually be an easier way of integrating this work, since it changes nothing about the algorithm we use for merging (it only improves memory locality), and so is really an independent piece of work (albeit one that makes a huge overall difference due to the other patches increasing the time spent merging in absolute terms, and especially as a proportion of the total). -- Peter Geoghegan
Attachment
On Tue, Nov 24, 2015 at 4:46 PM, Simon Riggs <simon@2ndquadrant.com> wrote: >> Parallel sort is very important. Robert, Amit and I had a call about >> this earlier today. We're all in agreement that this should be >> extended in that direction, and have a rough idea about how it ought >> to fit together with the parallelism primitives. Parallel sort in 9.6 >> could certainly happen -- that's what I'm aiming for. I haven't really >> done preliminary research yet; I'll know more in a little while. > > Glad to hear it, I was hoping to see that. As I mentioned just now, I'm working on parallel CREATE INDEX currently, which seems like a good proving ground for parallel sort, as its where the majority of really expensive sorts occur. It would be nice to get parallel-aware sort nodes in 9.6, but I don't think I'll be able to make that happen in time. The required work in the optimizer is just too complicated. The basic idea is that we use the parallel heapam interface, and have backends sort and write runs as with an external sort (if those runs are would-be internal sorts, we still write them to tape in the manner of external sorts). When done, worker processes release memory, but not tapes, initially. The leader reassembles an in-memory representation of the tapes that is basically consistent with it having generated those runs itself (using my new approach to external sorting). Then, it performs an on-the-fly merge, as before. At the moment, I have the sorting of runs within workers using the parallel heapam interface more or less working, with workers dumping out the runs to tape. I'll work on reassembling the state of the tapes within the leader in the coming week. It's all still rather rough, but I think I'll have benchmarks before people start taking time off later in the month, and possibly even code. Cutting the scope of parallel sort in 9.6 to only cover parallel CREATE INDEX will make it likely that I'll be able to deliver something acceptable for that release. -- Peter Geoghegan
So incidentally I've been running some benchmarks myself. Mostly to understand the current scaling behaviour of sorting to better judge whether Peter's analysis of where the pain points are and why we should not worry about optimizing for the multiple merge pass case were on target. I haven't actually benchmarked his patch at all, just stock head so far.
The really surprising result (for me) so far is that apparently merge passes spent actually very little time doing I/O. I had always assumed most of the time was spent waiting on I/O and that's why we spend so much effort ensuring sequential I/O and trying to maximize run lengths. I was expecting to see a huge step increase in the total time whenever there was an increase in merge passes. However I see hardly any increase, sometimes even a decrease despite the extra pass. The time generally increases as work_mem decreases but the slope is pretty moderate and gradual with no big steps due to extra passes.
On further analysis I'm less surprised by this than previously. The larger benchmarks I'm running are on a 7GB table which only actually generates 2.6GB of sort data so even writing all that out and then reading it all back in on a 100MB/s disk would only take an extra 50s. That won't make a big dent when the whole sort takes about 30 minutes. Even if you assume there's a substantial amount of random I/O it'll only be a 10% difference or so which is more or less in line with what I'm seeing.
I haven't actually got to benchmarking Peter's patch at all but this is reinforcing his argument dramatically. If the worst case for using quicksort is that the shorter runs might push us into doing an extra merge and that might add an extra 10% to the run-time that will be easily counter-balanced by the faster quicksort and in any case it only affects people who for some reason can't just increase work_mem to allow the single merge mode.
Table Size | Sort Size | 128MB | 64MB | 32MB | 16MB | 8MB | 4MB |
6914MB | 2672 MB | 3392.29 | 3102.13 | 3343.53 | 4081.23 | 4727.74 | 5620.77 |
3457MB | 1336 MB | 1669.16 | 1593.85 | 1444.22 | 1654.27 | 2076.74 | 2266.84 |
2765MB | 1069 MB | 1368.92 | 1250.44 | 1117.2 | 1293.45 | 1431.64 | 1772.18 |
1383MB | 535 MB | 716.48 | 625.06 | 557.14 | 575.67 | 644.2 | 721.68 |
691MB | 267 MB | 301.08 | 295.87 | 266.84 | 256.29 | 283.82 | 292.24 |
346MB | 134 MB | 145.48 | 149.48 | 133.23 | 130.69 | 127.67 | 137.74 |
35MB | 13 MB | 3.58 | 16.77 | 11.23 | 11.93 | 13.97 | 3.17 |
The colours are to give an idea of the number of merge passes. Grey, is an internal sort. White is a single merge. Yellow and red are successively more merges (though the exact boundary between yellow and red may not be exactly meaningful due to my misunderstanding polyphase merge).
The numbers here are seconds taken from the "elapsed" in the following log statements when running queries like the following with trace_sort enabled:
LOG: external sort ended, 342138 disk blocks used: CPU 276.04s/3173.04u sec elapsed 5620.77 sec
STATEMENT: select count(*) from (select * from n200000000 order by r offset 99999999999) AS x;
This was run on the smallest size VM on Google Compute Engine with 600MB of virtual RAM and a 100GB virtual network block device.
On Wed, Dec 2, 2015 at 10:03 AM, Robert Haas <robertmhaas@gmail.com> wrote: > > While large sorts are uncommon in queries, they are much more common > in index builds. Therefore, I think we ought to be worrying more > about regressions at 64MB than at 4MB, because we ship with > maintenance_work_mem = 64MB and a lot of people probably don't change > it before trying to build an index. You have more sympathy for people who don't tune their settings than I do. Especially now that auovacuum_work_mem exists, there is much less constraint on increasing maintance_work_mem than there is on work_mem. Unless, perhaps, you have a lot of user-driven temp tables which get indexes created on them. > If we make those index builds go > faster, users will be happy. If we make them go slower, users will be > sad. So I think it's worth asking the question "are there any CREATE > INDEX commands that someone might type on a system on which they've > done no other configuration that will be slower with this patch"? I found a regression on my 2nd attempt. I am indexing random md5 hashes (so they should get the full benefit of key abbreviation), and in this case 400,000,000 of them: create table foobar as select md5(random()::text) as x, random() as y from generate_series(1,100000000); insert into foobar select * from foobar ; insert into foobar select * from foobar ; Gives a 29GB table. with the index: create index on foobar (x); With 64MB maintenance_work_mem, I get (best time of 7 or 8): unpatched 2,436,483.834 ms allpatches 3,964,875.570 ms 62% slower not_0005 3,794,716.331 ms The unpatched sort ends with a 118-way merge followed by a 233-way merge: LOG: finished 118-way merge step: CPU 98.65s/835.67u sec elapsed 1270.61 sec LOG: performsort done (except 233-way final merge): CPU 98.75s/835.88u sec elapsed 1276.14 sec LOG: external sort ended, 2541465 disk blocks used: CPU 194.02s/1635.12u sec elapsed 2435.46 sec The patched one ends with a 2-way, two sequential 233-way merges, and a final 233-way merge: LOG: finished 2-way merge step: CPU 62.08s/435.70u sec elapsed 587.52 sec LOG: finished 233-way merge step: CPU 77.94s/660.11u sec elapsed 897.51 sec LOG: a multi-pass external merge sort is required (234 tape maximum) HINT: Consider increasing the configuration parameter "maintenance_work_mem". LOG: finished 233-way merge step: CPU 94.55s/884.63u sec elapsed 1185.17 sec LOG: performsort done (except 233-way final merge): CPU 94.76s/884.69u sec elapsed 1192.01 sec LOG: external sort ended, 2541656 disk blocks used: CPU 202.65s/1771.50u sec elapsed 3963.90 sec If you just look at the final merges of each, they should have the same number of tuples going through them (i.e. all of the tuples) but the patched one took well over twice as long, and all that time was IO time, not CPU time. I reversed out the memory pooling patch, and that shaved some time off, but nowhere near bringing it back to parity. I think what is going on here is that the different numbers of runs with the patched code just makes it land in an anti-sweat spot in the tape emulation and buffering algorithm. Each tape gets 256kB of buffer. But two tapes have one third of the tuples each other third are spread over all the other tapes almost equally (or maybe one tape has 2/3 of the tuples, if the output of one 233-way nonfinal merge was selected as the input of the other one). Once the large tape(s) has depleted its buffer, the others have had only slightly more than 1kB each depleted. Yet when it goes to fill the large tape, it also tops off every other tape while it is there, which is not going to get much read-ahead performance on them, leading to a lot of random IO. Now, I'm not sure why this same logic wouldn't apply to the unpatched code with 118-way merge too. So maybe I am all wet here. It seems like that imbalance would be enough to also cause the problem. I have seen this same type if things years ago, but was never able to analyze it to my satisfaction (as I haven't been able to do now, either). So if this patch with this exact workload just happens to land on a pre-existing infelicity, how big of a deal is that? It wouldn't be creating a regression, just shoving the region that experiences the problem around in such a way that it affects a different group of use cases. And perhaps more importantly, can anyone else reproduce this, or understand it? Cheers, Jeff
On Mon, Dec 7, 2015 at 9:01 AM, Jeff Janes <jeff.janes@gmail.com> wrote: > So if this patch with this exact workload just happens to land on a > pre-existing infelicity, how big of a deal is that? It wouldn't be > creating a regression, just shoving the region that experiences the > problem around in such a way that it affects a different group of use > cases. > > And perhaps more importantly, can anyone else reproduce this, or understand it? That's odd. I've never seen anything like that in the field myself, but then I've never really been a professional DBA. If possible, could you try using the ioreplay tool to correlate I/O with a point in the trace_sort timeline? For both master, and the patch, for comparison? The tool is available from here: https://code.google.com/p/ioapps/ There is also a tool available to graph the recorded I/O requests over time called ioprofiler. This is the only way that I've been able to graph I/O over time successfully before. Maybe there is a better way, using perf blockio or something like that, but this is the way I know to work. While I'm quite willing to believe that there are oddities about our polyphase merge implementation that can result in what you call anti-sweetspots (sourspots?), I have a much harder time imagining why reverting my merge patch could make things better, unless the system was experiencing some kind of memory pressure. I mean, it doesn't change the algorithm at all, except to make more memory available from the merge by avoiding palloc() fragmentation. How could that possibly hurt? -- Peter Geoghegan
On Mon, Dec 7, 2015 at 9:01 AM, Jeff Janes <jeff.janes@gmail.com> wrote: > > The patched one ends with a 2-way, two sequential 233-way merges, and > a final 233-way merge: > > LOG: finished 2-way merge step: CPU 62.08s/435.70u sec elapsed 587.52 sec > LOG: finished 233-way merge step: CPU 77.94s/660.11u sec elapsed 897.51 sec > LOG: a multi-pass external merge sort is required (234 tape maximum) > HINT: Consider increasing the configuration parameter "maintenance_work_mem". > LOG: finished 233-way merge step: CPU 94.55s/884.63u sec elapsed 1185.17 sec > LOG: performsort done (except 233-way final merge): CPU > 94.76s/884.69u sec elapsed 1192.01 sec > LOG: external sort ended, 2541656 disk blocks used: CPU > 202.65s/1771.50u sec elapsed 3963.90 sec > > > If you just look at the final merges of each, they should have the > same number of tuples going through them (i.e. all of the tuples) but > the patched one took well over twice as long, and all that time was IO > time, not CPU time. > > I reversed out the memory pooling patch, and that shaved some time > off, but nowhere near bringing it back to parity. > > I think what is going on here is that the different numbers of runs > with the patched code just makes it land in an anti-sweat spot in the > tape emulation and buffering algorithm. > > Each tape gets 256kB of buffer. But two tapes have one third of the > tuples each other third are spread over all the other tapes almost > equally (or maybe one tape has 2/3 of the tuples, if the output of one > 233-way nonfinal merge was selected as the input of the other one). > Once the large tape(s) has depleted its buffer, the others have had > only slightly more than 1kB each depleted. Yet when it goes to fill > the large tape, it also tops off every other tape while it is there, > which is not going to get much read-ahead performance on them, leading > to a lot of random IO. The final merge only refills each tape buffer as that buffer gets depleted, rather than refilling all of them whenever any is depleted, so my explanation doesn't work. But move it back one layer. There are 3 sequential 233-way merges. The first one produces a giant tape run. The second one consumes that giant tape run along with 232 small tape runs. At this point, the logic I describe above does come into play, refilling each of the buffers for the small runs much too often, freeing blocks on the tape emulation for those runs in dribs and drabs. Those free blocks get re-used by the giant output tape run, in a scattered fashion. Then in the next (final) merge, it is has to read in this huge fragmented tape run emulation, generating a lot of random IO to read it. With the patched code, the average length of reads on files in pgsql_tmp between lseeks or changing to a different file descriptor is 8, while in the unpatched code it is 14. > > Now, I'm not sure why this same logic wouldn't apply to the unpatched > code with 118-way merge too. So maybe I am all wet here. It seems > like that imbalance would be enough to also cause the problem. So my current theory is that it takes one large merge to generate an unbalanced tape, one large merge where that large unbalanced tape leads to fragmenting the output tape, and one final merge to be slowed down by this fragmentation. I looked at https://code.google.com/p/ioapps/ as Peter recommended, but couldn't figure out what do with it. The only conclusion I got from ioprofiler was that it spend a lot of time reading files in pgsql_tmp. I found just doing strace -y -ttt -T -p <pid> And then analyzing with perl one liners to work better, but it could just be the learning curve.
On Wed, Dec 9, 2015 at 12:02 AM, Jeff Janes <jeff.janes@gmail.com> wrote: > > > Then in the next (final) merge, it is has to read in this huge > fragmented tape run emulation, generating a lot of random IO to read > it. This seems fairly plausible. Logtape.c is basically implementing a small filesystem and doesn't really make any attempt to avoid fragmentation. The reason it does this is so that we can reuse blocks and avoid needing to store 2x disk space for the temporary space. I wonder if we're no longer concerned about keeping the number of tapes down if it makes sense to give up on this goal too and just write out separate files for each tape letting the filesystem avoid fragmentation. I suspect it would also be better for filesystems like ZFS and SSDs where rewriting blocks can be expensive. > With the patched code, the average length of reads on files in > pgsql_tmp between lseeks or changing to a different file descriptor is > 8, while in the unpatched code it is 14. I don't think Peter did anything to the scheduling of the merges so I don't see how this would be different. It might just have hit a preexisting case by changing the number and size of tapes. I also don't think the tapes really ought to be so unbalanced. I've noticed some odd things myself -- like what does a 1-way merge mean here? LOG: finished writing run 56 to tape 2 (9101313 blocks): CPU 0.19s/10.97u sec elapsed 16.68 sec LOG: finished writing run 57 to tape 3 (9084929 blocks): CPU 0.19s/11.14u sec elapsed 19.08 sec LOG: finished writing run 58 to tape 4 (9101313 blocks): CPU 0.20s/11.31u sec elapsed 19.26 sec LOG: performsort starting: CPU 0.20s/11.48u sec elapsed 19.44 sec LOG: finished writing run 59 to tape 5 (9109505 blocks): CPU 0.20s/11.49u sec elapsed 19.44 sec LOG: finished writing final run 60 to tape 6 (8151041 blocks): CPU 0.20s/11.55u sec elapsed 19.50 sec LOG: finished 1-way merge step (1810433 blocks): CPU 0.20s/11.58u sec elapsed 19.54 sec <-------------------------========= LOG: finished 10-way merge step (19742721 blocks): CPU 0.20s/12.23u sec elapsed 20.19 sec LOG: finished 13-way merge step (23666689 blocks): CPU 0.20s/13.15u sec elapsed 21.11 sec LOG: finished 13-way merge step (47333377 blocks): CPU 0.22s/14.07u sec elapsed 23.13 sec LOG: finished 14-way merge step (47333377 blocks): CPU 0.24s/15.65u sec elapsed 24.74 sec LOG: performsort done (except 14-way final merge): CPU 0.24s/15.66u sec elapsed 24.75 sec I wonder if something's wrong with the merge scheduling. Fwiw attached are two patches for perusal. One is a trivial patch to add the size of the tape to trace_sort output. I guess I'll just apply that without discussion. The other replaces the selection sort with an open coded sort network for cases up to 8 elements. (Only in the perl generated qsort for the moment). I don't have the bandwidth to benchmark this for the moment but if anyone's interested in trying I suspect it'll make a small but noticeable difference. I'm guessing 2-5%. -- greg
Attachment
On Tue, Dec 8, 2015 at 6:39 PM, Greg Stark <stark@mit.edu> wrote: > Fwiw attached are two patches for perusal. One is a trivial patch to > add the size of the tape to trace_sort output. I guess I'll just apply > that without discussion. +1 > The other replaces the selection sort with an > open coded sort network for cases up to 8 elements. (Only in the perl > generated qsort for the moment). I don't have the bandwidth to > benchmark this for the moment but if anyone's interested in trying I > suspect it'll make a small but noticeable difference. I'm guessing > 2-5%. I guess you mean insertion sort. What's the theoretical justification for the change? -- Peter Geoghegan
On Tue, Dec 8, 2015 at 6:44 PM, Peter Geoghegan <pg@heroku.com> wrote: > On Tue, Dec 8, 2015 at 6:39 PM, Greg Stark <stark@mit.edu> wrote: >> Fwiw attached are two patches for perusal. One is a trivial patch to >> add the size of the tape to trace_sort output. I guess I'll just apply >> that without discussion. > > +1 >> +/* >> + * Obtain total disk space currently used by a LogicalTapeSet, in blocks. >> + */ >> +long >> +LogicalTapeBlocks(LogicalTapeSet *lts, int tapenum) >> +{ >> + return lts->tapes[tapenum].numFullBlocks * BLCKSZ + 1; >> +} Why multiply by BLCKSZ here? -- Peter Geoghegan
<p dir="ltr"><br /> On 9 Dec 2015 02:44, "Peter Geoghegan" <<a href="mailto:pg@heroku.com">pg@heroku.com</a>> wrote:<br/> ><br /> > I guess you mean insertion sort. What's the theoretical justification<br /> > for the change?<pdir="ltr">Er, right. Insertion sort.<p dir="ltr">The sort networks I used here are optimal both in number of comparisonsand depth. I suspect modern CPUs actually manage to do some of the comparisons in parallel even. <p dir="ltr">Iwas experimenting with using SIMD registers and did a non SIMD implementation like this first and noticed it wasdoing 15% fewer comparisons than insertion sort and ran faster. That was for sets of 8, I'm not sure there's as much savingon smaller sets. <br />
On Tue, Dec 8, 2015 at 7:09 PM, Peter Geoghegan <pg@heroku.com> wrote: > Why multiply by BLCKSZ here? I ask because LogicalTapeSetBlocks() returns blocks directly, not tapes, and I'd expect the same. Also, the callers seem to expect blocks, not bytes. -- Peter Geoghegan
On 09/12/15 00:02, Jeff Janes wrote: > The second one consumes that giant tape run along with 232 small tape > runs. In terms of number of comparisons, binary merge works best when the inputs are of similar length. I'd assume the same goes for n-ary merge, but I don't know if comparison count is an issue here. -- Cheers, Jeremy
On Wed, Dec 9, 2015 at 2:44 AM, Peter Geoghegan <pg@heroku.com> wrote: > On Tue, Dec 8, 2015 at 6:39 PM, Greg Stark <stark@mit.edu> wrote: > > I guess you mean insertion sort. What's the theoretical justification > for the change? Well my thinking was that hard coding a series of comparisons would be faster than a loop doing a O(n^2) algorithm even for small constants. And sort networks are perfect for hard coded sorts because they do the same comparisons regardless of the results of previous comparisons so there are no branches. And even better the comparisons are as much as possible independent of each other -- sort networks are typically measured by the depth which assumes any comparisons between disjoint pairs can be done in parallel. Even if it's implemented in serial the processor is probably parallelizing some of the work. So I implemented a quick benchmark outside Postgres based on sorting actual SortTuples with datum1 defined to be random 64-bit integers (no nulls). Indeed the sort networks perform faster on average despite doing more comparisons. That makes me think the cpu is indeed doing some of the work in parallel. However the number of comparisons is significantly higher. And in the non-"abbreviated keys" case where the compare is going to be a function pointer call the number of comparisons is probably more important than the actual time spent when benchmarking comparing int64s. In that case insertion sort does seem to be better than using the sort networks. Interestingly it looks like we could raise the threshold to switching to insertion sort. At least on my machine the insertion sort is faster in real time as well as fewer comparisons up to 9 elements. It's actually faster up to 16 elements despite doing more comparisons than quicksort. Note also how our quicksort does more comparisons than the libc quicksort (which is actually merge sort in glibc I hear) which is probably due to the "presorted" check. $ for i in `seq 2 32` ; do echo ; echo $i ; ./a.out $i ; done 2 using bitonic sort 32.781ns per sort of 2 24-byte items 1.0 compares/sort 0.5 swaps/sort using insertion sort 29.805ns per sort of 2 24-byte items 1.0 compares/sort 0.5 swaps/sort using sort networks sort 26.392ns per sort of 2 24-byte items 1.0 compares/sort 0.5 swaps/sort using libc quicksort sort 54.250ns per sort of 2 24-byte items 1.0 compares/sort using qsort_ssup sort 46.666ns per sort of 2 24-byte items 1.0 compares/sort 3 using insertion sort 42.090ns per sort of 3 24-byte items 2.7 compares/sort 1.5 swaps/sort using sort networks sort 38.442ns per sort of 3 24-byte items 3.0 compares/sort 1.5 swaps/sort using libc quicksort sort 86.759ns per sort of 3 24-byte items 2.7 compares/sort using qsort_ssup sort 41.238ns per sort of 3 24-byte items 2.7 compares/sort 4 using bitonic sort 73.420ns per sort of 4 24-byte items 6.0 compares/sort 3.0 swaps/sort using insertion sort 61.087ns per sort of 4 24-byte items 4.9 compares/sort 3.0 swaps/sort using sort networks sort 58.930ns per sort of 4 24-byte items 5.0 compares/sort 2.7 swaps/sort using libc quicksort sort 135.930ns per sort of 4 24-byte items 4.7 compares/sort using qsort_ssup sort 59.669ns per sort of 4 24-byte items 4.9 compares/sort 5 using insertion sort 88.345ns per sort of 5 24-byte items 7.7 compares/sort 5.0 swaps/sort using sort networks sort 90.034ns per sort of 5 24-byte items 9.0 compares/sort 4.4 swaps/sort using libc quicksort sort 180.367ns per sort of 5 24-byte items 7.2 compares/sort using qsort_ssup sort 85.603ns per sort of 5 24-byte items 7.7 compares/sort 6 using insertion sort 119.697ns per sort of 6 24-byte items 11.0 compares/sort 7.5 swaps/sort using sort networks sort 122.071ns per sort of 6 24-byte items 12.0 compares/sort 5.4 swaps/sort using libc quicksort sort 234.436ns per sort of 6 24-byte items 9.8 compares/sort using qsort_ssup sort 115.407ns per sort of 6 24-byte items 11.0 compares/sort 7 using insertion sort 152.639ns per sort of 7 24-byte items 14.9 compares/sort 10.5 swaps/sort using sort networks sort 155.357ns per sort of 7 24-byte items 16.0 compares/sort 7.3 swaps/sort using libc quicksort sort 303.738ns per sort of 7 24-byte items 12.7 compares/sort using qsort_ssup sort 166.174ns per sort of 7 24-byte items 16.0 compares/sort 8 using bitonic sort 248.527ns per sort of 8 24-byte items 24.0 compares/sort 12.0 swaps/sort using insertion sort 193.057ns per sort of 8 24-byte items 19.3 compares/sort 14.0 swaps/sort using sort networks sort 230.738ns per sort of 8 24-byte items 24.0 compares/sort 12.0 swaps/sort using libc quicksort sort 360.852ns per sort of 8 24-byte items 15.7 compares/sort using qsort_ssup sort 211.729ns per sort of 8 24-byte items 20.6 compares/sort 9 using insertion sort 222.475ns per sort of 9 24-byte items 24.2 compares/sort 18.0 swaps/sort using libc quicksort sort 427.760ns per sort of 9 24-byte items 19.2 compares/sort using qsort_ssup sort 249.668ns per sort of 9 24-byte items 24.6 compares/sort 10 using insertion sort 277.386ns per sort of 10 24-byte items 29.6 compares/sort 22.5 swaps/sort using libc quicksort sort 482.730ns per sort of 10 24-byte items 22.7 compares/sort using qsort_ssup sort 294.956ns per sort of 10 24-byte items 29.0 compares/sort 11 using insertion sort 312.613ns per sort of 11 24-byte items 35.5 compares/sort 27.5 swaps/sort using libc quicksort sort 583.617ns per sort of 11 24-byte items 26.3 compares/sort using qsort_ssup sort 353.054ns per sort of 11 24-byte items 33.5 compares/sort 12 using insertion sort 381.011ns per sort of 12 24-byte items 41.9 compares/sort 33.0 swaps/sort using libc quicksort sort 640.265ns per sort of 12 24-byte items 30.0 compares/sort using qsort_ssup sort 396.703ns per sort of 12 24-byte items 38.2 compares/sort 13 using insertion sort 407.784ns per sort of 13 24-byte items 48.8 compares/sort 39.0 swaps/sort using libc quicksort sort 716.017ns per sort of 13 24-byte items 33.8 compares/sort using qsort_ssup sort 443.356ns per sort of 13 24-byte items 43.1 compares/sort 14 using insertion sort 461.696ns per sort of 14 24-byte items 56.3 compares/sort 45.5 swaps/sort using libc quicksort sort 782.418ns per sort of 14 24-byte items 37.7 compares/sort using qsort_ssup sort 492.749ns per sort of 14 24-byte items 48.1 compares/sort 15 using insertion sort 528.879ns per sort of 15 24-byte items 64.1 compares/sort 52.5 swaps/sort using libc quicksort sort 868.679ns per sort of 15 24-byte items 41.7 compares/sort using qsort_ssup sort 537.568ns per sort of 15 24-byte items 53.3 compares/sort 16 using bitonic sort 835.212ns per sort of 16 24-byte items 80.0 compares/sort 40.0 swaps/sort using insertion sort 575.019ns per sort of 16 24-byte items 72.6 compares/sort 60.0 swaps/sort using libc quicksort sort 944.284ns per sort of 16 24-byte items 45.7 compares/sort using qsort_ssup sort 591.027ns per sort of 16 24-byte items 58.5 compares/sort -- greg
On Fri, Dec 11, 2015 at 10:41 PM, Greg Stark <stark@mit.edu> wrote: > > Interestingly it looks like we could raise the threshold to switching > to insertion sort. At least on my machine the insertion sort is faster > in real time as well as fewer comparisons up to 9 elements. It's > actually faster up to 16 elements despite doing more comparisons than > quicksort. > > Note also how our quicksort does more comparisons than the libc > quicksort (which is actually merge sort in glibc I hear) which is > probably due to the "presorted" check. Heh. And if I comment out the presorted check the breakeven point is *exactly* where the threshold is today at 7 elements -- presumably because Hoare chose it on purpose. 7 using insertion sort 145.517ns per sort of 7 24-byte items 14.9 compares/sort 10.5 swaps/sort using sort networks sort 146.764ns per sort of 7 24-byte items 16.0 compares/sort 7.3 swaps/sort using libc quicksort sort 282.659ns per sort of 7 24-byte items 12.7 compares/sort using qsort_ssup sort 141.817ns per sort of 7 24-byte items 14.3 compares/sort -- greg
On Fri, Dec 11, 2015 at 2:52 PM, Greg Stark <stark@mit.edu> wrote: > Heh. And if I comment out the presorted check the breakeven point is > *exactly* where the threshold is today at 7 elements -- presumably > because Hoare chose it on purpose. I think it was Sedgewick, but yes. I'd be very hesitant to mess with the number of elements that we fallback to insertion sort on. I've heard of people removing that optimization on the theory that it no longer applies, but I think they were wrong to. -- Peter Geoghegan
On Fri, Dec 11, 2015 at 2:41 PM, Greg Stark <stark@mit.edu> wrote: > However the number of comparisons is significantly higher. And in the > non-"abbreviated keys" case where the compare is going to be a > function pointer call the number of comparisons is probably more > important than the actual time spent when benchmarking comparing > int64s. In that case insertion sort does seem to be better than using > the sort networks. Back when I wrote a prototype of Timsort, pre-abbreviated keys, it required significantly fewer text comparisons [1] in fair and representative cases (i.e. not particularly tickling our quicksort's precheck thing), and yet was significantly slower. [1] http://www.postgresql.org/message-id/CAEYLb_W++UhrcWprzG9TyBVF7Sn-c1s9oLbABvAvPGdeP2DFSQ@mail.gmail.com -- Peter Geoghegan
On Sun, Dec 6, 2015 at 4:25 PM, Peter Geoghegan <pg@heroku.com> wrote: > Maybe we should consider trying to get patch 0002 (the memory > pool/merge patch) committed first, something Greg Stark suggested > privately. That might actually be an easier way of integrating this > work, since it changes nothing about the algorithm we use for merging > (it only improves memory locality), and so is really an independent > piece of work (albeit one that makes a huge overall difference due to > the other patches increasing the time spent merging in absolute terms, > and especially as a proportion of the total). I have a question about the terminology used in this patch. What is a tuple proper? What is it in contradistinction to? I would think that a tuple which is located in its own palloc'ed space is the "proper" one, leaving a tuple allocated in the bulk memory pool to be called...something else. I don't know what the non-judgmental-sounding antonym of postpositive "proper" is. Also, if I am reading this correctly, when we refill a pool from a logical tape we still transform each tuple as it is read from the disk format to the memory format. This inflates the size quite a bit, at least for single-datum tuples. If we instead just read the disk format directly into the pool, and converted them into the in-memory format when each tuple came due for the merge heap, would that destroy the locality of reference you are seeking to gain? Cheers, Jeff
On Sat, Dec 12, 2015 at 12:41 AM, Greg Stark <stark@mit.edu> wrote: > On Wed, Dec 9, 2015 at 2:44 AM, Peter Geoghegan <pg@heroku.com> wrote: >> On Tue, Dec 8, 2015 at 6:39 PM, Greg Stark <stark@mit.edu> wrote: >> >> I guess you mean insertion sort. What's the theoretical justification >> for the change? > > Well my thinking was that hard coding a series of comparisons would be > faster than a loop doing a O(n^2) algorithm even for small constants. > And sort networks are perfect for hard coded sorts because they do the > same comparisons regardless of the results of previous comparisons so > there are no branches. And even better the comparisons are as much as > possible independent of each other -- sort networks are typically > measured by the depth which assumes any comparisons between disjoint > pairs can be done in parallel. Even if it's implemented in serial the > processor is probably parallelizing some of the work. > > So I implemented a quick benchmark outside Postgres based on sorting > actual SortTuples with datum1 defined to be random 64-bit integers (no > nulls). Indeed the sort networks perform faster on average despite > doing more comparisons. That makes me think the cpu is indeed doing > some of the work in parallel. The open coded version you shared bloats the code by 37kB, I'm not sure it is pulling it's weight, especially given relatively heavy comparators. A quick index creation test on int4's profiled with perf shows about 3% of CPU being spent in the code being replaced. Any improvement on that is going to be too small to easily quantify. As the open coding doesn't help with eliminating control flow dependencies, so my idea is to encode the sort network comparison order in an array and use that to drive a simple loop. The code size would be pretty similar to insertion sort and the loop overhead should mostly be hidden by the CPU OoO machinery. Probably won't help much, but would be interesting and simple enough to try out. Can you share you code for the benchmark so I can try it out? Regards, Ants Aasma
On Sat, Dec 12, 2015 at 7:42 PM, Ants Aasma <ants.aasma@eesti.ee> wrote: > As the open coding doesn't help with eliminating control flow > dependencies, so my idea is to encode the sort network comparison > order in an array and use that to drive a simple loop. The code size > would be pretty similar to insertion sort and the loop overhead should > mostly be hidden by the CPU OoO machinery. Probably won't help much, > but would be interesting and simple enough to try out. Can you share > you code for the benchmark so I can try it out? I can. But the further results showing the number of comparisons is higher than for insertion sort have dampened my enthusiasm for the change. I'm assuming that even if it's faster for a simple integer or sort it'll be much slower for anything that requires calling out to the datatype comparator. I also hadn't actually measured what percentage of the sort was being spent in the insertion sort. I had guessed it would be higher. The test is attached. qsort_tuple.c is copied from tuplesort (with the ifdef for NOPRESORT added, but you could skip that if you want.). Compile with something like: gcc -DNOPRESORT -O3 -DCOUNTS -Wall -Wno-unused-function simd-sort-test.c -- greg
Attachment
On Sat, Dec 12, 2015 at 12:10 AM, Jeff Janes <jeff.janes@gmail.com> wrote: > I have a question about the terminology used in this patch. What is a > tuple proper? What is it in contradistinction to? I would think that > a tuple which is located in its own palloc'ed space is the "proper" > one, leaving a tuple allocated in the bulk memory pool to be > called...something else. I don't know what the > non-judgmental-sounding antonym of postpositive "proper" is. "Tuple proper" is a term that appears 5 times in tuplesort.c today. As it says at the top of that file: /** The objects we actually sort are SortTuple structs. These contain* a pointer to the tuple proper (might be a MinimalTupleor IndexTuple),* which is a separate palloc chunk --- we assume it is just one chunk and* can be freed by a simplepfree(). SortTuples also contain the tuple's* first key column in Datum/nullflag format, and an index integer. > Also, if I am reading this correctly, when we refill a pool from a > logical tape we still transform each tuple as it is read from the disk > format to the memory format. This inflates the size quite a bit, at > least for single-datum tuples. If we instead just read the disk > format directly into the pool, and converted them into the in-memory > format when each tuple came due for the merge heap, would that destroy > the locality of reference you are seeking to gain? Are you talking about alignment? -- Peter Geoghegan
On Sat, Dec 12, 2015 at 2:28 PM, Peter Geoghegan <pg@heroku.com> wrote: > On Sat, Dec 12, 2015 at 12:10 AM, Jeff Janes <jeff.janes@gmail.com> wrote: >> I have a question about the terminology used in this patch. What is a >> tuple proper? What is it in contradistinction to? I would think that >> a tuple which is located in its own palloc'ed space is the "proper" >> one, leaving a tuple allocated in the bulk memory pool to be >> called...something else. I don't know what the >> non-judgmental-sounding antonym of postpositive "proper" is. > > "Tuple proper" is a term that appears 5 times in tuplesort.c today. As > it says at the top of that file: > > /* > * The objects we actually sort are SortTuple structs. These contain > * a pointer to the tuple proper (might be a MinimalTuple or IndexTuple), > * which is a separate palloc chunk --- we assume it is just one chunk and > * can be freed by a simple pfree(). SortTuples also contain the tuple's > * first key column in Datum/nullflag format, and an index integer. Those usages make sense to me, as they are locally self-contained and it is clear what they are in contradistinction to. But your usage is spread throughout (even in function names, not just comments) and seems to contradict the current usage as yours are not separately palloced, as the "proper" ones described here are. I think that "proper" only works when the same comment also defines the alternative, rather than as some file-global description. Maybe "pooltuple" rather than "tupleproper" > >> Also, if I am reading this correctly, when we refill a pool from a >> logical tape we still transform each tuple as it is read from the disk >> format to the memory format. This inflates the size quite a bit, at >> least for single-datum tuples. If we instead just read the disk >> format directly into the pool, and converted them into the in-memory >> format when each tuple came due for the merge heap, would that destroy >> the locality of reference you are seeking to gain? > > Are you talking about alignment? Maybe alignment, but also the size of the SortTuple struct itself, which is not present on tape but is present in memory if I understand correctly. When reading 128kb (32 blocks) worth of in-memory pool, it seems like it only gets to read 16 to 18 blocks of tape to fill them up, in the case of building an index on single column 32-byte random md5 digests. I don't exactly know where all of that space goes, I'm taking an experimentalist approach. Cheers, Jeff
On Tue, Dec 8, 2015 at 6:39 PM, Greg Stark <stark@mit.edu> wrote: > On Wed, Dec 9, 2015 at 12:02 AM, Jeff Janes <jeff.janes@gmail.com> wrote: >> >> >> Then in the next (final) merge, it is has to read in this huge >> fragmented tape run emulation, generating a lot of random IO to read >> it. > > This seems fairly plausible. Logtape.c is basically implementing a > small filesystem and doesn't really make any attempt to avoid > fragmentation. The reason it does this is so that we can reuse blocks > and avoid needing to store 2x disk space for the temporary space. I > wonder if we're no longer concerned about keeping the number of tapes > down if it makes sense to give up on this goal too and just write out > separate files for each tape letting the filesystem avoid > fragmentation. I suspect it would also be better for filesystems like > ZFS and SSDs where rewriting blocks can be expensive. During my testing I actually ran into space problems, where the index I was building and the temp files used to do the sort for it could not coexist, and I was wondering if there wasn't a way to free up some of those temp files as the index was growing. So I don't think we want to throw caution to the wind here. (Also, I think it does make *some* attempt to reduce fragmentation, but it could probably do more.) > > >> With the patched code, the average length of reads on files in >> pgsql_tmp between lseeks or changing to a different file descriptor is >> 8, while in the unpatched code it is 14. > > I don't think Peter did anything to the scheduling of the merges so I > don't see how this would be different. It might just have hit a > preexisting case by changing the number and size of tapes. Correct. (There was a small additional increase with the memory pool, but it was small enough that I am not worried about it). But, this changing number and size of tapes was exactly what Robert was worried about, so I don't want to just dismiss it without further investigation. > > I also don't think the tapes really ought to be so unbalanced. I've > noticed some odd things myself -- like what does a 1-way merge mean > here? I noticed some of those (although in my case they were always the first merges which were one-way) and I just attributed it to the fact that the algorithm doesn't know how many runs there will be up front, and so can't optimally distribute them among the tapes. But it does occur to me that we are taking the tape analogy rather too far in that case. We could say that we have only 223 tape *drives*, but that each run is a separate tape which can be remounted amongst the drives in any combination, as long as only 223 are active at one time. I started looking into this at one time, before I got sidetracked on the fact that the memory usage pattern would often leave a few bytes less than half of work_mem completely unused. Once that memory usage got fixed, I never returned to the original examination. And it would be a shame to sink more time into it now, when we are trying to avoid these polyphase merges altogether. So, is a sometimes-regression at 64MB really a blocker to substantial improvement most of the time at 64MB, and even more so at more realistic modern settings for large index building? > Fwiw attached are two patches for perusal. One is a trivial patch to > add the size of the tape to trace_sort output. I guess I'll just apply > that without discussion. +1 there. Having this in place would make evaluating the other things be easier. Cheers, Jeff On Tue, Dec 8, 2015 at 6:39 PM, Greg Stark <stark@mit.edu> wrote: > On Wed, Dec 9, 2015 at 12:02 AM, Jeff Janes <jeff.janes@gmail.com> wrote: >> >> >> Then in the next (final) merge, it is has to read in this huge >> fragmented tape run emulation, generating a lot of random IO to read >> it. > > This seems fairly plausible. Logtape.c is basically implementing a > small filesystem and doesn't really make any attempt to avoid > fragmentation. The reason it does this is so that we can reuse blocks > and avoid needing to store 2x disk space for the temporary space. I > wonder if we're no longer concerned about keeping the number of tapes > down if it makes sense to give up on this goal too and just write out > separate files for each tape letting the filesystem avoid > fragmentation. I suspect it would also be better for filesystems like > ZFS and SSDs where rewriting blocks can be expensive. > > >> With the patched code, the average length of reads on files in >> pgsql_tmp between lseeks or changing to a different file descriptor is >> 8, while in the unpatched code it is 14. > > I don't think Peter did anything to the scheduling of the merges so I > don't see how this would be different. It might just have hit a > preexisting case by changing the number and size of tapes. > > I also don't think the tapes really ought to be so unbalanced. I've > noticed some odd things myself -- like what does a 1-way merge mean > here? > > LOG: finished writing run 56 to tape 2 (9101313 blocks): CPU > 0.19s/10.97u sec elapsed 16.68 sec > LOG: finished writing run 57 to tape 3 (9084929 blocks): CPU > 0.19s/11.14u sec elapsed 19.08 sec > LOG: finished writing run 58 to tape 4 (9101313 blocks): CPU > 0.20s/11.31u sec elapsed 19.26 sec > LOG: performsort starting: CPU 0.20s/11.48u sec elapsed 19.44 sec > LOG: finished writing run 59 to tape 5 (9109505 blocks): CPU > 0.20s/11.49u sec elapsed 19.44 sec > LOG: finished writing final run 60 to tape 6 (8151041 blocks): CPU > 0.20s/11.55u sec elapsed 19.50 sec > LOG: finished 1-way merge step (1810433 blocks): CPU 0.20s/11.58u sec > elapsed 19.54 sec <-------------------------========= > LOG: finished 10-way merge step (19742721 blocks): CPU 0.20s/12.23u > sec elapsed 20.19 sec > LOG: finished 13-way merge step (23666689 blocks): CPU 0.20s/13.15u > sec elapsed 21.11 sec > LOG: finished 13-way merge step (47333377 blocks): CPU 0.22s/14.07u > sec elapsed 23.13 sec > LOG: finished 14-way merge step (47333377 blocks): CPU 0.24s/15.65u > sec elapsed 24.74 sec > LOG: performsort done (except 14-way final merge): CPU 0.24s/15.66u > sec elapsed 24.75 sec > > I wonder if something's wrong with the merge scheduling. > > Fwiw attached are two patches for perusal. One is a trivial patch to > add the size of the tape to trace_sort output. I guess I'll just apply > that without discussion. The other replaces the selection sort with an > open coded sort network for cases up to 8 elements. (Only in the perl > generated qsort for the moment). I don't have the bandwidth to > benchmark this for the moment but if anyone's interested in trying I > suspect it'll make a small but noticeable difference. I'm guessing > 2-5%. > > -- > greg
On Sat, Dec 12, 2015 at 4:41 PM, Jeff Janes <jeff.janes@gmail.com> wrote: > Those usages make sense to me, as they are locally self-contained and > it is clear what they are in contradistinction to. But your usage is > spread throughout (even in function names, not just comments) and > seems to contradict the current usage as yours are not separately > palloced, as the "proper" ones described here are. I think that > "proper" only works when the same comment also defines the > alternative, rather than as some file-global description. Maybe > "pooltuple" rather than "tupleproper" I don't think of it that way. The "tuple proper" is the thing that the client passes to their tuplesort -- the thing they are actually interested in having sorted. Like an IndexTuple for CREATE INDEX callers, for example. SortTuple is just an internal implementation detail. (That appears all over the file tuplesort.c, just as my new references to "tuple proper" do. But neither appear elsewhere.) >>> Also, if I am reading this correctly, when we refill a pool from a >>> logical tape we still transform each tuple as it is read from the disk >>> format to the memory format. This inflates the size quite a bit, at >>> least for single-datum tuples. If we instead just read the disk >>> format directly into the pool, and converted them into the in-memory >>> format when each tuple came due for the merge heap, would that destroy >>> the locality of reference you are seeking to gain? >> >> Are you talking about alignment? > > Maybe alignment, but also the size of the SortTuple struct itself, > which is not present on tape but is present in memory if I understand > correctly. > > When reading 128kb (32 blocks) worth of in-memory pool, it seems like > it only gets to read 16 to 18 blocks of tape to fill them up, in the > case of building an index on single column 32-byte random md5 digests. > I don't exactly know where all of that space goes, I'm taking an > experimentalist approach. I'm confused. readtup_datum(), just like every other READTUP() variant, has the new function tupproperalloc() as a drop-in replacement for the master branch palloc() + USEMEM() calls. It is true that tupproperalloc() (and a couple of other places relating to preloading) know *a little* about the usage pattern -- tupproperalloc() accepts a "tape number" argument to know what partition within the large pool/buffer to use for each logical allocation. However, from the point of view of correctness, tupproperalloc() should function as a drop-in replacement for palloc() + USEMEM() calls in the context of the various READTUP() routines. I have done nothing special with any particular READTUP() routine, including readtup_datum() (all READTUP() routines have received the same treatment). Nothing else was changed in those routines, including how tuples are stored on tape. The datum case does kind of store the SortTuples on tape today in one very limited sense, which is that the length is stored fairly naively (that's already available from the IndexTuple in the case of writetup_index(), for example, but length must be stored explicitly for the datum case). My guess is you're confusion comes from the fact that the memtuples array (the array of SortTuple) is also factored in to memory accounting, but that grows at geometric intervals, whereas the existing READTUP() retail palloc() calls (and their USEMEM() memory accounting calls) occur in drips and drabs. It's probably the case that the sizing of the memtuples array and the amount of memory we use for that rather than retail palloc()/"tuple proper" memory is a kind of arbitrary (why should the needs be the same when SortTuples are merge step "slots"?), but I don't think that's the biggest problem in this general area at all. -- Peter Geoghegan
On Sun, Dec 13, 2015 at 3:40 PM, Peter Geoghegan <pg@heroku.com> wrote: > On Sat, Dec 12, 2015 at 4:41 PM, Jeff Janes <jeff.janes@gmail.com> wrote: > >>>> Also, if I am reading this correctly, when we refill a pool from a >>>> logical tape we still transform each tuple as it is read from the disk >>>> format to the memory format. This inflates the size quite a bit, at >>>> least for single-datum tuples. If we instead just read the disk >>>> format directly into the pool, and converted them into the in-memory >>>> format when each tuple came due for the merge heap, would that destroy >>>> the locality of reference you are seeking to gain? >>> >>> Are you talking about alignment? >> >> Maybe alignment, but also the size of the SortTuple struct itself, >> which is not present on tape but is present in memory if I understand >> correctly. >> >> When reading 128kb (32 blocks) worth of in-memory pool, it seems like >> it only gets to read 16 to 18 blocks of tape to fill them up, in the >> case of building an index on single column 32-byte random md5 digests. >> I don't exactly know where all of that space goes, I'm taking an >> experimentalist approach. > > I'm confused. > > readtup_datum(), just like every other READTUP() variant, has the new > function tupproperalloc() as a drop-in replacement for the master > branch palloc() + USEMEM() calls. Right, I'm not comparing what your patch does to what the existing code does. I'm comparing it to what it could be doing. Only call READTUP when you need to go from the pool to the heap, not when you need to go from tape to the pool. If you store the data in the pool the same way they are stored on tape, then we no longer need memtuples at all. There is already a "mergetupcur" per tape pointing to the first tuple of the tape, and since they are now stored contiguously that is all that is needed, once you are done with one tuple the pointer is left pointing at the next one. The reason for memtuples is to handle random access. Since we are no longer doing random access, we no longer need it. We could free memtuples, re-allocate just enough to form the binary heap for the N-way merge, and use all the rest of that space (which could be a significant fraction of work_mem) as part of the new pool. Cheers, Jeff
On Sun, Dec 13, 2015 at 7:31 PM, Jeff Janes <jeff.janes@gmail.com> wrote: > The reason for memtuples is to handle random access. Since we are no > longer doing random access, we no longer need it. > > We could free memtuples, re-allocate just enough to form the binary > heap for the N-way merge, and use all the rest of that space (which > could be a significant fraction of work_mem) as part of the new pool. Oh, you're talking about having the final on-the-fly merge use a tuplestore-style array of pointers to "tuple proper" memory (this was how tuplesort.c worked in all cases about 15 years ago, actually). I thought about that. It's not obvious how we'd do without SortTuple.tupindex during the merge phase, since it sometimes represents an offset into memtuples (the SortTuple array). See "free list" management within mergeonerun(). -- Peter Geoghegan
I ran sorts with various parameters on my small NAS server. This is a fairly slow CPU and limited memory machine with lots of disk so I thought it would actually make a good test case for smaller servers. The following is the speedup (for values < 100%) or slowdown (values > 100%) for the first patch only, the "quicksort all runs" without the extra memory optimizations.
At first glance it's a clear pattern that the extra runs does cause a slowdown whenever it causes more polyphase merges which is bad news. But on further inspection look just how low work_mem had to be to have a significant effect. Only the 4MB and 8MB work_mem cases were significantly impacted and only when sorting over a GB of data (which was 2.7 - 7GB with the tuple overhead). The savings when work_mem was 64MB or 128MB was substantial.
Table Size | Sort Size | 128MB | 64MB | 32MB | 16MB | 8MB | 4MB |
6914MB | 2672 MB | 64% | 70% | 93% | 110% | 133% | 137% |
3457MB | 1336 MB | 64% | 67% | 90% | 92% | 137% | 120% |
2765MB | 1069 MB | 68% | 66% | 84% | 95% | 111% | 137% |
1383MB | 535 MB | 66% | 70% | 72% | 92% | 99% | 96% |
691MB | 267 MB | 65% | 69% | 70% | 86% | 99% | 98% |
346MB | 134 MB | 65% | 69% | 73% | 67% | 90% | 87% |
The raw numbers in seconds. I've only run the test once so far on the NAS and there are some other things running on it so I really should rerun it a few more times at least.
HEAD:
Table Size | Sort Size | 128MB | 64MB | 32MB | 16MB | 8MB | 4MB |
6914MB | 2672 MB | 1068.07 | 963.23 | 1041.94 | 1246.54 | 1654.35 | 2472.79 |
3457MB | 1336 MB | 529.34 | 482.3 | 450.77 | 555.76 | 657.34 | 1027.57 |
2765MB | 1069 MB | 404.02 | 394.36 | 348.31 | 414.48 | 507.38 | 657.17 |
1383MB | 535 MB | 196.48 | 194.26 | 173.48 | 182.57 | 214.42 | 258.05 |
691MB | 267 MB | 95.93 | 93.79 | 87.73 | 80.4 | 93.67 | 105.24 |
346MB | 134 MB | 45.6 | 44.24 | 42.39 | 44.22 | 46.17 | 49.85 |
With the quicksort patch:
Table Size | Sort Size | 128MB | 64MB | 32MB | 16MB | 8MB | 4MB |
6914MB | 2672 MB | 683.6 | 679.0 | 969.4 | 1366.2 | 2193.6 | 3379.3 |
3457MB | 1336 MB | 339.1 | 325.1 | 404.9 | 509.8 | 902.2 | 1229.1 |
2765MB | 1069 MB | 275.3 | 260.1 | 292.4 | 395.4 | 561.9 | 898.7 |
1383MB | 535 MB | 129.9 | 136.4 | 124.6 | 167.5 | 213.2 | 247.1 |
691MB | 267 MB | 62.3 | 64.3 | 61.4 | 69.2 | 92.3 | 103.2 |
346MB | 134 MB | 29.8 | 30.7 | 30.9 | 29.4 | 41.6 | 43.4 |
On Mon, Dec 14, 2015 at 6:58 PM, Greg Stark <stark@mit.edu> wrote: > I ran sorts with various parameters on my small NAS server. ... > without the extra memory optimizations. Thanks for taking the time to benchmark the patch! While I think it's perfectly fair that you didn't apply the final on-the-fly merge "memory pool" patch, I also think that it's quite possible that the regression you see at the very low end would be significantly ameliorated or even eliminated by applying that patch, too. After all, Jeff Janes had a much harder time finding a regression, probably because he benchmarked all patches together. -- Peter Geoghegan
On Mon, Dec 14, 2015 at 7:22 PM, Peter Geoghegan <pg@heroku.com> wrote: > Thanks for taking the time to benchmark the patch! Also, I should point out that you didn't add work_mem past the point where the master branch will get slower, while the patch continues to get faster. This seems to happen fairly reliably, certainly if work_mem is sized at about 1GB, and often at lower settings. With the POWER7 "Hydra" server, external sorting for a CREATE INDEX operation could put any possible maintenance_work_mem setting to good use -- my test case got faster with a 15GB maintenance_work_mem setting (the server has 64GB of ram). I think I tried 25GB as a maintenance_work_mem setting next, but started to get OOM errors at that point. Again, I point this out because I want to account for why my numbers were better (for the benefit of other people -- I think you get this, and are being fair). -- Peter Geoghegan
On Sat, Dec 12, 2015 at 5:28 PM, Peter Geoghegan <pg@heroku.com> wrote: > On Sat, Dec 12, 2015 at 12:10 AM, Jeff Janes <jeff.janes@gmail.com> wrote: >> I have a question about the terminology used in this patch. What is a >> tuple proper? What is it in contradistinction to? I would think that >> a tuple which is located in its own palloc'ed space is the "proper" >> one, leaving a tuple allocated in the bulk memory pool to be >> called...something else. I don't know what the >> non-judgmental-sounding antonym of postpositive "proper" is. > > "Tuple proper" is a term that appears 5 times in tuplesort.c today. As > it says at the top of that file: > > /* > * The objects we actually sort are SortTuple structs. These contain > * a pointer to the tuple proper (might be a MinimalTuple or IndexTuple), > * which is a separate palloc chunk --- we assume it is just one chunk and > * can be freed by a simple pfree(). SortTuples also contain the tuple's > * first key column in Datum/nullflag format, and an index integer. I see only three. In each case, "the tuple proper" could be replaced by "the tuple itself" or "the actual tuple" without changing the meaning, at least according to my understanding of the meaning. If that's causing confusion, perhaps we should just change the existing wording. Anyway, I agree with Jeff that this terminology shouldn't creep into function and structure member names. I don't really like the term "memory pool" either. We're growing a bunch of little special-purpose allocators all over the code base because of palloc's somewhat dubious performance and memory usage characteristics, but if any of those are referred to as memory pools it has thus far escaped my notice. -- Robert Haas EnterpriseDB: http://www.enterprisedb.com The Enterprise PostgreSQL Company
On Fri, Dec 18, 2015 at 10:12 AM, Robert Haas <robertmhaas@gmail.com> wrote: > Anyway, I agree with Jeff that this terminology shouldn't creep into > function and structure member names. Okay. > I don't really like the term "memory pool" either. We're growing a > bunch of little special-purpose allocators all over the code base > because of palloc's somewhat dubious performance and memory usage > characteristics, but if any of those are referred to as memory pools > it has thus far escaped my notice. It's a widely accepted term: https://en.wikipedia.org/wiki/Memory_pool But, sure, I'm not attached to it. -- Peter Geoghegan
On Fri, Dec 18, 2015 at 10:12 AM, Robert Haas <robertmhaas@gmail.com> wrote: > I don't really like the term "memory pool" either. We're growing a > bunch of little special-purpose allocators all over the code base > because of palloc's somewhat dubious performance and memory usage > characteristics, but if any of those are referred to as memory pools > it has thus far escaped my notice. BTW, I'm not necessarily determined to make the new special-purpose allocator work exactly as proposed. It seemed useful to prioritize simplicity, and currently so there is one big "huge palloc()" with which we blow our memory budget, and that's it. However, I could probably be more clever about "freeing ranges" initially preserved for a now-exhausted tape. That kind of thing. With the on-the-fly merge memory patch, I'm improving locality of access (for each "tuple proper"/"tuple itself"). If I also happen to improve the situation around palloc() fragmentation at the same time, then so much the better, but that's clearly secondary. -- Peter Geoghegan
On Fri, Dec 18, 2015 at 2:57 PM, Peter Geoghegan <pg@heroku.com> wrote: > On Fri, Dec 18, 2015 at 10:12 AM, Robert Haas <robertmhaas@gmail.com> wrote: >> I don't really like the term "memory pool" either. We're growing a >> bunch of little special-purpose allocators all over the code base >> because of palloc's somewhat dubious performance and memory usage >> characteristics, but if any of those are referred to as memory pools >> it has thus far escaped my notice. > > BTW, I'm not necessarily determined to make the new special-purpose > allocator work exactly as proposed. It seemed useful to prioritize > simplicity, and currently so there is one big "huge palloc()" with > which we blow our memory budget, and that's it. However, I could > probably be more clever about "freeing ranges" initially preserved for > a now-exhausted tape. That kind of thing. What about the case where we think that there will be a lot of data and have a lot of work_mem available, but then the user sends us 4 rows because of some mis-estimation? > With the on-the-fly merge memory patch, I'm improving locality of > access (for each "tuple proper"/"tuple itself"). If I also happen to > improve the situation around palloc() fragmentation at the same time, > then so much the better, but that's clearly secondary. I don't really understand this comment. -- Robert Haas EnterpriseDB: http://www.enterprisedb.com The Enterprise PostgreSQL Company
On Fri, Dec 18, 2015 at 12:50 PM, Robert Haas <robertmhaas@gmail.com> wrote: >> BTW, I'm not necessarily determined to make the new special-purpose >> allocator work exactly as proposed. It seemed useful to prioritize >> simplicity, and currently so there is one big "huge palloc()" with >> which we blow our memory budget, and that's it. However, I could >> probably be more clever about "freeing ranges" initially preserved for >> a now-exhausted tape. That kind of thing. > > What about the case where we think that there will be a lot of data > and have a lot of work_mem available, but then the user sends us 4 > rows because of some mis-estimation? The memory patch only changes the final on-the-fly merge phase. There is no estimate involved there. I continue to use whatever "slots" (memtuples) are available for the final on-the-fly merge. However, I allocate all remaining memory that I have budget for at once. My remarks about the efficient use of that memory was only really about each tape's use of their part of that over time. Again, to emphasize, this is only for the final on-the-fly merge phase. >> With the on-the-fly merge memory patch, I'm improving locality of >> access (for each "tuple proper"/"tuple itself"). If I also happen to >> improve the situation around palloc() fragmentation at the same time, >> then so much the better, but that's clearly secondary. > > I don't really understand this comment. I just mean that I wrote the memory patch with memory locality in mind, not palloc() fragmentation or other overhead. -- Peter Geoghegan
On Sun, Dec 6, 2015 at 7:25 PM, Peter Geoghegan <pg@heroku.com> wrote: > On Tue, Nov 24, 2015 at 4:33 PM, Peter Geoghegan <pg@heroku.com> wrote: >> So, the bottom line is: This patch seems very good, is unlikely to >> have any notable downside (no case has been shown to be regressed), >> but has yet to receive code review. I am working on a new version with >> the first two commits consolidated, and better comments, but that will >> have the same code, unless I find bugs or am dissatisfied. It mostly >> needs thorough code review, and to a lesser extent some more >> performance testing. > > I'm currently spending a lot of time working on parallel CREATE INDEX. > I should not delay posting a new version of my patch series any > further, though. I hope to polish up parallel CREATE INDEX to be able > to show people something in a couple of weeks. > > This version features consolidated commits, the removal of the > multipass_warning parameter, and improved comments and commit > messages. It has almost entirely unchanged functionality. > > The only functional changes are: > > * The function useselection() is taught to distrust an obviously bogus > caller reltuples hint (when it's already less than half of what we > know to be the minimum number of tuples that the sort must sort, > immediately after LACKMEM() first becomes true -- this is probably a > generic estimate). > > * Prefetching only occurs when writing tuples. Explicit prefetching > appears to hurt in some cases, as David Rowley has shown over on the > dedicated thread. But it might still be that writing tuples is a case > that is simple enough to benefit consistently, due to the relatively > uniform processing that memory latency can hide behind for that case > (before, the same prefetching instructions were used for CREATE INDEX > and for aggregates, for example). > > Maybe we should consider trying to get patch 0002 (the memory > pool/merge patch) committed first, something Greg Stark suggested > privately. That might actually be an easier way of integrating this > work, since it changes nothing about the algorithm we use for merging > (it only improves memory locality), and so is really an independent > piece of work (albeit one that makes a huge overall difference due to > the other patches increasing the time spent merging in absolute terms, > and especially as a proportion of the total). So I was looking at the 0001 patch and came across this code: + /* + * Crossover point is somewhere between where memtuples is between 40% + * and all-but-one of total tuples to sort. This weighs approximate + * savings in I/O, against generic heap sorting cost. + */ + avgTupleSize = (double) memNowUsed / (double) state->memtupsize; + + /* + * Starting from a threshold of 90%, refund 7.5% per 32 byte + * average-size-increment. + */ + increments = MAXALIGN_DOWN((int) avgTupleSize) / 32; + crossover = 0.90 - (increments * 0.075); + + /* + * Clamp, making either outcome possible regardless of average size. + * + * 40% is about the minimum point at which "quicksort with spillover" + * can still occur without a logical/physical correlation. + */ + crossover = Max(0.40, Min(crossover, 0.85)); + + /* + * The point where the overhead of maintaining the heap invariant is + * likely to dominate over any saving in I/O is somewhat arbitrarily + * assumed to be the point where memtuples' size exceeds MaxAllocSize + * (note that overall memory consumption may be far greater). Past + * this point, only the most compelling cases use replacement selection + * for their first run. + * + * This is not about cache characteristics so much as the O(n log n) + * cost of sorting larger runs dominating over the O(n) cost of + * writing/reading tuples. + */ + if (sizeof(SortTuple) * state->memtupcount > MaxAllocSize) + crossover = avgTupleSize > 32 ? 0.90 : 0.95; This looks like voodoo to me. I assume you tested it and maybe it gives correct answers, but it's got to be some kind of world record for number of arbitrary constants per SLOC, and there's no real justification for any of it. The comments say, essentially, well, we do this because it works. But suppose I try it on some new piece of hardware and it doesn't work well. What do I do? Email the author and ask him to tweak the arbitrary constants? The dependency on MaxAllocSize seems utterly bizarre to me. If we decide to modify our TOAST infrastructure so that we support datums up to 2GB in size, or alternatively datums of up to only 512MB in size, do you expect that to change the behavior of tuplesort.c? I bet not, but that's a major reason why MaxAllocSize is defined the way it is. I wonder if there's a way to accomplish what you're trying to do here that avoids the need to have a cost model at all. As I understand it, and please correct me wherever I go off the rails, the situation is: 1. If we're sorting a large amount of data, such that we can't fit it all in memory, we will need to produce a number of sorted runs and then merge those runs. If we generate each run using a heap with replacement selection, rather than quicksort, we will produce runs that are, on the average, about twice as long, which means that we will have fewer runs to merge at the end. 2. Replacement selection is slower than quicksort on a per-tuple basis. Furthermore, merging more runs isn't necessarily any slower than merging fewer runs. Therefore, building runs via replacement selection tends to lose even though it tends to reduce the number of runs to merge. Even when having a larger number of runs results in an increase in the number merge passes, we save so much time building the runs that we often (maybe not always) still come out ahead. 3. However, when replacement selection would result in a single run, and quicksort results in multiple runs, using quicksort loses. This is especially true when we the amount of data we have is between one and two times work_mem. If we fit everything into one run, we do not need to write any data to tape, but if we overflow by even a single tuple, we have to write a lot of data to tape. If this is correct so far, then I wonder if we could do this: Forget replacement selection. Always build runs by quicksorting. However, when dumping the first run to tape, dump it a little at a time rather than all at once. If the input ends before we've completely written the run, then we've got all of run 1 in memory and run 0 split between memory and tape. So we don't need to do any extra I/O; we can do a merge between run 1 and the portion of run 0 which is on tape. When the tape is exhausted, we only need to finish merging the in-memory tails of the two runs. I also wonder if you've thought about the case where we are asked to sort an enormous amount of data that is already in order, or very nearly in order (2,1,4,3,6,5,8,7,...). It seems worth including a check to see whether the low value of run N+1 is higher than the high value of run N, and if so, append it to the existing run rather than starting a new one. In some cases this could completely eliminate the final merge pass at very low cost, which seems likely to be worthwhile. Unfortunately, it's possible to fool this algorithm pretty easily - suppose the data is as in the parenthetical note in the previous paragraph, but the number of tuples that fits in work_mem is odd. I wonder if we can find instances where such cases regress significantly as compared with the replacement selection approach, which might be able to produce a single run out of an arbitrary amount of data. -- Robert Haas EnterpriseDB: http://www.enterprisedb.com The Enterprise PostgreSQL Company
On Tue, Dec 22, 2015 at 9:10 AM, Robert Haas <robertmhaas@gmail.com> wrote: > So I was looking at the 0001 patch Thanks. I'm going to produce a revision of 0002 shortly, so perhaps hold off on that one. The big change there will be to call grow_memtuples() to allow us to increase the number of slots without palloc() overhead spuriously being weighed (since the memory for the final on-the-fly merge phase doesn't have palloc() overhead). Also, will incorporate what Jeff and you wanted around terminology. > This looks like voodoo to me. I assume you tested it and maybe it > gives correct answers, but it's got to be some kind of world record > for number of arbitrary constants per SLOC, and there's no real > justification for any of it. The comments say, essentially, well, we > do this because it works. But suppose I try it on some new piece of > hardware and it doesn't work well. What do I do? Email the author > and ask him to tweak the arbitrary constants? That's not fair. DEFAULT_EQ_SEL, DEFAULT_RANGE_INEQ_SEL, and DEFAULT_NUM_DISTINCT are each about as arbitrary. We have to do something, though. MaxAllocHugeSize is used fairly arbitrarily in pg_stat_statements.c. And that part (the MaxAllocSize part of my patch) only defines a point after which we require a really favorable case for replacement selection/quicksort with spillover to proceed. It's a safety valve. We try to err on the side of not using replacement selection. > I wonder if there's a way to accomplish what you're trying to do here > that avoids the need to have a cost model at all. As I understand it, > and please correct me wherever I go off the rails, the situation is: > > 1. If we're sorting a large amount of data, such that we can't fit it > all in memory, we will need to produce a number of sorted runs and > then merge those runs. If we generate each run using a heap with > replacement selection, rather than quicksort, we will produce runs > that are, on the average, about twice as long, which means that we > will have fewer runs to merge at the end. > > 2. Replacement selection is slower than quicksort on a per-tuple > basis. Furthermore, merging more runs isn't necessarily any slower > than merging fewer runs. Therefore, building runs via replacement > selection tends to lose even though it tends to reduce the number of > runs to merge. Even when having a larger number of runs results in an > increase in the number merge passes, we save so much time building the > runs that we often (maybe not always) still come out ahead. I'm with you so far. I'll only add: doing multiple passes ought to be very rare anyway. > 3. However, when replacement selection would result in a single run, > and quicksort results in multiple runs, using quicksort loses. This > is especially true when we the amount of data we have is between one > and two times work_mem. If we fit everything into one run, we do not > need to write any data to tape, but if we overflow by even a single > tuple, we have to write a lot of data to tape. No, this is where you lose me. I think that it's basically not true that replacement selection can ever be faster than quicksort, even in the cases where the conventional wisdom would have you believe so (e.g. what you say here). Unless you have very little memory relative to data size, or something along those lines. The conventional wisdom obviously needs some revision, but it was perfectly correct in the 1970s and 1980s. However, where replacement selection can still help is avoiding I/O *entirely*. If we can avoid spilling 95% of tuples in the first place, and quicksort the remaining (heapified) tuples that were not spilled, and merge an in-memory run with an on-tape run, then we can win big. Quicksort is not amenable to incremental spilling at all. I call this "quicksort with spillover" (it is a secondary optimization that the patch adds). This shows up in EXPLAIN ANALYZE, and avoids a stark discontinuity in the cost function of sorts. That could really help with admission control, and simplifying the optimizer, making merge joins less scary. So with the patch, "quicksort with spillover" and "replacement selection" are almost synonymous, except that we acknowledge the historic importance of replacement selection to some degree. The patch completely discards the conventional use of replacement selection -- it just preserves its priority queue (heap) implementation where incrementalism is thought to be particularly useful (avoiding I/O entirely). But this comparison has nothing to do with comparing the master branch with my patch, since the master branch never attempts to avoid I/O having committed to an external sort. It uses replacement selection in a way that is consistent with the conventional wisdom, wisdom which has now been shown to be obsolete. BTW, I think that abandoning incrementalism (replacement selection) will have future benefits for memory management. I bet we can get away with one big palloc() for second or subsequent runs that are quicksorted, greatly reducing palloc() overhead and waste there, too. > If this is correct so far, then I wonder if we could do this: Forget > replacement selection. Always build runs by quicksorting. However, > when dumping the first run to tape, dump it a little at a time rather > than all at once. If the input ends before we've completely written > the run, then we've got all of run 1 in memory and run 0 split between > memory and tape. So we don't need to do any extra I/O; we can do a > merge between run 1 and the portion of run 0 which is on tape. When > the tape is exhausted, we only need to finish merging the in-memory > tails of the two runs. My first attempt at this -- before I realized that replacement selection was just not a very good algorithm, due to the upsides not remotely offsetting the downsides on modern hardware -- was a hybrid between quicksort and replacement selection. The problem is that there is too much repeated work. If you spill like this, you have to quicksort everything again. The replacement selection queue keeps track of a currentRun and nextRun, to avoid this, but quicksort can't really do that well. In general, the replacement selection heap will create a new run that cannot be spilled (nextRun -- there won't be one initially) if there is a value less than any of those values already spilled to tape. So it is built to avoid redundant work in a way that quicksort really cannot be. > I also wonder if you've thought about the case where we are asked to > sort an enormous amount of data that is already in order, or very > nearly in order (2,1,4,3,6,5,8,7,...). It seems worth including a > check to see whether the low value of run N+1 is higher than the high > value of run N, and if so, append it to the existing run rather than > starting a new one. In some cases this could completely eliminate the > final merge pass at very low cost, which seems likely to be > worthwhile. While I initially shared this intuition -- that replacement selection could hardly be beaten by a simple hybrid sort-merge strategy for almost sorted input -- I changed my mind. I simply did not see any evidence for it. I may have missed something, but it really does not appear to be worth while. The quicksort fallback to insertion sort also does well with presorted input. The merge is very cheap (over and above reading one big run off disk) for presorted input under most circumstances. A cost model adds a lot of complexity, which I hesitate to add without clear benefits. -- Peter Geoghegan
On Tue, Dec 22, 2015 at 4:37 PM, Peter Geoghegan <pg@heroku.com> wrote: >> This looks like voodoo to me. I assume you tested it and maybe it >> gives correct answers, but it's got to be some kind of world record >> for number of arbitrary constants per SLOC, and there's no real >> justification for any of it. The comments say, essentially, well, we >> do this because it works. But suppose I try it on some new piece of >> hardware and it doesn't work well. What do I do? Email the author >> and ask him to tweak the arbitrary constants? > > That's not fair. DEFAULT_EQ_SEL, DEFAULT_RANGE_INEQ_SEL, and > DEFAULT_NUM_DISTINCT are each about as arbitrary. We have to do > something, though. > > MaxAllocHugeSize is used fairly arbitrarily in pg_stat_statements.c. > And that part (the MaxAllocSize part of my patch) only defines a point > after which we require a really favorable case for replacement > selection/quicksort with spillover to proceed. It's a safety valve. We > try to err on the side of not using replacement selection. Sure, there are arbitrary numbers all over the code, driven by empirical observations about what factors are important to model. But this is not that. You don't have a thing called seq_page_cost and a thing called cpu_tuple_cost and then say, well, empirically the ratio is about 100:1, so let's make the former 1 and the latter 0.01. You just have some numbers, and it's not clear what, if anything, they actually represent. In the space of 7 lines of code, you introduce 9 nameless constants: The crossover point is clamped to a minimum of 40% [constant #1] and a maximum of 85% [constant #2] when the size of the SortTuple array is no more than MaxAllocSize. Between those bounds, the crossover point is 90% [constant #3] minus 7.5% [constant #4] per 32-byte increment [constant #5] of estimated average tuple size. On the other hand, when the estimated average tuple size exceeds MaxAllocSize, the crossover point is either 90% [constant #6] or 95% [constant #7] depending on whether the average tuple size is greater than 32 bytes [constant #8]. But if the row count hit is less than 50% [constant #9] of the rows we've already seen, then we ignore it and do not use selection. You make no attempt to justify why any of these numbers are correct, or what underlying physical reality they represent. The comment which describes the manner in which crossover point is computed for SortTuple volumes under 1GB says "Starting from a threshold of 90%, refund 7.5% per 32 byte average-size-increment." That is a precise restatement of what the code does, but it doesn't attempt to explain why it's a good idea. Perhaps the reader should infer that the crossover point drops as the tuples get bigger, except that in the over-1GB case, a larger tuple size causes the crossover point to go *up* while in the under-1GB case, a larger tuple size causes the crossover point to go *down*. Concretely, if we're sorting 44,739,242 224-byte tuples, the estimated crossover point is 40%. If we're sorting 44,739,243 244-byte tuples, the estimated crossover point is 95%. That's an extremely sharp discontinuity, and it seems very unlikely that any real system behaves that way. I'm prepared to concede that constant #9 - ignoring the input row estimate if we've already seen twice that many rows - probably doesn't need a whole lot of justification here, and what justification it does need is provided by the fact that (we think) replacement selection only wins when there are going to be less than 2 quicksorted runs. But the other 8 constants here have to have reasons why they exist, what they represent, and why they have the values they do, and that explanation needs to be something that can be understood by people besides you. The overall cost model needs some explanation of the theory of operation, too. In my opinion, reasoning in terms of a crossover point is a strange way of approaching the problem. What would be more typical at least in our code, and I suspect in general, is do a cost estimate of using selection and a cost estimate of not using selection and compare them. Replacement selection has a CPU cost and an I/O cost, each of which is estimable based on the tuple count, chosen comparator, and expected I/O volume. Quicksort has those same costs, in different amounts. If those respective costs are accurately estimated, then you can pick the strategy with the lower cost and expect to win. >> I wonder if there's a way to accomplish what you're trying to do here >> that avoids the need to have a cost model at all. As I understand it, >> and please correct me wherever I go off the rails, the situation is: >> >> 1. If we're sorting a large amount of data, such that we can't fit it >> all in memory, we will need to produce a number of sorted runs and >> then merge those runs. If we generate each run using a heap with >> replacement selection, rather than quicksort, we will produce runs >> that are, on the average, about twice as long, which means that we >> will have fewer runs to merge at the end. >> >> 2. Replacement selection is slower than quicksort on a per-tuple >> basis. Furthermore, merging more runs isn't necessarily any slower >> than merging fewer runs. Therefore, building runs via replacement >> selection tends to lose even though it tends to reduce the number of >> runs to merge. Even when having a larger number of runs results in an >> increase in the number merge passes, we save so much time building the >> runs that we often (maybe not always) still come out ahead. > > I'm with you so far. I'll only add: doing multiple passes ought to be > very rare anyway. > >> 3. However, when replacement selection would result in a single run, >> and quicksort results in multiple runs, using quicksort loses. This >> is especially true when we the amount of data we have is between one >> and two times work_mem. If we fit everything into one run, we do not >> need to write any data to tape, but if we overflow by even a single >> tuple, we have to write a lot of data to tape. > > No, this is where you lose me. I think that it's basically not true > that replacement selection can ever be faster than quicksort, even in > the cases where the conventional wisdom would have you believe so > (e.g. what you say here). Unless you have very little memory relative > to data size, or something along those lines. The conventional wisdom > obviously needs some revision, but it was perfectly correct in the > 1970s and 1980s. > > However, where replacement selection can still help is avoiding I/O > *entirely*. If we can avoid spilling 95% of tuples in the first place, > and quicksort the remaining (heapified) tuples that were not spilled, > and merge an in-memory run with an on-tape run, then we can win big. That's pretty much what I was trying to say, except that I'm curious to know whether replacement selection can win when it manages to generate a vastly longer run than what we get from quicksorting. Say quicksorting produces 10, or 100, or 1000 tapes, and replacement selection produces 1 due to a favorable data distribution. > Quicksort is not amenable to incremental spilling at all. I call this > "quicksort with spillover" (it is a secondary optimization that the > patch adds). This shows up in EXPLAIN ANALYZE, and avoids a stark > discontinuity in the cost function of sorts. That could really help > with admission control, and simplifying the optimizer, making merge > joins less scary. So with the patch, "quicksort with spillover" and > "replacement selection" are almost synonymous, except that we > acknowledge the historic importance of replacement selection to some > degree. The patch completely discards the conventional use of > replacement selection -- it just preserves its priority queue (heap) > implementation where incrementalism is thought to be particularly > useful (avoiding I/O entirely). > > But this comparison has nothing to do with comparing the master branch > with my patch, since the master branch never attempts to avoid I/O > having committed to an external sort. It uses replacement selection in > a way that is consistent with the conventional wisdom, wisdom which > has now been shown to be obsolete. > > BTW, I think that abandoning incrementalism (replacement selection) > will have future benefits for memory management. I bet we can get away > with one big palloc() for second or subsequent runs that are > quicksorted, greatly reducing palloc() overhead and waste there, too. > >> If this is correct so far, then I wonder if we could do this: Forget >> replacement selection. Always build runs by quicksorting. However, >> when dumping the first run to tape, dump it a little at a time rather >> than all at once. If the input ends before we've completely written >> the run, then we've got all of run 1 in memory and run 0 split between >> memory and tape. So we don't need to do any extra I/O; we can do a >> merge between run 1 and the portion of run 0 which is on tape. When >> the tape is exhausted, we only need to finish merging the in-memory >> tails of the two runs. > > My first attempt at this -- before I realized that replacement > selection was just not a very good algorithm, due to the upsides not > remotely offsetting the downsides on modern hardware -- was a hybrid > between quicksort and replacement selection. > > The problem is that there is too much repeated work. If you spill like > this, you have to quicksort everything again. The replacement > selection queue keeps track of a currentRun and nextRun, to avoid > this, but quicksort can't really do that well. I agree, but that's not what I proposed. You don't want to keep re-sorting to incorporate new tuples into the run, but if you've got 1010 tuples and you can fit 1000 tuples in, you can (a) quicksort the first 1000 tuples, (b) read in 10 more tuples, dumping the first 10 tuples from run 0 to disk, (c) quicksort the last 10 tuples to create run 1, and then (d) merge run 0 [which is mostly in memory] with run 1 [which is entirely in memory]. In other words, yes, quicksorting doesn't let you add things to the sort incrementally, but you can still write out the run incrementally, writing only as many tuples as you need to dump to get the rest of the input data into memory. >> I also wonder if you've thought about the case where we are asked to >> sort an enormous amount of data that is already in order, or very >> nearly in order (2,1,4,3,6,5,8,7,...). It seems worth including a >> check to see whether the low value of run N+1 is higher than the high >> value of run N, and if so, append it to the existing run rather than >> starting a new one. In some cases this could completely eliminate the >> final merge pass at very low cost, which seems likely to be >> worthwhile. > > While I initially shared this intuition -- that replacement selection > could hardly be beaten by a simple hybrid sort-merge strategy for > almost sorted input -- I changed my mind. I simply did not see any > evidence for it. I may have missed something, but it really does not > appear to be worth while. The quicksort fallback to insertion sort > also does well with presorted input. The merge is very cheap (over and > above reading one big run off disk) for presorted input under most > circumstances. A cost model adds a lot of complexity, which I hesitate > to add without clear benefits. I don't think you need any kind of cost model to implement the approach of appending to an existing run when the values in the new run are strictly greater. -- Robert Haas EnterpriseDB: http://www.enterprisedb.com The Enterprise PostgreSQL Company
On Tue, Dec 22, 2015 at 2:57 PM, Robert Haas <robertmhaas@gmail.com> wrote: > On Tue, Dec 22, 2015 at 4:37 PM, Peter Geoghegan <pg@heroku.com> wrote: >> That's not fair. DEFAULT_EQ_SEL, DEFAULT_RANGE_INEQ_SEL, and >> DEFAULT_NUM_DISTINCT are each about as arbitrary. We have to do >> something, though. >> > Sure, there are arbitrary numbers all over the code, driven by > empirical observations about what factors are important to model. But > this is not that. You don't have a thing called seq_page_cost and a > thing called cpu_tuple_cost and then say, well, empirically the ratio > is about 100:1, so let's make the former 1 and the latter 0.01. You > just have some numbers, and it's not clear what, if anything, they > actually represent. What I find difficult to accept about what you say here is that at *this* level, something like cost_sort() has little to recommend it. It costs a sort of a text attribute at the same level as the cost of sorting the same tuples using an int4 attribute (based on the default cpu_operator_cost for C functions -- without any attempt to differentiate text and int4). Prior to 9.5, sorting text took about 5 - 10 times longer that this similar int4 sort. That's a pretty big difference, and yet I recall no complaints. The cost of a comparison in a sort can hardly be considered in isolation, anyway -- cache efficiency is at least as important. Of course, the point is that the goal of a cost model is not to simulate reality as closely as possible -- it's to produce a good outcome for performance purposes under realistic assumptions. Realistic assumptions include that you can't hope to account for certain differences in cost. Avoiding a terrible outcome is very important, but the worst case for useselection() is no worse than today's behavior (or a lost opportunity to do better than today's behavior). Recently, the paper that was posted to the list about the Postgres optimizer stated formally what I know I had a good intuitive sense of for a long time: that better selectivity estimates are much more important than better cost models in practice. The "empirical observations" driving something like DEFAULT_EQ_SEL are very weak -- but what are you gonna do? > The crossover point is clamped to a minimum of 40% [constant #1] and a > maximum of 85% [constant #2] when the size of the SortTuple array is > no more than MaxAllocSize. Between those bounds, the crossover point > is 90% [constant #3] minus 7.5% [constant #4] per 32-byte increment > [constant #5] of estimated average tuple size. On the other hand, > when the estimated average tuple size exceeds MaxAllocSize, the > crossover point is either 90% [constant #6] or 95% [constant #7] > depending on whether the average tuple size is greater than 32 bytes > [constant #8]. But if the row count hit is less than 50% [constant > #9] of the rows we've already seen, then we ignore it and do not use > selection. > > You make no attempt to justify why any of these numbers are correct, > or what underlying physical reality they represent. Just like selfuncs.h for the most part, then. > The comment which > describes the manner in which crossover point is computed for > SortTuple volumes under 1GB says "Starting from a threshold of 90%, > refund 7.5% per 32 byte average-size-increment." That is a precise > restatement of what the code does, but it doesn't attempt to explain > why it's a good idea. Perhaps the reader should infer that the > crossover point drops as the tuples get bigger, except that in the > over-1GB case, a larger tuple size causes the crossover point to go > *up* while in the under-1GB case, a larger tuple size causes the > crossover point to go *down*. Concretely, if we're sorting 44,739,242 > 224-byte tuples, the estimated crossover point is 40%. If we're > sorting 44,739,243 244-byte tuples, the estimated crossover point is > 95%. That's an extremely sharp discontinuity, and it seems very > unlikely that any real system behaves that way. Again, the goal of the cost model is not to model reality as such. This cost model is conservative about using replacement selection. It makes sense when you consider that there tends to be a lot fewer external sorts on a realistic workload -- if we can cut that number in half, which seems quite possible, that's pretty good, especially from a DBA's practical perspective. I want to buffer DBAs against suddenly incurring more I/O, but not at the risk of having a far longer sort for the first run. Or with minimal exposure to that risk. The cost model weighs the cost of the hint being wrong to some degree (which is indeed novel). I think it makes sense in light of the cost and benefits in this case, although I will add that I'm not entirely comfortable with it. I just don't imagine that there is a solution that I will be fully comfortable with. There may be one that superficially looks correct, but I see little point in that. > I'm prepared to concede that constant #9 - ignoring the input row > estimate if we've already seen twice that many rows - probably doesn't > need a whole lot of justification here, and what justification it does > need is provided by the fact that (we think) replacement selection > only wins when there are going to be less than 2 quicksorted runs. > But the other 8 constants here have to have reasons why they exist, > what they represent, and why they have the values they do, and that > explanation needs to be something that can be understood by people > besides you. The overall cost model needs some explanation of the > theory of operation, too. The cost model is extremely fudged. I think that the greatest problem that it has is that it isn't explicit enough about that. But yes, let me concede more clearly: the cost model is based on frobbing. But at least it's relatively honest about that, and is relatively simple. I think it might be possible to make it simpler, but I have a feeling that anything we can come up with will basically have the same quality that you so dislike. I don't know how to do better. Frankly, I'd rather be roughly correct than exactly wrong. > In my opinion, reasoning in terms of a crossover point is a strange > way of approaching the problem. What would be more typical at least > in our code, and I suspect in general, is do a cost estimate of using > selection and a cost estimate of not using selection and compare them. > Replacement selection has a CPU cost and an I/O cost, each of which is > estimable based on the tuple count, chosen comparator, and expected > I/O volume. Quicksort has those same costs, in different amounts. If > those respective costs are accurately estimated, then you can pick the > strategy with the lower cost and expect to win. If you instrument the number of comparisons, I expect you'll find that master is very competitive with the patch in terms of number of comparisons performed in total. I think it might even win (Knuth specifically addresses this, actually). Where does that leave your theory of how to build a cost model? Also, the disadvantage of replacement selection's heap is smaller with smaller work_mem settings -- this has been shown many times to make a *huge* difference. Can the alternative cost model be reasonably expected to incorporate that, too? Heap sort isn't cache oblivious, which is why we see these weird effects, so don't forget to have CPU cache size as an input into your cost model (or maybe use a magic value based on something like MaxAllocSize!). How do you propose to way the distributed cost of a lost opportunity to reduce I/O against the distributed cost of heapsort wasting system memory bandwidth? And so on, and so on...believe me, I could go on. By the way, I think that there needs to be a little work done to cost_sort() too, which so far I've avoided. >> However, where replacement selection can still help is avoiding I/O >> *entirely*. If we can avoid spilling 95% of tuples in the first place, >> and quicksort the remaining (heapified) tuples that were not spilled, >> and merge an in-memory run with an on-tape run, then we can win big. > > That's pretty much what I was trying to say, except that I'm curious > to know whether replacement selection can win when it manages to > generate a vastly longer run than what we get from quicksorting. Say > quicksorting produces 10, or 100, or 1000 tapes, and replacement > selection produces 1 due to a favorable data distribution. I believe the answer is probably no, but if there is a counter example, it probably isn't worth pursuing. To repeat myself, I started out with exactly the same intuition as you on that question, but changed my mind when my efforts to experimentally verify the intuition were not successful. > I agree, but that's not what I proposed. You don't want to keep > re-sorting to incorporate new tuples into the run, but if you've got > 1010 tuples and you can fit 1000 tuples in, you can (a) quicksort the > first 1000 tuples, (b) read in 10 more tuples, dumping the first 10 > tuples from run 0 to disk, (c) quicksort the last 10 tuples to create > run 1, and then (d) merge run 0 [which is mostly in memory] with run 1 > [which is entirely in memory]. In other words, yes, quicksorting > doesn't let you add things to the sort incrementally, but you can > still write out the run incrementally, writing only as many tuples as > you need to dump to get the rest of the input data into memory. Merging is still sorting. The 10 tuples are not very cheap to merge against the 1000 tuples, because you'll probably still end up reading most of the 1000 tuples to do so. Perhaps you anticipate that there will be roughly disjoint ranges of values in each run due to a logical/physical correlation, and so you won't have to read that many of the 1000 tuples, but this approach has no ability to buffer even one outlier value (unlike replacement selection, in particular my approach within mergememruns()). The cost of heapification of 1.01 million tuples to spill 0.01 million tuples is pretty low (relative to the cost of sorting them in particular). The only difference between what you say here and what I actually do is that the remaining tuples are heapified rather than sorted, and I quicksort everything together to "merge run 1 and run 0" rather than doing two quicksorts and a merge. I believe that this can be demonstrated to be cheaper. Another factor is that the heap could be useful for other stuff in the future. As Simon Riggs pointed out, for deduplicating values as they're read in by tuplesort. (Okay, that's really the only other thing, but it's a good one). -- Peter Geoghegan
On Tue, Dec 22, 2015 at 8:10 PM, Peter Geoghegan <pg@heroku.com> wrote: >> Sure, there are arbitrary numbers all over the code, driven by >> empirical observations about what factors are important to model. But >> this is not that. You don't have a thing called seq_page_cost and a >> thing called cpu_tuple_cost and then say, well, empirically the ratio >> is about 100:1, so let's make the former 1 and the latter 0.01. You >> just have some numbers, and it's not clear what, if anything, they >> actually represent. > > What I find difficult to accept about what you say here is that at > *this* level, something like cost_sort() has little to recommend it. > It costs a sort of a text attribute at the same level as the cost of > sorting the same tuples using an int4 attribute (based on the default > cpu_operator_cost for C functions -- without any attempt to > differentiate text and int4). > > Prior to 9.5, sorting text took about 5 - 10 times longer that this > similar int4 sort. That's a pretty big difference, and yet I recall no > complaints. The cost of a comparison in a sort can hardly be > considered in isolation, anyway -- cache efficiency is at least as > important. > > Of course, the point is that the goal of a cost model is not to > simulate reality as closely as possible -- it's to produce a good > outcome for performance purposes under realistic assumptions. > Realistic assumptions include that you can't hope to account for > certain differences in cost. Avoiding a terrible outcome is very > important, but the worst case for useselection() is no worse than > today's behavior (or a lost opportunity to do better than today's > behavior). I agree with that. So, the question for any given cost model is: does it model the effects that matter? If you think that the cost of sorting integers vs. sorting text matters to the crossover point, then that should be modeled here. If it doesn't matter, then don't include it. The point is, nobody can tell WHAT effects this is modeling. Increasing the tuple size makes the crossover go up. Or down. > Recently, the paper that was posted to the list about the Postgres > optimizer stated formally what I know I had a good intuitive sense of > for a long time: that better selectivity estimates are much more > important than better cost models in practice. The "empirical > observations" driving something like DEFAULT_EQ_SEL are very weak -- > but what are you gonna do? This analogy is faulty. It's true that when we run across a qual whose selectivity we cannot estimate in any meaningful way, we have to just take a stab in the dark and hope for the best. Similarly, if we have no information about what the crossover point for a given sort is, we'd have to take some arbitrary estimate, like 75%, and hope for the best. But in this case, we DO have information. We have an estimated row count and an estimated row width. And those values are not being ignored, they are getting used. The problem is that they are being used in an arbitrary way that is not justified by any chain of reasoning. > But yes, let me concede more clearly: the cost model is based on > frobbing. But at least it's relatively honest about that, and is > relatively simple. I think it might be possible to make it simpler, > but I have a feeling that anything we can come up with will basically > have the same quality that you so dislike. I don't know how to do > better. Frankly, I'd rather be roughly correct than exactly wrong. Sure, but the fact that the model has huge discontinuities - perhaps most notably a case where adding a single tuple to the estimated cardinality changes the crossover point by a factor of two - suggests that you are probably wrong. The actual behavior does not change sharply when the size of the SortTuple array crosses 1GB, but the estimates do. That means that either the estimates are wrong for 44,739,242 tuples or they are wrong for 44,739,243 tuples. The behavior cannot be right in both cases unless that one extra tuple changes the behavior radically, or unless the estimate doesn't matter in the first place. > By the way, I think that there needs to be a little work done to > cost_sort() too, which so far I've avoided. Yeah, I agree, but that can be a separate topic. >> I agree, but that's not what I proposed. You don't want to keep >> re-sorting to incorporate new tuples into the run, but if you've got >> 1010 tuples and you can fit 1000 tuples in, you can (a) quicksort the >> first 1000 tuples, (b) read in 10 more tuples, dumping the first 10 >> tuples from run 0 to disk, (c) quicksort the last 10 tuples to create >> run 1, and then (d) merge run 0 [which is mostly in memory] with run 1 >> [which is entirely in memory]. In other words, yes, quicksorting >> doesn't let you add things to the sort incrementally, but you can >> still write out the run incrementally, writing only as many tuples as >> you need to dump to get the rest of the input data into memory. > > Merging is still sorting. The 10 tuples are not very cheap to merge > against the 1000 tuples, because you'll probably still end up reading > most of the 1000 tuples to do so. You're going to read all of the 1000 tuples no matter what, because you need to return them, but you will also need to make comparisons on most of them, unless the data distribution is favorable. Assuming no special good luck, it'll take something close to X + Y - 1 comparisons to do the merge, so something around 1009 comparisons here. Maintaining the heap property is not free either, but it might be cheaper. > The cost of heapification of 1.01 million tuples to spill 0.01 million > tuples is pretty low (relative to the cost of sorting them in > particular). The only difference between what you say here and what I > actually do is that the remaining tuples are heapified rather than > sorted, and I quicksort everything together to "merge run 1 and run 0" > rather than doing two quicksorts and a merge. I believe that this can > be demonstrated to be cheaper. > > Another factor is that the heap could be useful for other stuff in the > future. As Simon Riggs pointed out, for deduplicating values as > they're read in by tuplesort. (Okay, that's really the only other > thing, but it's a good one). Not sure how that would work? -- Robert Haas EnterpriseDB: http://www.enterprisedb.com The Enterprise PostgreSQL Company
On Wed, Dec 23, 2015 at 9:37 AM, Robert Haas <robertmhaas@gmail.com> wrote: > The point is, nobody can tell WHAT effects this is modeling. > Increasing the tuple size makes the crossover go up. Or down. There are multiple, competing considerations. > This analogy is faulty. It's true that when we run across a qual > whose selectivity we cannot estimate in any meaningful way, we have to > just take a stab in the dark and hope for the best. Similarly, if we > have no information about what the crossover point for a given sort > is, we'd have to take some arbitrary estimate, like 75%, and hope for > the best. But in this case, we DO have information. We have an > estimated row count and an estimated row width. And those values are > not being ignored, they are getting used. The problem is that they > are being used in an arbitrary way that is not justified by any chain > of reasoning. There is a chain of reasoning. It's not particularly satisfactory that it's so fuzzy, certainly, but the competing considerations here are substantive (and include erring towards not proceeding with replacement selection/"quicksort with spillover" when the benefits are low relative to the costs, which, to repeat myself, is itself novel). I am more than open to suggestions on alternatives. As I said, I don't particularly care for my current approach, either. But doing something analogous to cost_sort() for our private "Do we quicksort with spillover?"/useselection() model is going to be strictly worse than what I have proposed. Any cost model will have to be sensitive to different types of CPU costs at the level that matters here -- such as the size of the heap, and its cache efficiency. That's really important, but very complicated, and variable enough that erring against using replacement selection seems like a good idea with bigger heaps especially. That (cache efficiency) is theoretically the only difference that matters here (other than I/O, of course, but avoiding I/O is only the upside of proceeding, and if we only weigh that then the cost model always gives the same answer). Perhaps you can suggest an alternative model that weighs these factors. Most sorts are less than 1GB, and it seems worthwhile to avoid I/O at the level where an internal sort is just out of reach. Really big CREATE INDEX sorts are not really what I have in mind with "quicksort with spillover". This cost_sort() code seems pretty bogus to me, FWIW: /* Assume 3/4ths of accesses are sequential, 1/4th are not */ startup_cost += npageaccesses * (seq_page_cost* 0.75 + random_page_cost * 0.25); I think we can afford to be a lot more optimistic about the proportion of sequential accesses. >> Merging is still sorting. The 10 tuples are not very cheap to merge >> against the 1000 tuples, because you'll probably still end up reading >> most of the 1000 tuples to do so. > > You're going to read all of the 1000 tuples no matter what, because > you need to return them, but you will also need to make comparisons on > most of them, unless the data distribution is favorable. Assuming no > special good luck, it'll take something close to X + Y - 1 comparisons > to do the merge, so something around 1009 comparisons here. > Maintaining the heap property is not free either, but it might be > cheaper. I'm pretty sure that it's cheaper. Some of the really good cases for "quicksort with spillover" where only a little bit slower than a fully internal sort when the work_mem threshold was just crossed. >> Another factor is that the heap could be useful for other stuff in the >> future. As Simon Riggs pointed out, for deduplicating values as >> they're read in by tuplesort. (Okay, that's really the only other >> thing, but it's a good one). > > Not sure how that would work? Tuplesort would have license to discard tuples with matching existing values, because the caller gave it permission to. This is something that you can easily imagine occurring with ordered set aggregates, for example. It would work in a way not unlike a top-N heapsort does today. This would work well when it can substantially lower the use of memory (initially heapification when the threshold is crossed would probably measure the number of duplicates, and proceed only when it looked like a promising strategy). By the way, I think the heap currently does quite badly with many duplicated values. That case seemed significantly slower than a similar case with high cardinality tuples. -- Peter Geoghegan
On Mon, Dec 14, 2015 at 7:22 PM, Peter Geoghegan <pg@heroku.com> wrote: > On Mon, Dec 14, 2015 at 6:58 PM, Greg Stark <stark@mit.edu> wrote: >> I ran sorts with various parameters on my small NAS server. > > ... > >> without the extra memory optimizations. > > Thanks for taking the time to benchmark the patch! > > While I think it's perfectly fair that you didn't apply the final > on-the-fly merge "memory pool" patch, I also think that it's quite > possible that the regression you see at the very low end would be > significantly ameliorated or even eliminated by applying that patch, > too. After all, Jeff Janes had a much harder time finding a > regression, probably because he benchmarked all patches together. The regression I found when building an index on a column of 400,000,000 md5(random()::text) with 64MB maintenance_work_mem was not hard to find at all. I still don't understand what is going on with it, but it is reproducible. Perhaps it is very unlikely and I just got very lucky in finding it immediately after switching to that data-type for my tests, but I wouldn't assume that on current evidence. If we do think it is important to almost never cause regressions at the default maintenance_work_mem (I am agnostic on the importance of that), then I think we have more work to do here. I just don't know what that work is. Cheers, Jeff
On Wed, Dec 23, 2015 at 1:03 PM, Jeff Janes <jeff.janes@gmail.com> wrote: > If we do think it is important to almost never cause regressions at > the default maintenance_work_mem (I am agnostic on the importance of > that), then I think we have more work to do here. I just don't know > what that work is. My next revision will use grow_memtuples() in advance of the final on-the-fly merge step, in a way that considers that we won't be losing out to palloc() overhead (so it'll mostly be the memory patch that is revised). This can make a large difference to the number of slots (memtuples) available. I think I measured a 6% or 7% additional improvement for a case with a fairly small number of runs to merge. It might help significantly more when there are more runs to merge. -- Peter Geoghegan
On Wed, Dec 23, 2015 at 3:31 PM, Peter Geoghegan <pg@heroku.com> wrote: > On Wed, Dec 23, 2015 at 9:37 AM, Robert Haas <robertmhaas@gmail.com> wrote: >> The point is, nobody can tell WHAT effects this is modeling. >> Increasing the tuple size makes the crossover go up. Or down. > > There are multiple, competing considerations. Please explain what they are and how they lead you to believe that the cost factors you have chosen are good ones. My point here is: even if I were to concede that your cost model yields perfect answers in every case, the patch needs to give at least some hint as to why. Right now, it really doesn't. >>> Another factor is that the heap could be useful for other stuff in the >>> future. As Simon Riggs pointed out, for deduplicating values as >>> they're read in by tuplesort. (Okay, that's really the only other >>> thing, but it's a good one). >> >> Not sure how that would work? > > Tuplesort would have license to discard tuples with matching existing > values, because the caller gave it permission to. This is something > that you can easily imagine occurring with ordered set aggregates, for > example. It would work in a way not unlike a top-N heapsort does > today. This would work well when it can substantially lower the use of > memory (initially heapification when the threshold is crossed would > probably measure the number of duplicates, and proceed only when it > looked like a promising strategy). It's not clear to me how having a heap helps with that. -- Robert Haas EnterpriseDB: http://www.enterprisedb.com The Enterprise PostgreSQL Company
On Wed, Dec 23, 2015 at 1:16 PM, Robert Haas <robertmhaas@gmail.com> wrote: > On Wed, Dec 23, 2015 at 3:31 PM, Peter Geoghegan <pg@heroku.com> wrote: >> On Wed, Dec 23, 2015 at 9:37 AM, Robert Haas <robertmhaas@gmail.com> wrote: >>> The point is, nobody can tell WHAT effects this is modeling. >>> Increasing the tuple size makes the crossover go up. Or down. >> >> There are multiple, competing considerations. > > Please explain what they are and how they lead you to believe that the > cost factors you have chosen are good ones. Alright. I've gone on at length about how I'm blurring the distinction between internal and external sorting, or about how modern hardware characteristics allow that. There are several reasons for that. Now, we all know that main memory sizes have increased dramatically since the 1970s, and storage characteristics are very different, and that CPU caching effects have become very important, and that everyone has lots more data. There is one thing that hasn't really become bigger in all that time, though: the width of tuples. So, as I go into in comments within useselection(), that's the main reason why avoiding I/O isn't all that impressive, especially at the high end. It's just not that big of a cost at the high end. Beyond that, as linear costs go, palloc() is a much bigger concern to me at this point. I think we can waste a lot less time by amortizing that more extensively (to say nothing of the saving in memory). This is really obvious by just looking at trace_sort output with my patch applied when dealing with many runs, sorting millions of tuples: There just isn't that much time spent on I/O at all, and it's well hidden by foreground processing that is CPU bound. With smaller work_mem sizes and far fewer tuples, a case much more common within sort nodes (as opposed to utility statements), this is less true. Sorting 1,000 or 10,000 tuples is an entirely different thing to sorting 1,000,000 tuples. So, first of all, the main consideration is that saving I/O turns out to not matter that much at the high end. That's why we get very conservative past the fairly arbitrary MaxAllocSize memtuples threshold (which has a linear relationship to the number of tuples -- *not* the amount of memory used or disk space that may be used). A second consideration is how much I/O we can save -- one would hope it would be a lot, certainly the majority, to make up for the downside of using a cache inefficient technique. That is a different thing to the number of memtuples. If you had really huge tuples, there would be a really big saving in I/O, often without a corresponding degradation in cache performance (since there still many not be that many memtuples, which is more the problem for the heap than anything else). This distinction is especially likely to matter for the CLUSTER case, where wide heap tuples (including heap tuple headers, visibility info) are kind of along for the ride, which is less true elsewhere, particularly for the CREATE INDEX case. The cache inefficiency of spilling incrementally from a heap isn't so bad if we only end up sorting a small number of tuples that way. So as the number of tuples that we end up actually sorting that way increases, the cache inefficiency becomes worse, while at the same time, we save less I/O. The former is a bigger problem than the latter, by a wide margin, I believe. This code is an attempt to credit cases with really wide tuples: /* * Starting from a threshold of 90%, refund 7.5% per 32 byte * average-size-increment. */ increments = MAXALIGN_DOWN((int)avgTupleSize) / 32; crossover = 0.90 - (increments * 0.075); Most cases won't get too many "increments" of credit (although CLUSTER sorts will probably get relatively many). A third consideration is that we should be stingy about giving too much credit to wider tuples because the cache inefficiency hurts more as we achieve mere linear savings in I/O. So, most of the savings off a 99.99% theoretical baseline threshold are fixed (you usually save 9.99% off that up-front). A forth consideration is that the heap seems to do really badly past 1GB in general, due to cache characteristics. This is certainly not something that I know how to model well. I don't blame you for calling this voodoo, because to some extent it is. But I remind you that the consequences of making the wrong decision here are still better than the status quo today -- probably far better, overall. I also remind you that voodoo code is something you'll find in well regarded code bases at times. Have you ever written networking code? Packet switching is based on some handwavy observations about the real world. Practical implementations often contain voodoo magic numbers. So, to answer your earlier question: Yes, maybe it wouldn't be so bad, all things considered, to let someone complain about this if they have a real-world problem with it. The complexity of what we're talking about makes me modest about my ability to get it exactly right. At the same time, the consequences of getting it somewhat wrong are really not that bad. This is basically the same tension that you get with more rigorous cost models anyway (where greater rigor happens to be possible). I will abandon this cost model at the first sign of a better alternative -- I'm really not the least bit attached to it. I had hoped that we'd be able to do a bit better than this through discussion on list, but not far better. In any case, "quicksort with spillover" is of secondary importance here (even though it just so happens that I started with it). >>>> Another factor is that the heap could be useful for other stuff in the >>>> future. As Simon Riggs pointed out, for deduplicating values as >>>> they're read in by tuplesort. (Okay, that's really the only other >>>> thing, but it's a good one). >>> >>> Not sure how that would work? >> >> Tuplesort would have license to discard tuples with matching existing >> values, because the caller gave it permission to. This is something >> that you can easily imagine occurring with ordered set aggregates, for >> example. It would work in a way not unlike a top-N heapsort does >> today. This would work well when it can substantially lower the use of >> memory (initially heapification when the threshold is crossed would >> probably measure the number of duplicates, and proceed only when it >> looked like a promising strategy). > > It's not clear to me how having a heap helps with that. The immediacy of detecting a duplicate could be valuable. We could avoid allocating tuplesort-owned memory entirely much of the time. Basically, this is another example (quicksort with spillover being the first) where incrementalism helps rather than hurts. Another consideration is that we could thrash if we misjudge the frequency at which to eliminate duplicates if we quicksort + periodically dedup. This is especially of concern in the common case where there are big clusters of the same value, and big clusters of heterogeneous values. -- Peter Geoghegan
On Thu, Dec 24, 2015 at 8:44 AM, Peter Geoghegan <pg@heroku.com> wrote: > [long blahblah] (Patch moved to next CF, work is going on. Thanks to people here to be active) -- Michael
On Wed, Dec 23, 2015 at 1:03 PM, Jeff Janes <jeff.janes@gmail.com> wrote: > The regression I found when building an index on a column of > 400,000,000 md5(random()::text) with 64MB maintenance_work_mem was not > hard to find at all. I still don't understand what is going on with > it, but it is reproducible. Perhaps it is very unlikely and I just > got very lucky in finding it immediately after switching to that > data-type for my tests, but I wouldn't assume that on current > evidence. Well, that is a lot of tuples to sort with such a small amount of memory. I have a new theory. Maybe part of the problem here is that in very low memory conditions, the tape overhead really is kind of wasteful, and we're back to having to worry about per-tape overhead (6 tapes may have been far too miserly as a universal number back before that was fixed [1], but that doesn't mean that the per-tape overhead is literally zero). You get a kind of thrashing, perhaps. Also, more tapes results in more random I/O, and that's an added cost, too; the cure may be worse than the disease. I also think that this might be a problem in your case: * In this calculation we assume that each tape will cost us about 3 blocks * worth of buffer space (which is an underestimate for very large data * volumes, but it's probably close enough --- see logtape.c). I wonder, what's the situation here like with the attached patch applied on top of what you were testing? I think that we might be better off with more merge steps when under enormous memory pressure at the low end, in order to be able to store more tuples per tape (and do more sorting using quicksort). I also think that under conditions such as you describe, this code may play havoc with memory accounting: /* * Decrease availMem to reflect the space needed for tape buffers; but * don't decrease it to the point that we have no room for tuples. (That * case is only likely to occur if sorting pass-by-value Datums; in all * other scenarios the memtuples[] array is unlikely to occupy more than * half of allowedMem. In the pass-by-value case it's not important to * account for tuple space, so we don't care if LACKMEM becomes * inaccurate.) */ tapeSpace = (int64) maxTapes *TAPE_BUFFER_OVERHEAD; if (tapeSpace + GetMemoryChunkSpace(state->memtuples) < state->allowedMem) USEMEM(state, tapeSpace); Remember, this is after the final grow_memtuples() call that uses your intelligent resizing logic [2], so we'll USEMEM() in a way that effectively makes some non-trivial proportion of our optimal memtuples sizing unusable. Again, that could be really bad for cases like yours, with very little memory relatively to data volume. Thanks [1] Commit df700e6b4 [2] Commit 8ae35e918 -- Peter Geoghegan
Attachment
On Wed, Dec 23, 2015 at 7:48 PM, Peter Geoghegan <pg@heroku.com> wrote: > I wonder, what's the situation here like with the attached patch > applied on top of what you were testing? I think that we might be > better off with more merge steps when under enormous memory pressure > at the low end, in order to be able to store more tuples per tape (and > do more sorting using quicksort). Actually, now that I look into it, I think your 64MB work_mem setting would have 234 tapes in total, so my patch won't do anything for your case. Maybe change MAXORDER to 100 within the patch, to see where that leaves things? I want to see if there is any improvement. 234 tapes means that approximately 5.7MB of memory would go to just using tapes (for accounting purposes, which is mostly my concern here). However, for a case like this, where you're well short of being able to do everything in one pass, there is no benefit to having more than about 6 tapes (I guess that's probably still true these days). That 5.7MB of tape space for accounting purposes (and also in reality) may not only increase the amount of random I/O required, and not only throw off the memtuples estimate within grow_memtuples() (its balance against everything else), but also decrease the cache efficiency in the final on-the-fly merge (the efficiency in accessing tuples). -- Peter Geoghegan
On Fri, Dec 18, 2015 at 11:57 AM, Peter Geoghegan <pg@heroku.com> wrote: > BTW, I'm not necessarily determined to make the new special-purpose > allocator work exactly as proposed. It seemed useful to prioritize > simplicity, and currently so there is one big "huge palloc()" with > which we blow our memory budget, and that's it. However, I could > probably be more clever about "freeing ranges" initially preserved for > a now-exhausted tape. That kind of thing. Attached is a revision that significantly overhauls the memory patch, with several smaller changes. We can now grow memtuples to rebalance the size of the array (memtupsize) against the need for memory for tuples. Doing this makes a big difference with a 500MB work_mem setting in this datum sort case, as my newly expanded trace_sort instrumentation shows: LOG: grew memtuples 1.40x from 9362286 (219429 KB) to 13107200 (307200 KB) for final merge LOG: tape 0 initially used 34110 KB of 34110 KB batch (1.000) and 13107200 slots remaining LOG: tape 1 initially used 34110 KB of 34110 KB batch (1.000) and has 1534 slots remaining LOG: tape 2 initially used 34110 KB of 34110 KB batch (1.000) and has 1535 slots remaining LOG: tape 3 initially used 34110 KB of 34110 KB batch (1.000) and has 1533 slots remaining LOG: tape 4 initially used 34110 KB of 34110 KB batch (1.000) and has 1534 slots remaining LOG: tape 5 initially used 34110 KB of 34110 KB batch (1.000) and has 1535 slots remaining This is a big improvement. With the new batchmemtuples() call commented out (i.e. no new grow_memtuples() call), the LOG output around the same point is: LOG: tape 0 initially used 24381 KB of 48738 KB batch (0.500) and has 1 slots remaining LOG: tape 1 initially used 24381 KB of 48738 KB batch (0.500) and has 1 slots remaining LOG: tape 2 initially used 24381 KB of 48738 KB batch (0.500) and has 1 slots remaining LOG: tape 3 initially used 24381 KB of 48738 KB batch (0.500) and has 1 slots remaining LOG: tape 4 initially used 24381 KB of 48738 KB batch (0.500) and has 1 slots remaining LOG: tape 5 initially used 24381 KB of 48738 KB batch (0.500) and has 1 slots remaining (I actually added a bit more detail to what you see here during final clean-up) Obviously we're using memory a lot more efficiently here as compared to my last revision (or the master branch -- it always has palloc() overhead, of course). With no grow_memtuples, we're not wasting ~1530 slots per tape anymore (which is a tiny fraction of 1% of the total), but we are wasting 50% of all batch memory, or almost 30% of all work_mem. Note that this improvement is possible despite the fact that memory is still MAXALIGN()'d -- I'm mostly just clawing back what I can, having avoided much STANDARDCHUNKHEADERSIZE overhead for the final on-the-fly merge. I tend to think that the bigger problem here is that we use so many memtuples when merging in the first place though (e.g. 60% in the above case), because memtuples are much less useful than something like a simple array of pointers when merging; I can certainly see why you'd need 6 memtuples here, for the merge heap, but the other ~13 million seem mostly unnecessary. Anyway, what I have now is as far as I want to go to accelerate merging for 9.6, since parallel CREATE INDEX is where the next big win will come from. As wasteful as this can be, I think it's of secondary importance. With this revision, I've given up on the idea of trying to map USEMEM()/FREEMEM() to "logical" allocations and deallocations that consume from each tape's batch. The existing merge code in the master branch is concerned exclusively with making each tape's use of memory fair; each tape only gets so many "slots" (memtuples), and so much memory, and that's it (there is never any shuffling of those resource budgets between tapes). I get the same outcome from simply only allowing tapes to get memory from their own batch allocation, which isn't much complexity, because only READTUP() routines regularly need memory. We detect when memory has been exhausted within mergeprereadone() in a special way, not using LACKMEM() at all -- this seems simpler. (Specifically, we use something called overflow allocations for this purpose. This means that there are still a very limited number of retail palloc() calls.) This new version somewhat formalizes the idea that batch allocation may one day have uses beyond the final on-the-fly merge phase, which makes a lot of sense. We should really be saving a significant amount of memory when initially sorting runs, too. This revision also pfree()s tape memory early if the tape is exhausted early, which will help a lot when there is a logical/physical correlation. Overall, I'm far happier with how memory is managed in this revision, mostly because it's easier to reason about. trace_sort now closely monitors where memory goes, and I think that's a good idea in general. That makes production performance problems a lot easier to reason about -- the accounting should be available to expert users (that enable trace_sort). I'll have little sympathy for the suggestion that this will overwhelm users, because trace_sort is already only suitable for experts. Besides, it isn't that complicated to figure this stuff out, or at least gain an intuition for what might be going on based on differences seen in a problematic case. Getting a better picture of what "bad" looks like can guide an investigation without the DBA necessarily understanding the underlying algorithms. At worst, it gives them something specific to complain about here. Other changes: * No longer use "tuple proper" terminology. Also, memory pools are now referred to as batch memory allocations. This is at the request of Jeff and Robert. * Fixed silly bug in useselection() cost model that causes "quicksort with spillover" to never be used. The cost model is otherwise unchanged, because I didn't come up with any bright ideas about how to do better there. Ideas from other people are very much welcome. * Cap the maximum number of tapes to 500. I think it's silly that the number of tapes is currently a function of work_mem, without any further consideration of the details of the sort, but capping is a simpler solution than making tuplesort_merge_order() smarter. I previously saw quite a lot of waste with high work_mem settings, with tens of thousands of tapes that will never be used, precisely because we have lots of memory (the justification for having, say, 40k tapes seems to be almost an oxymoron). Tapes (or the accounting for never-allocated tapes) could take almost 10% of all memory. Also, less importantly, we now refund/FREEMEM() unallocated tape memory ahead of final on-the-fly merge preallocation of batch memory. Note that we contemplated bounding the number of tapes in the past several times. See the commit message of c65ab0bfa9, a commit from almost a decade ago, for an example of this. That message also describes how "slots" (memtuples) and memory for tuples must be kept in balance while merging, which is very much relevant to my new grow_memtuples() call. -- Peter Geoghegan
Attachment
On Wed, Dec 23, 2015 at 9:37 AM, Robert Haas <robertmhaas@gmail.com> wrote: >> But yes, let me concede more clearly: the cost model is based on >> frobbing. But at least it's relatively honest about that, and is >> relatively simple. I think it might be possible to make it simpler, >> but I have a feeling that anything we can come up with will basically >> have the same quality that you so dislike. I don't know how to do >> better. Frankly, I'd rather be roughly correct than exactly wrong. > > Sure, but the fact that the model has huge discontinuities - perhaps > most notably a case where adding a single tuple to the estimated > cardinality changes the crossover point by a factor of two - suggests > that you are probably wrong. The actual behavior does not change > sharply when the size of the SortTuple array crosses 1GB, but the > estimates do. Here is some fairly interesting analysis of Quicksort vs. Heapsort, from Bentley, coauthor of our own Quicksort implementation: https://youtu.be/QvgYAQzg1z8?t=16m15s (This link picks up at the right point to see the comparison, complete with an interesting graph). It probably doesn't tell you much that you didn't already know, at least at this exact point, but it's nice to see Bentley's graph. This perhaps gives you some idea of why my "quicksort with spillover" cost model had a cap of MaxAllocSize of SortTuples, past which we always needed a very compelling case. That was my rough guess of where the Heapsort graph takes a sharp upward turn. Before then, Bentley shows that it's close enough to a straight line. Correct me if I'm wrong, but I think that the only outstanding issue with all patches posted here so far is the "quicksort with spillover" cost model. Hopefully this can be cleared up soon. As I've said, I am very receptive to other people's suggestions about how that should work. -- Peter Geoghegan
On Tue, Dec 29, 2015 at 4:33 AM, Peter Geoghegan <pg@heroku.com> wrote:
>Attached is a revision that significantly overhauls the memory patch,
>with several smaller changes.
>with several smaller changes.
I just ran some tests on above patch. Mainly to compare
how "longer sort keys" would behave with new(Qsort) and old Algo(RS) for sorting.
I have 8GB of ram and ssd storage.
Settings and Results.
----------------------------
Work_mem= DEFAULT (4mb).
key width = 520.
CASE 1. Data is pre-sorted as per sort key order.
CASE 2. Data is sorted in opposite order of sort key.
CASE 3. Data is randomly distributed.
Key length 520 | ||||
Number of records | 3200000 | 6400000 | 12800000 | 25600000 |
1.7 GB | 3.5GB | 7 GB | 14GB | |
CASE 1 | ||||
RS | 23654.677 | 35172.811 | 44965.442 | 106420.155 |
Qsort | 14100.362 | 40612.829 | 101068.107 | 334893.391 |
CASE 2 | ||||
RS | 13427.378 | 36882.898 | 98492.644 | 310670.15 |
Qsort | 12475.133 | 32559.074 | 100772.531 | 322080.602 |
CASE 3 | ||||
RS | 17202.966 | 45163.234 | 122323.299 | 337058.856 |
Qsort | 12530.726 | 23343.753 | 59431.315 | 152862.837 |
If data is sorted as same as sort key order then current code performs better than proposed patch
as sort size increases.
It appears new algo do not seem have any major impact if rows are presorted in opposite order.
For randomly distributed order quick sort performs well when compared to current sort method (RS).
======================================================
Now Increase the work_mem to 64MB and for 14 GB of data to sort.
CASE 1: We can see Qsort is able to catchup with current sort method(RS).
CASE 2: No impact.
CASE 3: RS is able to catchup with Qsort.
CASE 1 | RS | 128822.735 |
Qsort | 90857.496 |
CSAE 2 | RS | 105631.775 |
Qsort | 105938.334 |
CASE 3 | RS | 152301.054 |
Qsort | 149649.347 |
I think for long keys both old (RS) and new (Qsort) sort method has its own characteristics
based on data distribution. I think work_mem is the key If properly set new method(Qsort) will
be able to fit most of the cases. If work_mem is not tuned right it, there are cases it can regress.
Attachment
On Fri, Jan 29, 2016 at 5:11 PM, Mithun Cy <mithun.cy@enterprisedb.com> wrote
>I just ran some tests on above patch. Mainly to compare>how "longer sort keys" would behave with new(Qsort) and old Algo(RS) for sorting.>I have 8GB of ram and ssd storage.
Key length 520
Number of records3200000 6400000 12800000 25600000 1.7 GB 3.5GB 7 GB 14GB CASE 1 RS 23654.677 35172.811 44965.442 106420.155 Qsort 14100.362 40612.829 101068.107 334893.391 CASE 2 RS 13427.378 36882.898 98492.644 310670.15 Qsort 12475.133 32559.074 100772.531 322080.602 CASE 3 RS 17202.966 45163.234 122323.299 337058.856 Qsort 12530.726 23343.753 59431.315 152862.837
CASE 1 RS 128822.735 Qsort 90857.496
CSAE 2 RS 105631.775 Qsort 105938.334
CASE 3 RS 152301.054 Qsort 149649.347
Sorry forgot to mention above data in table is in unit of ms, returned by psql client.
--
On Fri, Jan 29, 2016 at 3:41 AM, Mithun Cy <mithun.cy@enterprisedb.com> wrote: > I just ran some tests on above patch. Mainly to compare > how "longer sort keys" would behave with new(Qsort) and old Algo(RS) for sorting. > I have 8GB of ram and ssd storage. > > Settings and Results. > ---------------------------- > Work_mem= DEFAULT (4mb). > key width = 520. > If data is sorted as same as sort key order then current code performs better than proposed patch > as sort size increases. > > It appears new algo do not seem have any major impact if rows are presorted in opposite order. > > For randomly distributed order quick sort performs well when compared to current sort method (RS). > > > ====================================================== > Now Increase the work_mem to 64MB and for 14 GB of data to sort. > > CASE 1: We can see Qsort is able to catchup with current sort method(RS). > CASE 2: No impact. > CASE 3: RS is able to catchup with Qsort. I think that the basic method you're using to do these tests may have additional overhead: -- sort in ascending order. CREATE FUNCTION test_orderby_asc( ) RETURNS int AS $$ #print_strict_params on DECLARE gs int; jk text; BEGIN SELECT string_4k, generate_series INTO jk, gs FROM so order by string_4k, generate_series; RETURN gs; END $$ LANGUAGE plpgsql; Anyway, these test cases all remove much of the advantage of increased cache efficiency. No comparisons are *ever* resolved using the leading attribute, which calls into question why anyone would sort on that. It's 512 bytes, so artificially makes the comparisons themselves the bottleneck, as opposed to cache efficiency. You can't even fit the second attribute in the same cacheline as the first in the "tuple proper" (MinimalTuple). You are using a 4MB work_mem setting, but you almost certainly have a CPU with an L3 cache size that's a multiple of that, even with cheap consumer grade hardware. You have 8GB of ram; a 4MB work_mem setting is very small setting (I mean in an absolute sense, less so than relative to the size of data, although especially relative to the data). You mentioned "CASE 3: RS is able to catchup with Qsort", which doesn't make much sense to me. The only way I think that is possible is by making the increased work_mem sufficient to have much longer runs, because there is in fact somewhat of a correlation in the data, and an increased work_mem makes the critical difference, allowing perhaps one long run to be used -- there is now enough memory to "juggle" tuples without ever needing to start a new run. But, how could that be? You said case 3 was totally random data, so I'd only expect incremental improvement. It could also be some weird effect from polyphase merge. A discontinuity. I also don't understand why the patch ("Qsort") can be so much slower between case 1 and case 3 on 3.5GB+ sizes, but not the 1.7GB size. Even leaving aside the differences between "RS" and "Qsort", it makes no sense to me that *both* are faster with random data ("CASE 3") than with presorted data ("CASE 1"). Another weird thing is that the traditional best case for replacement selection ("RS") is a strong correlation, and a traditional worst case is an inverse correlation, where run size is bound strictly by memory. But you show just the opposite here -- the inverse correlation is faster with RS in the 1.7 GB data case. So, I have no idea what's going on here, and find it all very confusing. In order for these numbers to be useful, they need more detail -- "trace_sort" output. There are enough confounding factors in general, and especially here, that not having that information makes raw numbers very difficult to interpret. > I think for long keys both old (RS) and new (Qsort) sort method has its own characteristics > based on data distribution. I think work_mem is the key If properly set new method(Qsort) will > be able to fit most of the cases. If work_mem is not tuned right it, there are cases it can regress. work_mem is impossible to tune right with replacement selection. That's a key advantage of the proposed new approach. -- Peter Geoghegan
On Wed, Jan 27, 2016 at 8:20 AM, Peter Geoghegan <pg@heroku.com> wrote: > Correct me if I'm wrong, but I think that the only outstanding issue > with all patches posted here so far is the "quicksort with spillover" > cost model. Hopefully this can be cleared up soon. As I've said, I am > very receptive to other people's suggestions about how that should > work. I feel like this could be data driven. I mean, the cost model is based mainly on the tuple width and the size of the SortTuple array. So, it should be possible to tests of both algorithms on 32, 64, 96, 128, ... byte tuples with a SortTuple array that is 256MB, 512MB, 768MB, 1GB, ... Then we can judge how closely the cost model comes to mimicking the actual behavior. -- Robert Haas EnterpriseDB: http://www.enterprisedb.com The Enterprise PostgreSQL Company
On Fri, Jan 29, 2016 at 9:24 AM, Robert Haas <robertmhaas@gmail.com> wrote: > I feel like this could be data driven. I mean, the cost model is > based mainly on the tuple width and the size of the SortTuple array. > So, it should be possible to tests of both algorithms on 32, 64, 96, > 128, ... byte tuples with a SortTuple array that is 256MB, 512MB, > 768MB, 1GB, ... Then we can judge how closely the cost model comes to > mimicking the actual behavior. You would also need to represent how much of the input actually ended up being sorted with the heap in each case. Maybe that could be tested at 50% (bad for "quicksort with spillover"), 25% (better), and 5% (good). An alternative approach that might be acceptable is to add a generic, conservative 90% threshold (so 10% of tuples sorted by heap). -- Peter Geoghegan
On Fri, Jan 29, 2016 at 12:46 PM, Peter Geoghegan <pg@heroku.com> wrote: > On Fri, Jan 29, 2016 at 9:24 AM, Robert Haas <robertmhaas@gmail.com> wrote: >> I feel like this could be data driven. I mean, the cost model is >> based mainly on the tuple width and the size of the SortTuple array. >> So, it should be possible to tests of both algorithms on 32, 64, 96, >> 128, ... byte tuples with a SortTuple array that is 256MB, 512MB, >> 768MB, 1GB, ... Then we can judge how closely the cost model comes to >> mimicking the actual behavior. > > You would also need to represent how much of the input actually ended > up being sorted with the heap in each case. Maybe that could be tested > at 50% (bad for "quicksort with spillover"), 25% (better), and 5% > (good). > > An alternative approach that might be acceptable is to add a generic, > conservative 90% threshold (so 10% of tuples sorted by heap). I don't quite know what you mean by these numbers. Add a generic, conservative threshold to what? Thinking about this some more, I really think we should think hard about going back to the strategy which you proposed and discarded in your original post: always generate the first run using replacement selection, and every subsequent run by quicksorting. In that post you mention powerful advantages of this method: "even without a strong logical/physical correlation, the algorithm tends to produce runs that are about twice the size of work_mem. (It's also notable that replacement selection only produces one run with mostly presorted input, even where input far exceeds work_mem, which is a neat trick.)"You went on to dismiss that strategy, saying that "despitethese upsides, replacement selection is obsolete, and should usually be avoided." But I don't see that you've justified that statement. It seems pretty easy to construct cases where this technique regresses, and a large percentage of those cases are precisely those where replacement selection would have produced a single run, avoiding the merge step altogether. I think those cases are extremely important. I'm quite willing to run somewhat more slowly than in other cases to be certain of not regressing the case of completely or almost-completely ordered input. Even if that didn't seem like a sufficient reason unto itself, I'd be willing to go that way just so we don't have to depend on a cost model that might easily go wrong due to bad input even if it were theoretically perfect in every other respect (which I'm pretty sure is not true here anyway). I also have another idea that might help squeeze more performance out of your approach and avoid regressions. Suppose that we add a new GUC with a name like sort_mem_stretch_multiplier or something like that, with a default value of 2.0 or 4.0 or whatever we think is reasonable. When we've written enough runs that a polyphase merge will be required, or when we're actually performing a polyphase merge, the amount of memory we're allowed to use increases by this multiple. The idea is: we hope that people will set work_mem appropriately and consequently won't experience polyphase merges at all, but it might. However, it's almost certain not to happen very frequently. Therefore, using extra memory in such cases should be acceptable, because while you might have every backend in the system using 1 or more copies of work_mem for something if the system is very busy, it is extremely unlikely that you will have more than a handful of processes doing polyphase merges. -- Robert Haas EnterpriseDB: http://www.enterprisedb.com The Enterprise PostgreSQL Company
On Fri, Jan 29, 2016 at 2:58 PM, Robert Haas <robertmhaas@gmail.com> wrote: > I don't quite know what you mean by these numbers. Add a generic, > conservative threshold to what? I meant use "quicksort with spillover" simply because an estimated 90%+ of all tuples have already been consumed. Don't consider the tuple width, etc. > Thinking about this some more, I really think we should think hard > about going back to the strategy which you proposed and discarded in > your original post: always generate the first run using replacement > selection, and every subsequent run by quicksorting. In that post you > mention powerful advantages of this method: "even without a strong > logical/physical correlation, the algorithm tends to produce runs that > are about twice the size of work_mem. (It's also notable that > replacement selection only produces one run with mostly presorted > input, even where input far exceeds work_mem, which is a neat trick.)" > You went on to dismiss that strategy, saying that "despite these > upsides, replacement selection is obsolete, and should usually be > avoided." But I don't see that you've justified that statement. Really? Just try it with a heap that is not tiny. Performance tanks. The fact that replacement selection can produce one long run then becomes a liability, not a strength. With a work_mem of something like 1GB, it's *extremely* painful. > It seems pretty easy to construct cases where this technique regresses, > and a large percentage of those cases are precisely those where > replacement selection would have produced a single run, avoiding the > merge step altogether. ...*and* where many passes are otherwise required (otherwise, the merge is still cheap enough to leave us ahead). Typically with very small work_mem settings, like 4MB, and far larger data volumes. It's easy to construct those cases, but that doesn't mean that they particularly matter. Using 4MB of work_mem to sort 10GB of data is penny wise and pound foolish. The cases we've seen regressed are mostly a concern because misconfiguration happens. A compromise that may be acceptable is to always do a "quicksort with spillover" when there is a very low work_mem setting and the estimate of the number of input tuples is less than 10x of what we've seen so far. Maybe less than 20MB. That will achieve the same thing. > I'm quite willing to run somewhat more slowly than in other cases to > be certain of not regressing the case of completely or > almost-completely ordered input. Even if that didn't seem like a > sufficient reason unto itself, I'd be willing to go that way just so > we don't have to depend on a cost model that might easily go wrong due > to bad input even if it were theoretically perfect in every other > respect (which I'm pretty sure is not true here anyway). The consequences of being wrong either way are not severe (note that making one long run isn't a goal of the cost model currently). > I also have another idea that might help squeeze more performance out > of your approach and avoid regressions. Suppose that we add a new GUC > with a name like sort_mem_stretch_multiplier or something like that, > with a default value of 2.0 or 4.0 or whatever we think is reasonable. > When we've written enough runs that a polyphase merge will be > required, or when we're actually performing a polyphase merge, the > amount of memory we're allowed to use increases by this multiple. The > idea is: we hope that people will set work_mem appropriately and > consequently won't experience polyphase merges at all, but it might. > However, it's almost certain not to happen very frequently. > Therefore, using extra memory in such cases should be acceptable, > because while you might have every backend in the system using 1 or > more copies of work_mem for something if the system is very busy, it > is extremely unlikely that you will have more than a handful of > processes doing polyphase merges. I'm not sure that that's practical. Currently, tuplesort decides on a number of tapes ahead of time. When we're constrained on those, the stretch multiplier would apply, but I think that that could be invasive because the number of tapes ("merge order" + 1) was a function of non-stretched work_mem. -- Peter Geoghegan
<p dir="ltr"><br /> On 29 Jan 2016 11:58 pm, "Robert Haas" <<a href="mailto:robertmhaas@gmail.com">robertmhaas@gmail.com</a>>wrote:<br /> > It<br /> > seems pretty easy to constructcases where this technique regresses,<br /> > and a large percentage of those cases are precisely those where<br/> > replacement selection would have produced a single run, avoiding the<br /> > merge step altogether. <pdir="ltr">Now that avoiding the merge phase altogether didn't necessarily represent any actual advantage.<p dir="ltr">Wedon't find out we've avoided the merge phase until the entire run has been spiked to disk. Then we need to readit back in from disk to serve up those tuples.<p dir="ltr">If we have tapes to merge but can do then in a single passwe do that lazily and merge as needed when we serve up the tuples. I doubt there's any speed difference in reading twosequential streams with our buffering over one especially in the midst of a quiet doing other i/o. And N extra comparisonsis less than the quicksort advantage.<p dir="ltr">If we could somehow predict that it'll be a single output runthat would be a huge advantage. But having to spill all the tuples and then find out isn't really helpful.
<p dir="ltr"><br /> On 30 Jan 2016 8:27 am, "Greg Stark" <<a href="mailto:stark@mit.edu">stark@mit.edu</a>> wrote:<br/> ><br /> ><br /> > On 29 Jan 2016 11:58 pm, "Robert Haas" <<a href="mailto:robertmhaas@gmail.com">robertmhaas@gmail.com</a>>wrote:<br /> > > It<br /> > > seems pretty easyto construct cases where this technique regresses,<br /> > > and a large percentage of those cases are preciselythose where<br /> > > replacement selection would have produced a single run, avoiding the<br /> > >merge step altogether. <br /> ><br /> > Now that avoiding the merge phase altogether didn't necessarily representany actual advantage.<br /> ><br /> > We don't find out we've avoided the merge phase until the entire runhas been spiked to disk. <p dir="ltr">Hm, sorry about the phone typos. I thought I proofread it as I went but obviouslynot that effectively...
On Sat, Jan 30, 2016 at 2:25 AM, Peter Geoghegan <pg@heroku.com> wrote: > On Fri, Jan 29, 2016 at 2:58 PM, Robert Haas <robertmhaas@gmail.com> wrote: >> I don't quite know what you mean by these numbers. Add a generic, >> conservative threshold to what? > > I meant use "quicksort with spillover" simply because an estimated > 90%+ of all tuples have already been consumed. Don't consider the > tuple width, etc. Hmm, it's a thought. >> Thinking about this some more, I really think we should think hard >> about going back to the strategy which you proposed and discarded in >> your original post: always generate the first run using replacement >> selection, and every subsequent run by quicksorting. In that post you >> mention powerful advantages of this method: "even without a strong >> logical/physical correlation, the algorithm tends to produce runs that >> are about twice the size of work_mem. (It's also notable that >> replacement selection only produces one run with mostly presorted >> input, even where input far exceeds work_mem, which is a neat trick.)" >> You went on to dismiss that strategy, saying that "despite these >> upsides, replacement selection is obsolete, and should usually be >> avoided." But I don't see that you've justified that statement. > > Really? Just try it with a heap that is not tiny. Performance tanks. > The fact that replacement selection can produce one long run then > becomes a liability, not a strength. With a work_mem of something like > 1GB, it's *extremely* painful. I'm not sure exactly what you think I should try. I think a couple of people have expressed the concern that your patch might regress things on data that is all in order, but I'm not sure if you think I should try that case or some case that is not-quite-in-order. "I don't see that you've justified that statement" is referring to the fact that you presented no evidence in your original post that it's important to sometimes use quicksorting even for run #1. If you've provided some test data illustrating that point somewhere, I'd appreciate a pointer back to it. > A compromise that may be acceptable is to always do a "quicksort with > spillover" when there is a very low work_mem setting and the estimate > of the number of input tuples is less than 10x of what we've seen so > far. Maybe less than 20MB. That will achieve the same thing. How about always starting with replacement selection, but limiting the amount of memory that can be used with replacement selection to some small value? It could be a separate GUC, or a hard-coded constant like 20MB if we're fairly confident that the same value will be good for everyone. If the tuples aren't in order, then we'll pretty quickly come to the end of the first run and switch to quicksort. If we do end up using replacement selection for the whole sort, the smaller heap is an advantage. What I like about this sort of thing is that it adds no reliance on any estimate; it's fully self-tuning. -- Robert Haas EnterpriseDB: http://www.enterprisedb.com The Enterprise PostgreSQL Company
On Sat, Jan 30, 2016 at 5:29 AM, Robert Haas <robertmhaas@gmail.com> wrote: >> I meant use "quicksort with spillover" simply because an estimated >> 90%+ of all tuples have already been consumed. Don't consider the >> tuple width, etc. > > Hmm, it's a thought. To be honest, it's a bit annoying that this is one issue we're stuck on, because "quicksort with spillover" is clearly of less importance overall. (This is a distinct issue from the issue of not using a replacement selection style heap for the first run much of the time, which seems to be a discussion about whether and to what extent the *traditional* advantages of replacement selection hold today, as opposed to a discussion about a very specific crossover point in my patch.) >> Really? Just try it with a heap that is not tiny. Performance tanks. >> The fact that replacement selection can produce one long run then >> becomes a liability, not a strength. With a work_mem of something like >> 1GB, it's *extremely* painful. > > I'm not sure exactly what you think I should try. I think a couple of > people have expressed the concern that your patch might regress things > on data that is all in order, but I'm not sure if you think I should > try that case or some case that is not-quite-in-order. "I don't see > that you've justified that statement" is referring to the fact that > you presented no evidence in your original post that it's important to > sometimes use quicksorting even for run #1. If you've provided some > test data illustrating that point somewhere, I'd appreciate a pointer > back to it. I think that the answer to what you should try is simple: Any case involving a large heap (say, a work_mem of 1GB). No other factor like correlation seems to change the conclusion about that being generally bad. If you have a correlation, then that is *worse* if "quicksort with spillover" always has us use a heap for the first run, because it prolongs the pain of using the cache inefficient heap (note that this is an observation about "quicksort with spillover" in particular, and not replacement selection in general). The problem you'll see is that there is a large heap which is __slow__ to spill from, and that's pretty obvious with or without a correlation. In general it seems unlikely that having one long run during the merge (i.e. no merge -- seen by having the heap build one long run because we got "lucky" and "quicksort with spillover" encountered a correlation) can ever hope to make up for this. It *could* still make up for it if: 1. There isn't much to make up for in the first place, because the heap is CPU cache resident. Testing this with a work_mem that is the same size as CPU L3 cache seems a bit pointless to me, and I think we've seen that a few times. and: 2. There are many passes required without a replacement selection heap, because the volume of data is just so much greater than the low work_mem setting. Replacement selection makes the critical difference because there is a correlation, perhaps strong enough to make it one or two runs rather than, say, 10 or 20 or 100. I've already mentioned many times that linear growth in the size of work_mem sharply reduces the need for additional passes during the merge phase (the observation about quadratic growth that I won't repeat). These days, it's hard to recommend anything other than "use more memory" to someone trying to use 4MB to sort 10GB of data. Yeah, it would also be faster to use replacement selection for the first run in the hope of getting lucky (actually lucky this time; no quotes), but it's hard to imagine that that's going to be a better option, no matter how frugal the user is. Helping users recognize when they could use more memory effectively seems like the best strategy. That was the idea behind multipass_warning, but you didn't like that (Greg Stark was won over on the multipass_warning warning, though). I hope we can offer something roughly like that at some point (a view?), because it makes sense. > How about always starting with replacement selection, but limiting the > amount of memory that can be used with replacement selection to some > small value? It could be a separate GUC, or a hard-coded constant > like 20MB if we're fairly confident that the same value will be good > for everyone. If the tuples aren't in order, then we'll pretty > quickly come to the end of the first run and switch to quicksort. This seems acceptable, although note that we don't have to decide until we reach the work_mem limit, and not before. If you want to use a heap for the first run, I'm not excited about the idea, but if you insist then I'm glad that you at least propose to limit it to the kind of cases that we *actually* saw regressed (i.e. low work_mem settings -- like the default work_mem setting, 4MB). We've seen no actual case with a larger work_mem that is advantaged by using a heap, even *with* a strong correlation (this is actually *worst of all*); that's where I am determined to avoid using a heap automatically. It wasn't my original insight that replacement selection has become all but obsolete. It took me a while to come around to that point of view. One 2014 SIGMOD paper says of replacement selection sort: "Finally, there has been very little interest in replacement selection sort and its variants over the last 15 years. This is easy to understand when one considers that the previous goal of replacement selection sort was to reduce the number of external memory passes to 2." > If we do end up using replacement selection for the whole sort, the > smaller heap is an advantage. What I like about this sort of thing is > that it adds no reliance on any estimate; it's fully self-tuning. Fine, but the point of "quicksort with spillover" is that it avoids I/O entirely. I'm not promoting it as useful for any of the reasons that replacement selection was traditionally useful (on 1970s hardware). So, we aren't much closer to working out a better cost model for "quicksort with spillover" (I guess you weren't really talking about that, though), an annoying sticking point (as already mentioned). -- Peter Geoghegan
On Thu, Feb 4, 2016 at 1:46 AM, Peter Geoghegan <pg@heroku.com> wrote: > It wasn't my original insight that replacement selection has become > all but obsolete. It took me a while to come around to that point of > view. Nyberg et al may have said it best in 1994, in the Alphasort Paper [1]: "By comparison, OpenVMS sort uses a pure replacement-selection sort to generate runs (Knuth, 1973). Replacement-selection is best for a memory-constrained environment. On average, replacement-selection generates runs that are twice as large as available memory, while the QuickSort runs are typically less than half of available memory. However, in a memory-rich environment, QuickSort is faster because it is simpler, makes fewer exchanges on average, and has superior address locality to exploit processor caching. " (I believe that the authors state that "QuickSort runs are typically less than half of available memory" because of the use of explicit asynchronous I/O in each thread, which doesn't apply to us). The paper also has very good analysis of the economics of sorting: "Even for surprisingly large sorts, it is economical to perform the sort in one pass." Of course, memory capacities have scaled enormously in the 20 years since this analysis was performed, so the analysis applies even at the very low end these days. The high capacity memory system that they advocate to get a one pass sort (instead of having faster disks) had 100MB of memory, which is of course tiny by contemporary standards. If you pay Heroku $7 a month, you get a "Hobby Tier" database with 512MB of memory. The smallest EC2 instance size, the t2.nano, costs about $1.10 to run for one week, and has 0.5GB of memory. The economics of using 4MB or even 20MB to sort 10GB of data are already preposterously bad for everyone that runs a database server, no matter how budget conscious they may be. I can reluctantly accept that we need to still use a heap with very low work_mem settings to avoid the risk of a regression (in the event of a strong correlation) on general principle, but I'm well justified in proposing "just don't do that" as the best practical advice. I thought I had your agreement on that point, Robert; is that actually the case? [1] http://www.cs.berkeley.edu/~rxin/db-papers/alphasort.pdf -- Peter Geoghegan
On Thu, Feb 4, 2016 at 6:14 AM, Peter Geoghegan <pg@heroku.com> wrote: > The economics of using 4MB or even 20MB to sort 10GB of data are > already preposterously bad for everyone that runs a database server, > no matter how budget conscious they may be. I can reluctantly accept > that we need to still use a heap with very low work_mem settings to > avoid the risk of a regression (in the event of a strong correlation) > on general principle, but I'm well justified in proposing "just don't > do that" as the best practical advice. > > I thought I had your agreement on that point, Robert; is that actually the case? Peter and I spent a few hours talking on Skype this morning about this point and I believe we have agreed on an algorithm that I think will address all of my concerns and hopefully also be acceptable to him. Peter, please weigh in and let me know if I've gotten anything incorrect here or if you think of other concerns afterwards. The basic idea is that we will add a new GUC with a name like replacement_sort_mem that will have a default value in the range of 20-30MB; or possibly we will hardcode this value, but for purposes of this email I'm going to assume it's a GUC. If the value of work_mem or maintenance_work_mem, whichever applies, is smaller than the value of replacement_sort_mem, then the latter has no effect. However, if replacement_sort_mem is the smaller value, then the amount of memory that can be used for a heap with replacement selection is limited to replacement_sort_mem: we can use more memory than that in total for the sort, but the amount that can be used for a heap is restricted to that value. The way we do this is explained in more detail below. One thing I just thought of (after the call) is that it might be better for this GUC to be in units of tuples rather than in units of memory; it's not clear to me why the optimal heap size should be dependent on the tuple size, so we could have a threshold like 300,000 tuples or whatever. But that's a secondary issue and I might be wrong about it: the point is that in order to have a chance of winning, a heap used for replacement selection needs to be not very big at all by the standards of modern hardware, so the plan is to limit it to a size at which it may have a chance. Here's how that will work, assuming Peter and I understand each other: 1. We start reading the input data. If we reach the end of the input data before (maintenance_)work_mem is exhausted, then we can simply quicksort the data and we're done. This is no different than what we already do today. 2. If (maintenance_)work_mem fills up completely, we will quicksort all of the data we have in memory. We will then regard the tail end of that sorted data, in an amount governed by replacement_sort_mem, as a heap, and use it to perform replacement selection until no tuples remain for the current run. Meanwhile, the rest of the sorted data remains in memory untouched. Logically, we're constructing a run of tuples which is split between memory and disk: the head of the run (what fits in all of (maintenance_)work_mem except for replacement_sort_mem) is in memory, and the tail of the run is on disk. 3. If we reach the end of input before replacement selection runs out of tuples for the current run, and if it finds no tuples for the next run prior to that time, then we are done. All of the tuples form a single run and we can return the tuples in memory first followed by the tuples on disk. This case is highly likely to be a huge win over what we have today, because (a) some portion of the tuples were sorted via quicksort rather than heapsort and that's faster, (b) the tuples that were sorted using a heap were sorted using a small heap rather than a big one, and (c) we only wrote out the minimal number of tuples to tape instead of, as we would have done today, all of them. 4. If we reach this step, then replacement selection with a small heap wasn't able to sort the input in a single run. We have a bunch of sorted data in memory which is the head of the same run whose tail is already on disk; we now spill all of these tuples to disk. That leaves only the heapified tuples in memory. We just ignore the fact that they are a heap and treat them as unsorted. We repeatedly do the following: read tuples until work_mem is full, sort them, and dump the result to disk as a run. When all runs have been created, we merge runs just as we do today. This algorithm seems very likely to beat what we do today in practically all cases. The benchmarking Peter and others have already done shows that building runs with quicksort rather than replacement selection can often win even if the larger number of tapes requires a multi-pass merge. The only cases where it didn't seem to be a clear win involved data that was already in sorted order, or very close to it. But with this algorithm, presorted input is fine: we'll quicksort some of it (which is faster than replacement selection because quicksort checks for presorted input) and sort the rest with a *small* heap (which is faster than our current approach of sorting it with a big heap when the data is already in order). On top of that, we'll only write out the minimal amount of data to disk rather than all of it. So we should still win. On the other hand, if the data is out of order, then we will do only a little bit of replacement selection before switching over to building runs by quicksorting, which should also win. The worst case I was able to think of for this algorithm is an input stream that is larger than work_mem and almost sorted: the only exception is that the record that should be exactly in the middle is all the way at the end. In that case, today's code will use a large heap and will consequently produce only a single run. The algorithm above will end up producing two runs, the second containing only that one tuple. That means we're going to incur the additional cost of a merge pass. On the other hand, we're also going to have substantial savings to offset that - the building-runs stage will save by using quicksort for some of the data and a small heap for the rest. So the cost to merge the runs will be at least partially, maybe completely, offset by reduced time spent building them. Furthermore, Peter has got other improvements in the patch which also make merging faster, so if we don't buy enough building the runs to completely counterbalance the cost of the merge, well, we may still win for that reason. Even if not, this is so much faster overall that a regression in some sort of constructed worst case isn't really important. I feel that presorted input is a sufficiently common case that we should try hard not to regress it - but presorted input with the middle value moved to the end is not. We need to not be horrible in that case, but there's absolutely no reason to believe that we will be. We may even be faster, but we certainly shouldn't be abysmally slower. Doing it this way also avoids the need to have a cost model that makes decisions on how to sort based on the anticipated size of the input. I'm really very happy about that, because I feel that any such cost model, no matter how good, is a risk: estimation errors are not uncommon. Maybe a really sturdy cost model would be OK in the end, but not needing one is better. We don't need to fear burning a lot of time on replacement selection, because the heap is small - any significant amount of out-of-order data will cause us to switch to the main algorithm, which is building runs by quicksorting. The decision is made based on the actual data we see rather than any estimate. There's only one potentially tunable parameter - replacement_sort_mem - but it probably won't hurt you very much even if it's wrong by a factor of two - and there's no reason to believe that value is going to be very different on one machine than another. So this seems like it should be pretty robust. -- Robert Haas EnterpriseDB: http://www.enterprisedb.com The Enterprise PostgreSQL Company
On Fri, Feb 5, 2016 at 9:31 AM, Robert Haas <robertmhaas@gmail.com> wrote: > Peter, please weigh in and let me know if I've gotten anything > incorrect here or if you think of other concerns afterwards. Right. Let me give you the executive summary first: I continue to believe, following thinking about the matter in detail, that this is a sensible compromise, that weighs everyone's concerns. It is pretty close to a win-win. I just need you to confirm what I say here in turn, so we're sure that we understand each other perfectly. > The basic idea is that we will add a new GUC with a name like > replacement_sort_mem that will have a default value in the range of > 20-30MB; or possibly we will hardcode this value, but for purposes of > this email I'm going to assume it's a GUC. If the value of work_mem > or maintenance_work_mem, whichever applies, is smaller than the value > of replacement_sort_mem, then the latter has no effect. By "no effect", you must mean that we always use a heap for the entire first run (albeit for the tail, with a hybrid quicksort/heap approach), but still use quicksort for every subsequent run, when it's clearly established that we aren't going to get one huge run. Is that correct? It was my understanding, based on your emphasis on producing only a single run, as well as your recent remarks on this thread about the first run being special, that you are really only interested in the presorted case, where one run is produced. That is, you are basically not interested in preserving the general ability of replacement selection to double run size in the event of a uniform distribution. (That particular doubling property of replacement selection is now technically lost by virtue of using this new hybrid model *anyway*, although it will still make runs longer in general). You don't want to change the behavior of the current patch for the second or subsequent run; that should remain a quicksort, pure and simple. Do I have that right? BTW, parallel sort should probably never use a heap anyway (ISTM that that will almost certainly be based on external sorts in the end). A heap is not really compatible with the parallel heap scan model. > One thing I just thought of (after the call) is that it might be > better for this GUC to be in units of tuples rather than in units of > memory; it's not clear to me why the optimal heap size should be > dependent on the tuple size, so we could have a threshold like 300,000 > tuples or whatever. I think you're right that a number of tuples is the logical way to express the heap size (as a GUC unit). I think that the ideal setting for the GUC is large enough to recognize significant correlations in input data, which may be clustered, but no larger (at least while things don't all fit in L1 cache, or maybe L2 cache). We should "go for broke" with replacement selection -- we don't aim for anything less than ending up with 1 run by using the heap (merging 2 or 3 runs rather than 4 or 6 is far less useful, maybe harmful, when one of them is much larger). Therefore, I don't expect that we'll be practically disadvantaged by having fewer "hands to juggle" tuples here (we'll simply almost always have enough in practice -- more on that later). FWIW I don't think that any benchmark we've seen so far justifies doing less than "going for broke" with RS, even if you happen to have a very conservative perspective. One advantage of a GUC is that you can set it to zero, and always get a simple hybrid sort-merge strategy if that's desirable. I think that it might not matter much with multi-gigabyte work_mem settings anyway, though; you'll just see a small blip. Big (maintenance_)work_mem was by far my greatest concern in relation to using a heap in general, so I'm left pretty happy by this plan, I think. Lots of people can afford a multi-GB maintenance_work_mem these days, and CREATE INDEX is gonna be the most important case overall, by far. > 2. If (maintenance_)work_mem fills up completely, we will quicksort > all of the data we have in memory. We will then regard the tail end > of that sorted data, in an amount governed by replacement_sort_mem, as > a heap, and use it to perform replacement selection until no tuples > remain for the current run. Meanwhile, the rest of the sorted data > remains in memory untouched. Logically, we're constructing a run of > tuples which is split between memory and disk: the head of the run > (what fits in all of (maintenance_)work_mem except for > replacement_sort_mem) is in memory, and the tail of the run is on > disk. I went back and forth on this during our call, but I now think that I was right that there will need to be changes in order to make the tail of the run a heap (*not* the quicksorted head), because routines like tuplesort_heap_siftup() assume that state->memtuples[0] is the head of the heap. This is currently assumed by the master branch for both the currentRun/nextRun replacement selection heap, as well as the heap used for merging. Changing this is probably fairly manageable, though (probably still not going to use memmove() for this, contrary to my remarks on the call). > 3. If we reach the end of input before replacement selection runs out > of tuples for the current run, and if it finds no tuples for the next > run prior to that time, then we are done. All of the tuples form a > single run and we can return the tuples in memory first followed by > the tuples on disk. This case is highly likely to be a huge win over > what we have today, because (a) some portion of the tuples were sorted > via quicksort rather than heapsort and that's faster, (b) the tuples > that were sorted using a heap were sorted using a small heap rather > than a big one, and (c) we only wrote out the minimal number of tuples > to tape instead of, as we would have done today, all of them. Agreed. > 4. If we reach this step, then replacement selection with a small heap > wasn't able to sort the input in a single run. We have a bunch of > sorted data in memory which is the head of the same run whose tail is > already on disk; we now spill all of these tuples to disk. That > leaves only the heapified tuples in memory. We just ignore the fact > that they are a heap and treat them as unsorted. We repeatedly do the > following: read tuples until work_mem is full, sort them, and dump the > result to disk as a run. When all runs have been created, we merge > runs just as we do today. Right, so: having read this far, I'm almost sure that you intend that replacement selection is only ever used for the first run (we "go for broke" with RS). Good. > This algorithm seems very likely to beat what we do today in > practically all cases. The benchmarking Peter and others have already > done shows that building runs with quicksort rather than replacement > selection can often win even if the larger number of tapes requires a > multi-pass merge. The only cases where it didn't seem to be a clear > win involved data that was already in sorted order, or very close to > it. ...*and* where there was an awful lot of data, *and* where there was very little memory in an absolute sense (e.g. work_mem = 4MB). > But with this algorithm, presorted input is fine: we'll quicksort > some of it (which is faster than replacement selection because > quicksort checks for presorted input) and sort the rest with a *small* > heap (which is faster than our current approach of sorting it with a > big heap when the data is already in order). I'm not going to defend the precheck in our quicksort implementation. It's unadulterated nonsense. The B&M quicksort implementation's use of insertion sort does accomplish this pretty well, though. > On top of that, we'll > only write out the minimal amount of data to disk rather than all of > it. So we should still win. On the other hand, if the data is out of > order, then we will do only a little bit of replacement selection > before switching over to building runs by quicksorting, which should > also win. Yeah -- we retain much of the benefit of "quicksort with spillover", too, without any cost model. This is also better than "quicksort with spillover" in that it limits the size of the heap, and so limits the extent to which the algorithm can "helpfully" spend ages spilling from an enormous heap. The new GUC can be explained to users as a kind of minimum burst capacity for getting a "half internal, half external" sort, which seems intuitive enough. > The worst case I was able to think of for this algorithm is an input > stream that is larger than work_mem and almost sorted: the only > exception is that the record that should be exactly in the middle is > all the way at the end. > We need to not be horrible in that case, but there's > absolutely no reason to believe that we will be. We may even be > faster, but we certainly shouldn't be abysmally slower. Agreed. If we take a historical perspective, a 10MB or 30MB heap will still have a huge "juggling capacity" -- in practice it will almost certainly store enough tuples to make the "plate spinning circus trick" of replacement selection make the critical difference to run size. This new GUC is a delta between tuples for RS reordering. You can perhaps construct a "strategically placed banana skin" case to make this look bad before caching effects start to weigh us down, but I think you agree that it doesn't matter. "Juggling capacity" has nothing to do with modern hardware characteristics, except that modern machines are where the cost of excessive "juggling capacity" really hurts, so this is simple. It is simple *especially* because we can throw out the idea of a cost model that cares about caching effects in particular, but that's just one specific thing. BTW, you probably know this, but to be clear: When I talk about correlation, I refer specifically to what would appear within pg_stats.correlation as 1.0 -- I am not referring to a pg_stats.correlation of -1.0. The latter case is traditionally considered a worst case for RS. -- Peter Geoghegan
On Sun, Feb 7, 2016 at 11:00 AM, Peter Geoghegan <pg@heroku.com> wrote: > Right. Let me give you the executive summary first: I continue to > believe, following thinking about the matter in detail, that this is a > sensible compromise, that weighs everyone's concerns. It is pretty > close to a win-win. I just need you to confirm what I say here in > turn, so we're sure that we understand each other perfectly. Makes sense to me. >> The basic idea is that we will add a new GUC with a name like >> replacement_sort_mem that will have a default value in the range of >> 20-30MB; or possibly we will hardcode this value, but for purposes of >> this email I'm going to assume it's a GUC. If the value of work_mem >> or maintenance_work_mem, whichever applies, is smaller than the value >> of replacement_sort_mem, then the latter has no effect. > > By "no effect", you must mean that we always use a heap for the entire > first run (albeit for the tail, with a hybrid quicksort/heap > approach), but still use quicksort for every subsequent run, when it's > clearly established that we aren't going to get one huge run. Is that > correct? Yes. > It was my understanding, based on your emphasis on producing only a > single run, as well as your recent remarks on this thread about the > first run being special, that you are really only interested in the > presorted case, where one run is produced. That is, you are basically > not interested in preserving the general ability of replacement > selection to double run size in the event of a uniform distribution. > (That particular doubling property of replacement selection is now > technically lost by virtue of using this new hybrid model *anyway*, > although it will still make runs longer in general). > > You don't want to change the behavior of the current patch for the > second or subsequent run; that should remain a quicksort, pure and > simple. Do I have that right? Yes. > BTW, parallel sort should probably never use a heap anyway (ISTM that > that will almost certainly be based on external sorts in the end). A > heap is not really compatible with the parallel heap scan model. I don't think I agree with this part, though I think it's unimportant as far as the current patch is concerned. My initial thought is that parallel sort should work like this: 1. Each worker reads and sorts its input tuples just as it would in non-parallel mode. 2. If, at the conclusion of the sort, the input tuples are still in memory (quicksort) or partially in memory (quicksort with spillover), then write them all to a tape. If they are on multiple tapes, merge those to a single tape. If they are on a single tape, do nothing else at this step. 3. At this point, we have one sorted tape per worker. Perform a final merge pass to get the final result. The major disadvantage of this is that if the input hasn't been relatively evenly partitioned across the workers, the work of sorting will fall disproportionately on those that got more input. We could, in the future, make the logic more sophisticated. For example, if worker A is still reading the input and dumping sorted runs, worker B could start merging those runs. Or worker A could read tuples into a DSM instead of backend-private memory, and worker B could then sort them to produce a run. While such optimizations are clearly beneficial, I would not try to put them into a first parallel sort patch. It's too complicated. >> One thing I just thought of (after the call) is that it might be >> better for this GUC to be in units of tuples rather than in units of >> memory; it's not clear to me why the optimal heap size should be >> dependent on the tuple size, so we could have a threshold like 300,000 >> tuples or whatever. > > I think you're right that a number of tuples is the logical way to > express the heap size (as a GUC unit). I think that the ideal setting > for the GUC is large enough to recognize significant correlations in > input data, which may be clustered, but no larger (at least while > things don't all fit in L1 cache, or maybe L2 cache). We should "go > for broke" with replacement selection -- we don't aim for anything > less than ending up with 1 run by using the heap (merging 2 or 3 runs > rather than 4 or 6 is far less useful, maybe harmful, when one of them > is much larger). Therefore, I don't expect that we'll be practically > disadvantaged by having fewer "hands to juggle" tuples here (we'll > simply almost always have enough in practice -- more on that later). > FWIW I don't think that any benchmark we've seen so far justifies > doing less than "going for broke" with RS, even if you happen to have > a very conservative perspective. > > One advantage of a GUC is that you can set it to zero, and always get > a simple hybrid sort-merge strategy if that's desirable. I think that > it might not matter much with multi-gigabyte work_mem settings anyway, > though; you'll just see a small blip. Big (maintenance_)work_mem was > by far my greatest concern in relation to using a heap in general, so > I'm left pretty happy by this plan, I think. Lots of people can afford > a multi-GB maintenance_work_mem these days, and CREATE INDEX is gonna > be the most important case overall, by far. Agreed. I suspect that a default setting that is relatively small but not zero will be good for most people, but if some people find advantage in changing it to a smaller value, or zero, or a larger value, that's fine with me. >> 2. If (maintenance_)work_mem fills up completely, we will quicksort >> all of the data we have in memory. We will then regard the tail end >> of that sorted data, in an amount governed by replacement_sort_mem, as >> a heap, and use it to perform replacement selection until no tuples >> remain for the current run. Meanwhile, the rest of the sorted data >> remains in memory untouched. Logically, we're constructing a run of >> tuples which is split between memory and disk: the head of the run >> (what fits in all of (maintenance_)work_mem except for >> replacement_sort_mem) is in memory, and the tail of the run is on >> disk. > > I went back and forth on this during our call, but I now think that I > was right that there will need to be changes in order to make the tail > of the run a heap (*not* the quicksorted head), because routines like > tuplesort_heap_siftup() assume that state->memtuples[0] is the head of > the heap. This is currently assumed by the master branch for both the > currentRun/nextRun replacement selection heap, as well as the heap > used for merging. Changing this is probably fairly manageable, though > (probably still not going to use memmove() for this, contrary to my > remarks on the call). OK. I think if possible we want to try to do this by changing the Tuplesortstate to identify where the heap is, rather than by using memmove() to put it where we want it to be. >> 3. If we reach the end of input before replacement selection runs out >> of tuples for the current run, and if it finds no tuples for the next >> run prior to that time, then we are done. All of the tuples form a >> single run and we can return the tuples in memory first followed by >> the tuples on disk. This case is highly likely to be a huge win over >> what we have today, because (a) some portion of the tuples were sorted >> via quicksort rather than heapsort and that's faster, (b) the tuples >> that were sorted using a heap were sorted using a small heap rather >> than a big one, and (c) we only wrote out the minimal number of tuples >> to tape instead of, as we would have done today, all of them. > > Agreed. Cool. >> 4. If we reach this step, then replacement selection with a small heap >> wasn't able to sort the input in a single run. We have a bunch of >> sorted data in memory which is the head of the same run whose tail is >> already on disk; we now spill all of these tuples to disk. That >> leaves only the heapified tuples in memory. We just ignore the fact >> that they are a heap and treat them as unsorted. We repeatedly do the >> following: read tuples until work_mem is full, sort them, and dump the >> result to disk as a run. When all runs have been created, we merge >> runs just as we do today. > > Right, so: having read this far, I'm almost sure that you intend that > replacement selection is only ever used for the first run (we "go for > broke" with RS). Good. Yes, absolutely. >> This algorithm seems very likely to beat what we do today in >> practically all cases. The benchmarking Peter and others have already >> done shows that building runs with quicksort rather than replacement >> selection can often win even if the larger number of tapes requires a >> multi-pass merge. The only cases where it didn't seem to be a clear >> win involved data that was already in sorted order, or very close to >> it. > > ...*and* where there was an awful lot of data, *and* where there was > very little memory in an absolute sense (e.g. work_mem = 4MB). > >> But with this algorithm, presorted input is fine: we'll quicksort >> some of it (which is faster than replacement selection because >> quicksort checks for presorted input) and sort the rest with a *small* >> heap (which is faster than our current approach of sorting it with a >> big heap when the data is already in order). > > I'm not going to defend the precheck in our quicksort implementation. > It's unadulterated nonsense. The B&M quicksort implementation's use of > insertion sort does accomplish this pretty well, though. We'll leave that discussion for another day so as not to argue about it now. >> On top of that, we'll >> only write out the minimal amount of data to disk rather than all of >> it. So we should still win. On the other hand, if the data is out of >> order, then we will do only a little bit of replacement selection >> before switching over to building runs by quicksorting, which should >> also win. > > Yeah -- we retain much of the benefit of "quicksort with spillover", > too, without any cost model. This is also better than "quicksort with > spillover" in that it limits the size of the heap, and so limits the > extent to which the algorithm can "helpfully" spend ages spilling from > an enormous heap. The new GUC can be explained to users as a kind of > minimum burst capacity for getting a "half internal, half external" > sort, which seems intuitive enough. Right. I really like the idea of limiting the heap size - I'm quite hopeful that will let us hang onto the limited number of cases where RS is better while giving up on it pretty quickly when it's a loser. But even better, if you've got a case where RS is a win, limiting the heap size has an excellent chance of making it a bigger win. That's quite appealing, too. >> The worst case I was able to think of for this algorithm is an input >> stream that is larger than work_mem and almost sorted: the only >> exception is that the record that should be exactly in the middle is >> all the way at the end. > >> We need to not be horrible in that case, but there's >> absolutely no reason to believe that we will be. We may even be >> faster, but we certainly shouldn't be abysmally slower. > > Agreed. > > If we take a historical perspective, a 10MB or 30MB heap will still > have a huge "juggling capacity" -- in practice it will almost > certainly store enough tuples to make the "plate spinning circus > trick" of replacement selection make the critical difference to run > size. This new GUC is a delta between tuples for RS reordering. You > can perhaps construct a "strategically placed banana skin" case to > make this look bad before caching effects start to weigh us down, but > I think you agree that it doesn't matter. "Juggling capacity" has > nothing to do with modern hardware characteristics, except that modern > machines are where the cost of excessive "juggling capacity" really > hurts, so this is simple. It is simple *especially* because we can > throw out the idea of a cost model that cares about caching effects in > particular, but that's just one specific thing. Yep. I'm mostly relying on you to be correct about the actual performance characteristics of replacement selection here. If the cutover point when we go from RS to QS to build runs turns out to be wildly wrong, I plan to look sidelong in your direction. I don't think that's going to happen, though. > BTW, you probably know this, but to be clear: When I talk about > correlation, I refer specifically to what would appear within > pg_stats.correlation as 1.0 -- I am not referring to a > pg_stats.correlation of -1.0. The latter case is traditionally > considered a worst case for RS. Makes sense. -- Robert Haas EnterpriseDB: http://www.enterprisedb.com The Enterprise PostgreSQL Company
On Sun, Feb 7, 2016 at 8:21 PM, Robert Haas <robertmhaas@gmail.com> wrote: > On Sun, Feb 7, 2016 at 11:00 AM, Peter Geoghegan <pg@heroku.com> wrote: > > It was my understanding, based on your emphasis on producing only a > > single run, as well as your recent remarks on this thread about the > > first run being special, that you are really only interested in the > > presorted case, where one run is produced. That is, you are basically > > not interested in preserving the general ability of replacement > > selection to double run size in the event of a uniform distribution. >... > > You don't want to change the behavior of the current patch for the > > second or subsequent run; that should remain a quicksort, pure and > > simple. Do I have that right? > > Yes. I'm not even sure this is necessary. The idea of missing out on producing a single sorted run sounds bad but in practice since we normally do the final merge on the fly there doesn't seem like there's really any difference between reading one tape or reading two or three tapes when outputing the final results. There will be the same amount of I/O happening and a 2-way or 3-way merge for most data types should be basically free. On Sun, Feb 7, 2016 at 8:21 PM, Robert Haas <robertmhaas@gmail.com> wrote: > 3. At this point, we have one sorted tape per worker. Perform a final > merge pass to get the final result. I don't even think you have to merge until you get one tape per worker. You can statically decide how many tapes you can buffer in memory based on work_mem and merge until you get N/workers tapes so that a single merge in the gather node suffices. I would expect that to nearly always mean the workers are only responsible for generating the initial sorted runs and the single merge pass is done in the gather node on the fly as the tuples are read. -- greg
On Sun, Feb 7, 2016 at 10:51 AM, Greg Stark <stark@mit.edu> wrote: >> > You don't want to change the behavior of the current patch for the >> > second or subsequent run; that should remain a quicksort, pure and >> > simple. Do I have that right? >> >> Yes. > > I'm not even sure this is necessary. The idea of missing out on > producing a single sorted run sounds bad but in practice since we > normally do the final merge on the fly there doesn't seem like there's > really any difference between reading one tape or reading two or three > tapes when outputing the final results. There will be the same amount > of I/O happening and a 2-way or 3-way merge for most data types should > be basically free. I basically agree with you, but it seems possible to fix the regression (generally misguided though those regressed cases are). It's probably easiest to just fix it. -- Peter Geoghegan
On Sun, Feb 7, 2016 at 4:50 PM, Peter Geoghegan <pg@heroku.com> wrote: >> I'm not even sure this is necessary. The idea of missing out on >> producing a single sorted run sounds bad but in practice since we >> normally do the final merge on the fly there doesn't seem like there's >> really any difference between reading one tape or reading two or three >> tapes when outputing the final results. There will be the same amount >> of I/O happening and a 2-way or 3-way merge for most data types should >> be basically free. > > I basically agree with you, but it seems possible to fix the > regression (generally misguided though those regressed cases are). > It's probably easiest to just fix it. On a related note, we should probably come up with a way of totally supplanting the work_mem model with something smarter in the next couple of years. Something that treats memory as a shared resource even when it's allocated privately, per-process. This external sort stuff really smooths out the cost function of sorts. ISTM that that makes the idea of dynamic memory budgets (in place of a one size fits all work_mem) seem desirable for the first time. That said, I really don't have a good sense of how to go about moving in that direction at this point. It seems less than ideal that DBAs have to be so conservative in sizing work_mem. -- Peter Geoghegan
On Sun, Feb 7, 2016 at 4:50 PM, Peter Geoghegan <pg@heroku.com> wrote: >> I'm not even sure this is necessary. The idea of missing out on >> producing a single sorted run sounds bad but in practice since we >> normally do the final merge on the fly there doesn't seem like there's >> really any difference between reading one tape or reading two or three >> tapes when outputing the final results. There will be the same amount >> of I/O happening and a 2-way or 3-way merge for most data types should >> be basically free. > > I basically agree with you, but it seems possible to fix the > regression (generally misguided though those regressed cases are). > It's probably easiest to just fix it. Here is a benchmark on my laptop: $ pgbench -i -s 500 --unlogged This results in a ~1GB accounts PK: postgres=# \di+ pgbench_accounts_pkey List of relations ─[ RECORD 1 ]────────────────────── Schema │ public Name │ pgbench_accounts_pkey Type │ index Owner │ pg Table │ pgbench_accounts Size │ 1071 MB Description │ The query I'm testing is: "reindex index pgbench_accounts_pkey;" Now, with a maintenance_work_mem of 5MB, the most recent revision of my patch takes about 54.2 seconds to complete this, as compared to master's 44.4 seconds. So, clearly a noticeable regression there of just under 20%. I did not see a regression with a 5MB maintenance_work_mem when pgbench scale was 100, though. And, with the default maintenance_work_mem of 64MB, it's a totally different story -- my patch takes about 28.3 seconds, whereas master takes 48.5 seconds (i.e. longer than with 5MB). My patch needs a 56-way final merge with the 64MB maintenance_work_mem case, and 47 distinct merge steps, plus a final on-the-fly merge for the 5MB maintenance_work_mem case. So, a huge amount of merging, but RS still hardly pays for itself. With the regressed case for my patch, we finish sorting *runs* about 15 seconds in to a 54.2 second operation -- very early. So it isn't "quicksort vs replacement selection", so much as "polyphase merge vs replacement selection". There is a good reason to think that we can make progress on fixing that regression by doubling down on the general strategy of improving cache characteristics, and being cleverer about memory use during non-final merging, too. I looked at what it would take to make the heap a smaller part of memtuples, along the lines Robert and I talked about, and I think it's non-trivial because it needs to make the top of the heap something other than memtuples[0]. I'd need to change the heap code, which already has 3 reasons for existing (RS, merging, and top-N heap). I'll find it really hard to justify the effort, and especially the risk of adding bugs, for a benefit that there is *scant* evidence for. My guess is that the easiest, and most sensible way to fix the ~20% regression seen here is to introduce batch memory allocation to non-final merge steps, which is where most time was spent. (For simplicity, that currently only happens during the final merge phase, but I could revisit that -- seems not that hard). Now, I accept that the cost model has to go. So, what I think would be best is if we still added a GUC, like the replacement_sort_mem suggestion that Robert made. This would be a threshold for using what is currently called "quicksort with spillover". There'd be no cost model. Jeff Janes also suggested something like this. The only regression that I find concerning is the one reported by Jeff Janes [1]. That didn't even involve a correlation, though, so no reason to think that it would be at all helped by what Robert and I talked about. It seemed like the patch happened to have the effect of tickling a pre-existing problem with polyphase merge -- what Jeff called an "anti-sweetspot". Jeff had a plausible theory for why that is. So, what if we try to fix polyphase merge? That would be easier. We could look at the tape buffer size, and the number of tapes, as well as memory access patterns. We might even make more fundamental changes to polyphase merge, since we don't use the more advanced variant that I think correlation is a red herring. Knuth suggests that his algorithm 5.4.3, cascade merge, has more efficient distribution of runs. The bottom line is that there will always be some regression somewhere. I'm not sure what the guiding principle for when that becomes unacceptable is, but you did seem sympathetic to the idea that really low work_mem settings (e.g. 4MB) with really big inputs were not too concerning [2]. I'm emphasizing Jeff's case now because I, like you [2], am much more worried about maintenance_work_mem default cases with regressions than anything else, and that *was* such a case. Like Jeff Janes, I don't care about his other regression of about 5% [3], which involved a 4MB work_mem + 100 million tuples. The other case (the one I do care about) was 64MB + 400 million tuples, and was a much bigger regression, which is suggestive of the unpredictable nature of problems with polyphase merge scheduling that Jeff talked about. Maybe we just got unlucky there, but that risk should not blind us to the fact that overwhelmingly, replacement selection is the wrong thing. I'm sorry that I've reversed myself like this, Robert, but I'm just not seeing a benefit to what we talked about, but I do see a cost. [1] http://www.postgresql.org/message-id/CAMkU=1zKBOzkX-nqE-kJFFMyNm2hMGYL9AsKDEUHhwXASsJEbg@mail.gmail.com [2] http://www.postgresql.org/message-id/CA+TgmoZGFt6BAxW9fYOn82VAf1u=V0ZZx3bXMs79phjg_9NYjQ@mail.gmail.com [3] http://www.postgresql.org/message-id/CAM3SWZTYneCG1oZiPwRU=J6ks+VpRxt2Da1ZMmqFBrd5jaSJSA@mail.gmail.com -- Peter Geoghegan
On 2/7/16 8:57 PM, Peter Geoghegan wrote: > It seems less than ideal that DBAs have to be so > conservative in sizing work_mem. +10 -- Jim Nasby, Data Architect, Blue Treble Consulting, Austin TX Experts in Analytics, Data Architecture and PostgreSQL Data in Trouble? Get it in Treble! http://BlueTreble.com
On Mon, Feb 15, 2016 at 8:43 PM, Jim Nasby <Jim.Nasby@bluetreble.com> wrote: > On 2/7/16 8:57 PM, Peter Geoghegan wrote: >> >> It seems less than ideal that DBAs have to be so >> conservative in sizing work_mem. > > > +10 I was thinking about this over the past couple weeks. I'm starting to think the quicksort runs gives at least the beginnings of a way forward on this front. Keeping in mind that we know how many tapes we can buffer in memory and the number is likely to be relatively large -- on the order of 100+ is typical, what if do something like the following rough sketch: Give users two knobs, a lower bound "sort in memory using quicksort" memory size and an upper bound "absolutely never use more than this" which they can set to a substantial fraction of physical memory. Then when we overflow the lower bound we start generating runs, the first one being of that length. Each run we generate we double (or increase by 50% or something) until we hit the maximum. That means that the first few runs may be shorter than necessary but we have enough tapes available that that doesn't hurt much and we'll eventually get to a large enough run size that we won't run out of tapes and can still do a single final (on the fly) merge. In fact what's really attractive about this idea is that it might give us a reasonable spot to do some global system resource management. Each time we want to increase the run size we check some shared memory counter of how much memory is in use and refuse to increase if there's too much in use (or if we're using too large a fraction of it or some other heuristic). The key point is that since we don't need to decide up front at the beginning of the sort and we don't need to track it continuously there is neither too little nor too much contention on this shared memory variable. Also the behaviour would be not too chaotic if there's a user-tunable minimum and the other activity in the system only controls how more memory it can steal from the global pool on top of that. -- greg
On Mon, Feb 15, 2016 at 3:45 PM, Greg Stark <stark@mit.edu> wrote: > I was thinking about this over the past couple weeks. I'm starting to > think the quicksort runs gives at least the beginnings of a way > forward on this front. As I've already pointed out several times, I wrote a tool that makes it easy to load sortbenchmark.org data into a PostgreSQL table: https://github.com/petergeoghegan/gensort (You should use the Python script that invokes the "gensort" utility -- see its "--help" display for details). This seems useful as a standard benchmark, since it's perfectly deterministic, allowing the user to create arbitrarily large tables to use for sort benchmarks. Still, it doesn't produce data that is any way organic; sort data is uniformly distributed. Also, it produces a table that really only has one attribute to sort on, a text attribute. I suggest looking at real world data, too. I have downloaded UK land registry data, which is a freely available dataset about property sales in the UK since the 1990s, of which there have apparently been about 20 million (I started with a 20 million line CSV file). I've used COPY to load the data into one PostgreSQL table. I attach instructions on how to recreate this, and some suggested CREATE INDEX statements that seemed representative to me. There are a variety of Postgres data types in use, including UUID, numeric, and text. The final Postgres table is just under 3GB. I will privately make available a URL that those CC'd here can use to download a custom format dump of the table, which comes in at 1.1GB (ask me off-list if you'd like to get that URL, but weren't CC'd here). This URL is provided as a convenience for reviewers, who can skip my detailed instructions. An expensive rollup() query on the "land_registry_price_paid_uk" table is interesting. Example: select date_trunc('year', transfer_date), county, district, city, sum(price) from land_registry_price_paid_uk group by rollup (1, county, district, city); Performance is within ~5% of an *internal* sort with the patch series applied, even though ~80% of time is spent copying and sorting SortTuples overall in the internal sort case (the internal case cannot overlap sorting and aggregate processing, since it has no final merge step). This is a nice demonstration of how this work has significantly blurred the line between internal and external sorts. -- Peter Geoghegan
Attachment
Hi, On Mon, 2015-12-28 at 15:03 -0800, Peter Geoghegan wrote: > On Fri, Dec 18, 2015 at 11:57 AM, Peter Geoghegan <pg@heroku.com> wrote: > > BTW, I'm not necessarily determined to make the new special-purpose > > allocator work exactly as proposed. It seemed useful to prioritize > > simplicity, and currently so there is one big "huge palloc()" with > > which we blow our memory budget, and that's it. However, I could > > probably be more clever about "freeing ranges" initially preserved for > > a now-exhausted tape. That kind of thing. > > Attached is a revision that significantly overhauls the memory patch, > with several smaller changes. I was thinking about running some benchmarks on this patch, but the thread is pretty huge so I want to make sure I'm not missing something and this is indeed the most recent version. Is that the case? regards -- Tomas Vondra http://www.2ndQuadrant.com PostgreSQL Development, 24x7 Support, Remote DBA, Training & Services
On Thu, Mar 10, 2016 at 5:40 AM, Tomas Vondra <tomas.vondra@2ndquadrant.com> wrote: > I was thinking about running some benchmarks on this patch, but the > thread is pretty huge so I want to make sure I'm not missing something > and this is indeed the most recent version. Wait 24 - 48 hours, please. Big update coming. -- Peter Geoghegan
On Thu, Mar 10, 2016 at 1:40 PM, Tomas Vondra <tomas.vondra@2ndquadrant.com> wrote:
> I was thinking about running some benchmarks on this patch, but the
> thread is pretty huge so I want to make sure I'm not missing something
> and this is indeed the most recent version.
I also ran some preliminary benchmarks just before FOSDEM and intend to get back to in after running different benchmarks. These are preliminary because it was only a single run and on a machine that wasn't dedicated for benchmarks. These were comparing the quicksort-all-runs patch against HEAD at the time without the memory management optimizations which I think are independent of the sort algorithm.
It looks to me like the interesting space to test is on fairly small work_mem compared to the data size. There's a general slowdown on 4MB-8MB work_mem when the data set is more than a gigabyte but but even in the worst case it's only a 30% slowdown and the speedup in the more realistic scenarios looks at least as big.

I want to rerun these on a dedicated machine and with trace_sort enabled so that we can see how many merge passes were actually happening and how much I/O was actually happening.
--
greg
Attachment
On Thu, Mar 10, 2016 at 10:39 AM, Greg Stark <stark@mit.edu> wrote: > I want to rerun these on a dedicated machine and with trace_sort > enabled so that we can see how many merge passes were actually > happening and how much I/O was actually happening. Putting the results in context, by keeping trace_sort output with the results is definitely a good idea here. Otherwise, it's almost impossible to determine what happened after the fact. I have had "trace_sort = on" in my dev postgresql.conf for some time now. :-) When I produce my next revision, we should focus on regressions at the low end, like the 4MB work_mem for multiple GB table size cases you show here. So, I ask that any benchmarks that you or Tomas do look at that first and foremost. It's clear that in high memory environments the patch significantly improves performance, often by as much as 2.5x, and so that isn't really a concern anymore. I think we may be able to comprehensively address Robert's concerns about regressions with very little work_mem and lots of data by fixing a problem with polyphase merge. More to come soon. -- Peter Geoghegan
On Sun, Feb 14, 2016 at 8:01 PM, Peter Geoghegan <pg@heroku.com> wrote: > The query I'm testing is: "reindex index pgbench_accounts_pkey;" > > Now, with a maintenance_work_mem of 5MB, the most recent revision of > my patch takes about 54.2 seconds to complete this, as compared to > master's 44.4 seconds. So, clearly a noticeable regression there of > just under 20%. I did not see a regression with a 5MB > maintenance_work_mem when pgbench scale was 100, though. I've fixed this regression, and possibly all regressions where workMem > 4MB. I've done so without resorting to making the heap structure more complicated, or using a heap more often than when replacement_sort_mem is exceeded by work_mem or maintenance_work_mem (so replacement_sort_mem becomes something a bit different to what we discussed, Robert -- more on that later). This seems like an "everybody wins" situation, because in this revision the patch series is now appreciably *faster* where the amount of memory available is only a tiny fraction of the total input size. Jeff Janes deserves a lot of credit for helping me to figure out how to do this. I couldn't get over his complaint about the regression he saw a few months back. He spoke of an "anti-sweetspot" in polyphase merge, and how he suspected that to be the real culprit (after all, most of his time was spent merging, with or without the patch applied). He also said that reverting the memory batch/pool patch made things go a bit faster, somewhat ameliorating his regression (when just the quicksort patch was applied). This made no sense to me, since I understood the memory batching patch to be orthogonal to the quicksort thing, capable of being applied independently, and more or less a strict improvement on master, no matter what the variables of the sort are. Jeff's regressed case especially made no sense to me (and, I gather, to him) given that the regression involved no correlation, and so clearly wasn't reliant on generating far fewer/longer runs than the patch (that's the issue we've discussed more than any other now -- it's a red herring, it seems). As I suspected out loud on February 14th, replacement selection mostly just *masked* the real problem: the problem of palloc() fragmentation. There doesn't seem to be much of an issue with the scheduling of polyphase merging, once you fix palloc() fragmentation. I've created a new revision, incorporating this new insight. New Revision ============ Attached revision of patch series: 1. Creates a separate memory context for tuplesort's copies of caller's tuples, which can be reset at key points, avoiding fragmentation. Every SortTuple.tuple is allocated there (with trivial exception); *everything else*, including the memtuples array, is allocated in the existing tuplesort context, which becomes the parent of this new "caller's tuples" context. Roughly speaking, that means that about half of total memory for the sort is managed by each context in common cases. Even with a high work_mem memory budget, memory fragmentation could previously get so bad that tuplesort would in effect claim a share of memory from the OS that is *significantly* higher than the work_mem budget allotted to its sort. And with low work_mem settings, fragmentation previously made palloc() thrash the sort, especially during non-final merging. In this latest revision, tuplesort now almost gets to use 100% of the memory that was requested from the OS by palloc() is cases tested. 2. Loses the "quicksort with spillover" case entirely, making the quicksort patch significantly simpler. A *lot* of code was thrown out. This change is especially significant because it allowed me to remove the cost model that Robert took issue with so vocally. "Quicksort with spillover" was always far less important than the basic idea of using quicksort for external sorts, so I'm not sad to see it go. And, I thought that the cost model was pretty bad myself. 3. Fixes cost_sort(), making optimizer account for the fact that runs are now about sort_mem-sized, not (sort_mem * 2)-sized. While I was at it, I made cost_sort() more optimistic about the amount of random I/O required relative to sequential I/O. This additional change to cost_sort() was probably overdue. 4. Restores the ability of replacement selection to generate one run and avoid any merging (previously, only one really long run and one short run was possible, because at the time I conceptualized replacement selection as being all about enabling "quicksort with spillover", which quicksorted that second run in memory). This only-one-run case is the case that Robert particularly cared about, and it's fully restored when RS is in use (which can still only happen for the first run, just never for the benefit of the now-axed "quicksort with spillover" case). 5. Adds a new GUC, "replacement_sort_mem". The default setting is 16MB. Docs are included. If work_mem/maintenance_work_mem is less than or equal to this, the first (and hopefully only) run uses replacement selection. "replacement_sort_mem" is a different thing to the concept for a GUC Robert and I discussed (only the name is the same). That other concept for a GUC related to the hybrid heap/quicksort idea (it controlled how big the heap portion of memtuples was supposed to be, in a speculative world where the patch took that "hybrid" approach [1] at all). In light of this new information about palloc() fragmentation, and given the risk to tuplesort's stability posed by implementing this "hybrid" algorithm, this seems like a good compromise. I cannot see an upside to pursuing the "hybrid" approach now. I regret reversing my position on that, but that's just how it happened. Since Robert was seemingly only worried about regressions, which are fixed now for a variety of cases that I tested, I'm optimistic that this will be acceptable to him. I believe that replacement_sort_mem as implemented here is quite useful, although mostly because I see some further opportunities for it. Replacement Selection uses -------------------------- What opportunities, you ask? Maybe CREATE INDEX can be made to accept a "presorted" parameter, letting the user promise that the input is more or less presorted. This allows tuplesort to only use a tiny heap, while having it throw an error if it cannot produce one long run (i.e. CREATE INDEX is documented as raising an error if the input is not more or less presorted). The nice thing about that idea is that we can be very optimistic about the data actually being more or less presorted, so the implementation doesn't *actually* produce one long run -- it produces one long *index*, with IndexTuples passed back to nbtsort.c as soon as the heap fills for the first time, a bit like an on-the-fly merge. Unlike an on-the-fly merge, no tapes or temp files are actually involved; we write out IndexTuples by actually writing out the index optimistically. There is a significant saving by using a heap *because there is no need for a TSS_SORTEDONTAPE pass over the data*. We succeed at doing it all at once with a tiny heap, or die trying. So, in a later version of Postgres (9.7?), replacement_sort_mem becomes more important because of this "presorted" CREATE INDEX parameter. That's a relatively easy patch to write, but it's not 9.6 material. Commits ------- Note that the attached revision makes the batch memory patch the first commit in the patch series. It might be useful to get that one out of the way first, since I imagine it is now considered the least controversial, and is perhaps the simplest of the two big patches in the series. I'm not very optimistic about the memory prefetch patch 0003-* getting committed, but so far I've only seen it help, and all of my testing is based on having it applied. In any case, it's clearly way way less important than the other two patches. Testing ------- N.B.: The debug patch, 0004-*, should not be applied during benchmarking. I've used amcheck [2] to test this latest revision -- the tool ought to not see any problems with any index created with the patch applied. Reviewers might find it helpful to use amcheck, too. As 9.6 is stabilized, I anticipate that amcheck will give us a fighting chance at early detection of any bugs that might have slipped into tuplesort, or a B-Tree operator class. Since we still don't even have one single test of the external sort code [3], it's just as well. If we wanted to test external sorting, maybe we'd do that by adding tests to amcheck, that are not run by default, much like test_decoding, which tests logical decoding but is not targeted by "make installcheck"; that would allow the tests to be fairly comprehensive without being annoying. Using amcheck neatly side-steps issues with the portability of "expected" pg_regress output when collatable type sorting is tested. Thoughts? [1] http://www.postgresql.org/message-id/CA+TgmoY87y9FuZ=NE7JayH2emtovm9Jp9aLfFWunjF3utq4hfg@mail.gmail.com [2] https://commitfest.postgresql.org/9/561/ [3] http://pgci.eisentraut.org/jenkins/job/postgresql_master_coverage/Coverage/src/backend/utils/sort/tuplesort.c.gcov.html -- Peter Geoghegan
Attachment
On Thu, Mar 10, 2016 at 9:54 PM, Peter Geoghegan <pg@heroku.com> wrote: > 1. Creates a separate memory context for tuplesort's copies of > caller's tuples, which can be reset at key points, avoiding > fragmentation. Every SortTuple.tuple is allocated there (with trivial > exception); *everything else*, including the memtuples array, is > allocated in the existing tuplesort context, which becomes the parent > of this new "caller's tuples" context. Roughly speaking, that means > that about half of total memory for the sort is managed by each > context in common cases. Even with a high work_mem memory budget, > memory fragmentation could previously get so bad that tuplesort would > in effect claim a share of memory from the OS that is *significantly* > higher than the work_mem budget allotted to its sort. And with low > work_mem settings, fragmentation previously made palloc() thrash the > sort, especially during non-final merging. In this latest revision, > tuplesort now almost gets to use 100% of the memory that was requested > from the OS by palloc() is cases tested. I spent some time looking at this part of the patch yesterday and today. This is not a full review yet, but here are some things I noticed: - I think that batchmemtuples() is somewhat weird. Normally, grow_memtuples() doubles the size of the array each time it's called. So if you somehow called this function when you still had lots of memory available, it would just double the size of the array. However, I think the expectation is that it's only going to be called when availMem is less than half of allowedMem, in which case we're going to get the special "last increment of memtupsize" behavior, where we expand the memtuples array by some multiple between 1.0 and 2.0 based on allowedMem/memNowUsed. And after staring at this for a long time ... yeah, I think this does the right thing. But it certainly is hairy. - It's not exactly clear what you mean when you say that the tuplesort context contains "everything else". I don't understand why that only ends up containing half the memory ... what, other than the memtuples array, ends up there? - If I understand correctly, the point of the MemoryContextReset call is: there wouldn't be any tuples in memory at that point anyway. But the OS-allocated chunks might be divided up into a bunch of small chunks that then got stuck on freelists, and those chunks might not be the right size for the next merge pass. Resetting the context avoids that problem by blowing up the freslists. Right? Clever. - I haven't yet figured out why we use batching only for the final on-the-fly merge pass, instead of doing it for all merges. I expect you have a reason. I just don't know what it is. - I have also not yet figured out why you chose to replace state->datumTypByVal with state->tuples and reverse the sense. I bet there's a reason for this, too. I don't know what it is, either. That's as far as I've gotten thus far. -- Robert Haas EnterpriseDB: http://www.enterprisedb.com The Enterprise PostgreSQL Company
On Wed, Mar 16, 2016 at 3:31 PM, Robert Haas <robertmhaas@gmail.com> wrote: > I spent some time looking at this part of the patch yesterday and > today. Thanks for getting back to it. > - I think that batchmemtuples() is somewhat weird. Normally, > grow_memtuples() doubles the size of the array each time it's called. > So if you somehow called this function when you still had lots of > memory available, it would just double the size of the array. > However, I think the expectation is that it's only going to be called > when availMem is less than half of allowedMem, in which case we're > going to get the special "last increment of memtupsize" behavior, > where we expand the memtuples array by some multiple between 1.0 and > 2.0 based on allowedMem/memNowUsed. That's right. It might be possible for the simple doubling behavior to happen under artificial conditions instead, for example when we have enormous individual tuples, but if that does happen it's still correct. I just didn't think it was worth worrying about giving back more memory in such extreme edge-cases. > And after staring at this for a > long time ... yeah, I think this does the right thing. But it > certainly is hairy. No arguments from me here. I think this is justified, though. It's great that palloc() provides a simple, robust abstraction. However, there are a small number of modules in the code, including tuplesort.c, where we need to be very careful about memory management. Probably no more than 5 and no less than 3. In these places, large memory allocations are the norm. We ought to pay close attention to memory locality, heap fragmentation, that memory is well balanced among competing considerations, etc. It's entirely appropriate that we'd go to significant lengths to get it right in these places using somewhat ad-hoc techniques, simply because these are the places where we'll get a commensurate benefit. Some people might call this adding custom memory allocators, but I find that to be a loaded term because it suggests intimate involvement from mcxt.c. > - It's not exactly clear what you mean when you say that the tuplesort > context contains "everything else". I don't understand why that only > ends up containing half the memory ... what, other than the memtuples > array, ends up there? I meant that the existing memory context "sortcontext" contains everything else that has anything to do with sorting. Everything that it contains in the master branch it continues to contain today, with the sole exception of a vast majority of caller's tuples. So, "sortcontext" continues to include everything you can think of: * As you pointed out, the memtuples array. * SortSupport state (assuming idiomatic usage of the API, at least). * State specific to the cluster case. * Transient state, specific to the index case (i.e. scankey memory) * logtape.c stuff. * Dynamically allocated stuff for managing tapes (see inittapes()) * For the sake of simplicity, a tiny number of remaining tuples (from "overflow" allocations, or from when we need to free a tape's entire batch when it is one tuple from exhaustion). This is for tuples that the tuplesort caller needs to pfree() anyway, per the tuplesort_get*tuple() API. It's just easier to put these allocations in the "main" context, to avoid having to reason about any consequences to calling MemoryContextReset() against our new tuple context. This precaution is just future-proofing IMV. I believe that this list is exhaustive. > - If I understand correctly, the point of the MemoryContextReset call > is: there wouldn't be any tuples in memory at that point anyway. But > the OS-allocated chunks might be divided up into a bunch of small > chunks that then got stuck on freelists, and those chunks might not be > the right size for the next merge pass. Resetting the context avoids > that problem by blowing up the freslists. Right? Your summary of the practical benefit is accurate. While I've emphasized regressions at the low-end with this latest revision, it's also true that resetting helps in memory rich environments, when we switch from retail palloc() calls to the final merge step's batch allocation, which palloc() seemed to do very badly with. It makes sense that this abrupt change in the pattern of allocations could cause significant heap memory fragmentation. > Clever. Thanks. Introducing a separate memory context that is strictly used for caller tuples makes it clear and obvious that it's okay to call MemoryContextReset() when state->memtupcount == 0. It's not okay to put anything in the new context that could break the calls to MemoryContextReset(). You might not have noticed that a second MemoryContextReset() call appears in the quicksort patch, which helps a bit too. I couldn't easily make that work with the replacement selection heap, because master's tupleosrt.c never fully empties its RS heap until the last run. I can only perform the first call to MemoryContextReset() in the memory patch because it happens at a point memtupcount == 0 -- it's called when a run is merged (outside a final on-the-fly merge). Notice that the mergeonerun() loop invariant is: while (state->memtupcount > 0) { ... } So, it must be that state->memtupcount == 0 (and that we have no batch memory) when I call MemoryContextReset() immediately afterwards. > - I haven't yet figured out why we use batching only for the final > on-the-fly merge pass, instead of doing it for all merges. I expect > you have a reason. I just don't know what it is. The most obvious reason, and possibly the only reason, is that I have license to lock down memory accounting in the final on-the-fly merge phase. Almost equi-sized runs are the norm, and code like this is no longer obligated to work: FREEMEM(state, GetMemoryChunkSpace(stup->tuple)); That's why I explicitly give up on "conventional accounting". USEMEM() and FREEMEM() calls become unnecessary for this case that is well locked down. Oh, and I know that I won't use most tapes, so I can give myself a FREEMEM() refund before doing the new grow_memtuples() thing. I want to make batch memory usable for runs, too. I haven't done that either for similar reasons. FWIW, I see no great reason to worry about non-final merges. > - I have also not yet figured out why you chose to replace > state->datumTypByVal with state->tuples and reverse the sense. I bet > there's a reason for this, too. I don't know what it is, either. It makes things slightly easier to make this a generic property of any tuplesort: "Can SortTuple.tuple ever be set?", rather than allowing it to remain a specific property of a datum tuplesort. state->datumTypByVal often isn't initialized in master, and so cannot be checked as things stand (unless the code is in a datum-case-specific routine). This new flag controls batch memory in slightly higher-level way than would otherwise be possible. It also controls the memory prefetching added by patch/commit 0003-*, FWIW. -- Peter Geoghegan
On Wed, Mar 16, 2016 at 9:42 PM, Peter Geoghegan <pg@heroku.com> wrote: >> - I haven't yet figured out why we use batching only for the final >> on-the-fly merge pass, instead of doing it for all merges. I expect >> you have a reason. I just don't know what it is. > > The most obvious reason, and possibly the only reason, is that I have > license to lock down memory accounting in the final on-the-fly merge > phase. Almost equi-sized runs are the norm, and code like this is no > longer obligated to work: > > FREEMEM(state, GetMemoryChunkSpace(stup->tuple)); > > That's why I explicitly give up on "conventional accounting". USEMEM() > and FREEMEM() calls become unnecessary for this case that is well > locked down. Oh, and I know that I won't use most tapes, so I can give > myself a FREEMEM() refund before doing the new grow_memtuples() thing. > > I want to make batch memory usable for runs, too. I haven't done that > either for similar reasons. FWIW, I see no great reason to worry about > non-final merges. Fair enough. My concern was mostly whether the code would become simpler if we always did this when merging, instead of only on the final merge. But the final merge seems to be special in quite a few respects, so maybe not. -- Robert Haas EnterpriseDB: http://www.enterprisedb.com The Enterprise PostgreSQL Company
On Wed, Mar 16, 2016 at 6:42 PM, Peter Geoghegan <pg@heroku.com> wrote: >> - I think that batchmemtuples() is somewhat weird. Normally, >> grow_memtuples() doubles the size of the array each time it's called. >> So if you somehow called this function when you still had lots of >> memory available, it would just double the size of the array. >> However, I think the expectation is that it's only going to be called >> when availMem is less than half of allowedMem, in which case we're >> going to get the special "last increment of memtupsize" behavior, >> where we expand the memtuples array by some multiple between 1.0 and >> 2.0 based on allowedMem/memNowUsed. > > That's right. It might be possible for the simple doubling behavior to > happen under artificial conditions instead, for example when we have > enormous individual tuples, but if that does happen it's still > correct. I just didn't think it was worth worrying about giving back > more memory in such extreme edge-cases. Come to think of it, maybe the pass-by-value datum sort case should also call batchmemtuples() too (or something similar). If you look at how beginmerge() is called, you'll see that that doesn't happen. Obviously this case is not entitiled to a "memtupsize * STANDARDCHUNKHEADERSIZE" refund, since of course there never was any overhead like that at any point. And, obviously this case has no need for batch memory at all. However, it is entitled to get a refund for non-used tapes (accounted for, but, it turns out, never allocated tapes). It should then get the benefit of that refund by way of growing memtuples through a similar "final, honestly, I really mean it this time" call to grow_memtuples(). So, while the "memtupsize * STANDARDCHUNKHEADERSIZE refund" part should still be batch-specific (i.e. used for the complement of tuplesort cases, never the datum pass-by-val case), the new grow_memtuples() thing should always happen with external sorts. The more I think about it, the more I wonder if we should commit something like the debugging patch 0004-* (enabled only when trace_sort = on, of course). Close scrutiny of what tuplesort.c is doing with memory is important. -- Peter Geoghegan
On Wed, Mar 16, 2016 at 9:42 PM, Peter Geoghegan <pg@heroku.com> wrote: > On Wed, Mar 16, 2016 at 3:31 PM, Robert Haas <robertmhaas@gmail.com> wrote: >> I spent some time looking at this part of the patch yesterday and >> today. > > Thanks for getting back to it. OK, I have now committed 0001, and separately, some comment improvements - or at least, I think they are improvements - based on this discussion. Thanks. -- Robert Haas EnterpriseDB: http://www.enterprisedb.com The Enterprise PostgreSQL Company
On Thu, Mar 17, 2016 at 1:13 PM, Robert Haas <robertmhaas@gmail.com> wrote: > OK, I have now committed 0001, and separately, some comment > improvements - or at least, I think they are improvements - based on > this discussion. Thanks! Your changes look good to me. It's always interesting to learn what wasn't so obvious to you when you review my patches. It's probably impossible to stare at something like tuplesort.c for as long as I have and get that balance just right. -- Peter Geoghegan
On Thu, Mar 17, 2016 at 1:13 PM, Robert Haas <robertmhaas@gmail.com> wrote: > OK, I have now committed 0001 I attach a revision of the external quicksort patch and supplementary small patches, rebased on top of the master branch. Changes: 1. As I mentioned on the "Improve memory management for external sorts" -committers thread, we should protect against currentRun integer overflow. This new revision does so. I'm not sure if that change needs to be back-patched; I just don't want to take any risks, and see this as low cost insurance. Really low workMem sorts are now actually fast enough that this seems like something that could happen on a misconfigured system. 2. Add explicit constants for special run numbers that replacement selection needs to care about in particular. I did this because change 1 reminded me of the "currentRun vs. SortTuple.tupindex" run numbering subtleties. The explicit use of certain run number constants seems to better convey some tricky details, in part by letting me add a few documenting if obvious assertions. It's educational to be able to grep for the these constants (e.g., the new HEAP_RUN_NEXT constant) to jump to the parts of the code that need to think about replacement selection. As things were, that code relied on too much from too great a distance (arguably this is true even in the master branch). This change in turn led to minor wordsmithing to adjacent areas here and there, most of it subtractive. As an example of where this helps, ISTM that the assertion added to the routine tuplesort_heap_insert() is now self-documenting, which wasn't the case before. 3. There was one very tricky consideration around an edge-case that required careful thought. This was an issue within my new function dumpbatch(). It could previously perform what turns out to be a superfluous selectnewtape() call when we take the dumpbatch() "state->memtupcount == 0" early return path (see the last revision for full details of that now-axed code path). Now, we accept that there may on occasion be 0 tuple runs. In other words, we now never returned early from within dumpbatch(). There was previously no explanation for why it was okay to have a superfluous selectnewtape() call. However, needing to be certain that any newly selected destTape tape will go on to receive a run is implied for the general case by this existing selectnewtape() code comment: * This is called after finishing a run when we know another run * must be started. This implements steps D3, D4 of Algorithm D. While the previous revision was correct anyway, I tried to explain why it was correct in comments, and soon realized that that was a really bad idea; the rationale was excessively complicated. Allowing 0 tuple runs in rare cases seems like the simplest solution. After all, mergeprereadone() is expressly prepared for 0 tuple runs. It says "ensure that we have at least one tuple, if any are to be had". There is no reason to assume that it says this only because it imagines that no tuples might be found *only after* the first preread for the merge (by which I mean I don't think that only applies when a final on-the-fly merge reloads tuples from one particular tape following running out of tuples of the tape/run in memory). 4. I updated the function beginmerge() to acknowledge an inconsistency for pass-by-value datumsorts, which I mentioned in passing on this thread a few days back. The specific change: @@ -2610,7 +2735,12 @@ beginmerge(Tuplesortstate *state, bool finalMergeBatch) if (finalMergeBatch) { - /* Free outright buffers for tape never actually allocated */ + /* + * Free outright buffers for tape never actually allocated. The + * !state->tuples case is actually entitled to have at least this much + * of a refund ahead of its final merge, but we don't go out of our way + * to marginally improve that case. + */ FREEMEM(state, (state->maxTapes - activeTapes) * TAPE_BUFFER_OVERHEAD); It's not worth worrying about this case, since the savings are small (especially now that maxTapes is capped). But it's worth acknowledging that the "!state->tuples" case is being "short-changed", in the new spirit of heavily scrutinizing where memory goes in tuplesort.c. 5. I updated the "Add MemoryContextStats() calls for debugging" patch. I now formally propose that this debugging instrumentation be committed. This revised debugging instrumentation patch does not have the system report anything about the memory context just because "trace_sort = on". Rather, it does nothing on ordinary builds, where the macro SHOW_MEMORY_STATS will not be defined (it also respects trace_sort). This is about the same approach seen in postgres.c's finish_xact_command(). ISTM that we ought to provide a way of debugging memory use within tuplesort.c, since we now know that that could be very important. Let's not forget where the useful places to look for problems are. 6. Based on your feedback on the batch memory patch (your commit c27033ff), I made a stylistic change. I made similar comments about the newly added quicksort/dumpbatch() MemoryContextReset() call, since it has its own special considerations (a big change in the pattern of allocations occurs after batch memory is used -- we need to be careful about how that could impact the "bucketing by size class"). Thanks -- Peter Geoghegan
Attachment
Hi, I've finally managed to do some benchmarks on the patches. I haven't really studied the details of the patch, so I simply collected a bunch of queries relying on sorting - various forms of SELECT and a few CREATE INDEX commands). It's likely some of the queries can't really benefit from the patch - those should not be positively or negatively affected, though. I've executed the queries on a few basic synthetic data sets with different cardinality 1) unique data 2) hight cardinality (rows/100) 3) low cardinality (rows/1000) initial ordering 1) random 2) sorted 3) almost sorted and different data types 1) int 2) numeric 3) text Tables with and without additional data (padding) were created. So there are quite a few combinations. Attached is a shell script I've used for testing, and also results for 1M and 10M rows on two different machines (one with i5-2500k CPU, the other one with Xeon E5450). Each query was executed 5x for each work_mem value (between 8MB and 1GB), and then a median of the runs was computed and that's what's on the "comparison". This compares a414d96ad2b without (master) and with the patches applied (patched). The last set of columns is simply a "speedup" where "<1.0" means the patched code is faster, while >1.0 means it's slower. Values below 0.9 or 1.1 are using green or red background, to make the most significant improvements or regressions clearly visible. For the smaller data set (1M rows), things works pretty fine. There are pretty much no red cells (so no significant regressions), but quite a few green ones (with duration reduced by up to 50%). There are some results in the 1.0-1.05 range, but considering how short the queries are, I don't think this is a problem. Overall the total duration was reduced by ~20%, which is nice. For the 10M data sets, total speedup is also almost ~20%, and the speedups for most queries are also very nice (often ~50%). But the number of regressions is considerably higher - there's a small number of queries that got significantly slower for multiple data sets, particularly for smaller work_mem values. For example these two queries got almost 2x as slow for some data sets: SELECT a FROM numeric_test UNION SELECT a FROM numeric_test_padding SELECT a FROM text_test UNION SELECT a FROM text_test_padding I assume the slowdown is related to the batching (as it's only happening for low work_mem values), so perhaps there's an internal heuristics that we could tune? I also find it quite interesting that on the i5 machine the CREATE INDEX commands are pretty much not impacted, while on the Xeon machine there's an obvious significant improvement. regards -- Tomas Vondra http://www.2ndQuadrant.com PostgreSQL Development, 24x7 Support, Remote DBA, Training & Services
Attachment
On Tue, Mar 22, 2016 at 2:27 PM, Tomas Vondra <tomas.vondra@2ndquadrant.com> wrote: > Each query was executed 5x for each work_mem value (between 8MB and 1GB), > and then a median of the runs was computed and that's what's on the > "comparison". This compares a414d96ad2b without (master) and with the > patches applied (patched). The last set of columns is simply a "speedup" > where "<1.0" means the patched code is faster, while >1.0 means it's slower. > Values below 0.9 or 1.1 are using green or red background, to make the most > significant improvements or regressions clearly visible. > > For the smaller data set (1M rows), things works pretty fine. There are > pretty much no red cells (so no significant regressions), but quite a few > green ones (with duration reduced by up to 50%). There are some results in > the 1.0-1.05 range, but considering how short the queries are, I don't think > this is a problem. Overall the total duration was reduced by ~20%, which is > nice. > > For the 10M data sets, total speedup is also almost ~20%, and the speedups > for most queries are also very nice (often ~50%). To be clear, you seem to mean that ~50% of the runtime of the query was removed. In other words, the quicksort version is twice as fast. > But the number of > regressions is considerably higher - there's a small number of queries that > got significantly slower for multiple data sets, particularly for smaller > work_mem values. No time to fully consider these benchmarks right now, but: Did you make sure to set replacement_sort_mem very low so that it was never used when patched? And, was this on the latest version of the patch, where memory contexts were reset (i.e. the version that got committed recently)? You said something about memory batching, so ISTM that you should set that to '64', to make sure you don't get one longer run. That might mess with merging. Note that the master branch has the memory batching patch as of a few days back, so it that's the problem at the low end, then that's bad. But I don't think it is: I think that the regressions at the low end are about abbreviated keys, particularly the numeric cases. There is a huge gulf in the cost of those comparisons (abbreviated vs authoritative), and it is legitimately a weakness of the patch that it reduces the number in play. I think it's still well worth it, but it is a downside. There is no reason why the authoritative numeric comparator has to allocate memory, but right now that case isn't optimized I find it weird that the patch is exactly the same as master in a lot of cases. ISTM that with a case where you use 1GB of memory to sort 1 million rows, you're so close to an internal sort that it hardly matters (master will not need a merge step at all, most likely). The patch works best with sorts that take tens of seconds, and I don't think I see any here, nor any high memory tests where RS flops. Now, I think you focused on regressions because that was what was interesting, which is good. I just want to put that in context. Thanks -- Peter Geoghegan
Hi, On 03/22/2016 11:07 PM, Peter Geoghegan wrote: > On Tue, Mar 22, 2016 at 2:27 PM, Tomas Vondra > <tomas.vondra@2ndquadrant.com> wrote: >> Each query was executed 5x for each work_mem value (between 8MB and 1GB), >> and then a median of the runs was computed and that's what's on the >> "comparison". This compares a414d96ad2b without (master) and with the >> patches applied (patched). The last set of columns is simply a "speedup" >> where "<1.0" means the patched code is faster, while >1.0 means it's slower. >> Values below 0.9 or 1.1 are using green or red background, to make the most >> significant improvements or regressions clearly visible. >> >> For the smaller data set (1M rows), things works pretty fine. There are >> pretty much no red cells (so no significant regressions), but quite a few >> green ones (with duration reduced by up to 50%). There are some results in >> the 1.0-1.05 range, but considering how short the queries are, I don't think >> this is a problem. Overall the total duration was reduced by ~20%, which is >> nice. >> >> For the 10M data sets, total speedup is also almost ~20%, and the speedups >> for most queries are also very nice (often ~50%). > > To be clear, you seem to mean that ~50% of the runtime of the query > was removed. In other words, the quicksort version is twice as fast. Yes, that's what I meannt. Sorry for the inaccuracy. > >> But the number of regressions is considerably higher - there's a >> small number of queries that got significantly slower for multiple >> data sets, particularly for smaller work_mem values. > > No time to fully consider these benchmarks right now, but: Did you > make sure to set replacement_sort_mem very low so that it was never > used when patched? And, was this on the latest version of the patch, > where memory contexts were reset (i.e. the version that got > committed recently)? You said something about memory batching, so > ISTM that you should set that to '64', to make sure you don't get one > longer run. That might mess with merging. I've tested the patch you've sent on 2016/3/11, which I believe is the last version. I haven't tuned the replacement_sort_mem at all. because my understanding was that it's not a 9.6 material (per your message). So my intent was to test the configuration people are likely to use by default. I'm not sure about the batching - that was merely a guess of what might be the problem. > > Note that the master branch has the memory batching patch as of a > few days back, so it that's the problem at the low end, then that's > bad. I'm not sure which commit are you referring to. The benchmark was done on a414d96a (from 2016/3/10). However I'd expect that to affect both sets of measurements, although it's possible that it affects the patched version differently. > But I don't think it is: I think that the regressions at the low end > are about abbreviated keys, particularly the numeric cases. There is > a huge gulf in the cost of those comparisons (abbreviated vs > authoritative), and it is legitimately a weakness of the patch that > it reduces the number in play. I think it's still well worth it, but > it is a downside. There is no reason why the authoritative numeric > comparator has to allocate memory, but right now that case isn't > optimized. Yes, numeric and text are the most severely affected cases. > > I find it weird that the patch is exactly the same as master in a > lot of cases. ISTM that with a case where you use 1GB of memory to > sort 1 million rows, you're so close to an internal sort that it > hardly matters (master will not need a merge step at all, most > likely). The patch works best with sorts that take tens of seconds, > and I don't think I see any here, nor any high memory tests where RS > flops. Now, I think you focused on regressions because that was what > was interesting, which is good. I just want to put that in context. I don't think the tests on 1M rows are particularly interesting, and I don't see any noticeable regressions there. Perhaps you mean the tests on 10M rows instead? Yes, you're correct - I was mostly looking for regressions. However, the worst cases of regressions are on relatively long sorts, e.g. slowing down from 35 seconds to 64 seconds, etc. So that's quite long, and it's surely using non-trivial amount of memory. Or am I missing something? regards -- Tomas Vondra http://www.2ndQuadrant.com PostgreSQL Development, 24x7 Support, Remote DBA, Training & Services
On Tue, Mar 22, 2016 at 3:35 PM, Tomas Vondra <tomas.vondra@2ndquadrant.com> wrote: > I've tested the patch you've sent on 2016/3/11, which I believe is the last > version. I haven't tuned the replacement_sort_mem at all. because my > understanding was that it's not a 9.6 material (per your message). So my > intent was to test the configuration people are likely to use by default. I meant that using replacement selection in a special way with CREATE INDEX was not 9.6 material. But replacement_sort_mem is. And so, any case with the (maintenance)_work_mem <= 16MB will have used a heap for the first run. I'm sorry I did not make a point of telling you this. It's my fault. The result in any case is that pre-sorted cases will be similar with and without the patch, since replacement selection can thereby make one long run. But on non-sorted cases, the patch helps less because it is in use less -- with not so much data overall, possibly much less (which I think explains why the 1M row tests seem so much less interesting than the 10M row tests). I worry that at the low end, replacement_sort_mem makes the patch have one long run, but still some more other runs, so merging is unbalanced. We should consider if the patch can beat the master branch at the low end without using a replacement selection heap. It would do better in at least some cases in low memory conditions, possibly a convincing majority of cases. I had hoped that my recent idea (since committed) of resetting memory contexts would help a lot with regressions when work_mem is very low, and that particular theory isn't really tested here. > I'm not sure which commit are you referring to. The benchmark was done on > a414d96a (from 2016/3/10). However I'd expect that to affect both sets of > measurements, although it's possible that it affects the patched version > differently. You did test the right patches. It just so happens that the master branch now has the memory batching stuff now, so it doesn't get credited with that. I think this is good, though, because we care about 9.5 -> 9.6 regressions. Improvement ratio (master time/patched time) for Xeon 10 million row case "SELECT * FROM int_test_padding ORDER BY a DESC": For work_mem of 8MB = 0.83, 32MB = 0.62, 128MB = 0.52, 512MB = 0.47, 1024MB = 1.00 So, it gets faster than the master branch as more memory is available, but then it goes to 1.00 -- a perfect draw. I think that this happened simply because at that point, the sort was an internal sort (even though similar CREATE INDEX case did not go internal at the same point). The (internal) 1024MB case is not that much faster than the 512MB external case, which is pretty good. There are also "near draws", where the ratio is 0.98 or so. I think that this is because abbreviation is aborted, which can be a problem with synthetic data + text -- you get a very slow sort either way, where most time is spent calling strcoll(), and cache characteristics matter much less. Those cases seemingly take much longer overall, so this theory makes sense. Unfortunately, abbreviated keys for text that is not C locale text was basically disabled across the board today due to a glibc problem. :-( Whenever I see that the patch is exactly as fast as the master branch, I am skeptical. I am particularly skeptical of all i5 results (including 10M cases), because the patch seems to be almost perfectly matched to the master branch for CREATE INDEX cases (which are the best cases for the patch on your Xeon server) -- it's much easier to believe that there was a problem during the test, honestly, like maintenance_work_mem wasn't set correctly. Those two things are so different that I have a hard time imagining that they'd ever really draw. I mean, it's possible, but it's more likely to be a problem with testing. And, queries like "SELECT * FROM int_test_padding ORDER BY a DESC" return all rows, which adds noise from all the client overhead. In fact, you often see that adding more memory helps no case here, so it seem a bit pointless. Maybe they should be written like "SELECT * FROM (select * from int_test_padding ORDER BY a DESC OFFSET 1e10) ff" instead. And maybe queries like "SELECT DISTINCT a FROM int_test ORDER BY a" would be better as "SELECT COUNT(DISTINCT a) FROM int_test", in order to test the datum/aggregate case. Just suggestions. If you really wanted to make the patch look good, a sort with 5GB of work_mem is the best way, FWIW. The heap data structure used by the master branch tuplesort.c will handle that very badly. You use no temp_tablespaces here. I wonder if the patch would do better with that. Sorting can actually be quite I/O bound with the patch sometimes, where it's usually CPU/memory bound with the heap, especially with lots of work_mem. More importantly, it would be more informative if the temp_tablespace was not affected by I/O from Postgres' heap. I also like seeing a sample of "trace_sort = on" output. I don't expect you to carefully collect that in every case, but it can tell us a lot about what's really going on when benchmarking. Thanks -- Peter Geoghegan
On Tue, Mar 22, 2016 at 2:27 PM, Tomas Vondra <tomas.vondra@2ndquadrant.com> wrote: > For example these two queries got almost 2x as slow for some data sets: > > SELECT a FROM numeric_test UNION SELECT a FROM numeric_test_padding > SELECT a FROM text_test UNION SELECT a FROM text_test_padding > > I assume the slowdown is related to the batching (as it's only happening for > low work_mem values), so perhaps there's an internal heuristics that we > could tune? Can you show trace_sort output for these cases? Both master, and patched? Thanks -- Peter Geoghegan
Hi, On 03/24/2016 03:00 AM, Peter Geoghegan wrote: > On Tue, Mar 22, 2016 at 3:35 PM, Tomas Vondra > <tomas.vondra@2ndquadrant.com> wrote: >> I've tested the patch you've sent on 2016/3/11, which I believe is the last >> version. I haven't tuned the replacement_sort_mem at all. because >> my understanding was that it's not a 9.6 material (per your >> message). So my intent was to test the configuration people are >> likely to use by default. > > I meant that using replacement selection in a special way with > CREATE INDEX was not 9.6 material. But replacement_sort_mem is. And > so, any case with the (maintenance)_work_mem <= 16MB will have used a > heap for the first run. FWIW, maintenance_work_mem was set to 1GB on the i5 machine and 256MB on the Xeon. Hmm, maybe that's why we see no difference for CREATE INDEX on the i5, and an improvement on the Xeon. > > I'm sorry I did not make a point of telling you this. It's my fault. > The result in any case is that pre-sorted cases will be similar with > and without the patch, since replacement selection can thereby make > one long run. But on non-sorted cases, the patch helps less because > it is in use less -- with not so much data overall, possibly much > less (which I think explains why the 1M row tests seem so much less > interesting than the 10M row tests). Not a big deal - it's easy enough to change the config and repeat the benchmark. Are there any particular replacement_sort_mem values that you think would be interesting to configure? I have to admit I'm a bit afraid we'll introduce a new GUC that only very few users will know how to set properly, and so most people will run with the default value or set it to something stupid. > > I worry that at the low end, replacement_sort_mem makes the patch > have one long run, but still some more other runs, so merging is > unbalanced. We should consider if the patch can beat the master > branch at the low end without using a replacement selection heap. It > would do better in at least some cases in low memory conditions, > possibly a convincing majority of cases. I had hoped that my recent > idea (since committed) of resetting memory contexts would help a lot > with regressions when work_mem is very low, and that particular > theory isn't really tested here. Are you saying none of the queries triggers the memory context resets? What queries would trigger that (to test the theory)? > >> I'm not sure which commit are you referring to. The benchmark was >> done on a414d96a (from 2016/3/10). However I'd expect that to >> affect both sets of measurements, although it's possible that it >> affects the patched version differently. > > You did test the right patches. It just so happens that the master > branch now has the memory batching stuff now, so it doesn't get > credited with that. I think this is good, though, because we care > about 9.5 -> 9.6 regressions. So there's a commit in master (but not in 9.5), adding memory batching, but it got committed before a414d96a so the benchmark does not measure it's impact (with respect to 9.5). Correct? But if we care about 9.5 -> 9.6 regressions, then perhaps we should include that commit into the benchmark, because that's what the users will see? Or have I misunderstood the second part? BTW which patch does the memory batching? A quick search through git log did not return any recent patches mentioning these terms. > Improvement ratio (master time/patched time) for Xeon 10 million row > case "SELECT * FROM int_test_padding ORDER BY a DESC": > > For work_mem of 8MB = 0.83, 32MB = 0.62, 128MB = 0.52, 512MB = 0.47, > 1024MB = 1.00 > > So, it gets faster than the master branch as more memory is > available, but then it goes to 1.00 -- a perfect draw. I think that > this happened simply because at that point, the sort was an internal > sort (even though similar CREATE INDEX case did not go internal at > the same point). The (internal) 1024MB case is not that much faster > than the 512MB external case, which is pretty good. Indeed. > > There are also "near draws", where the ratio is 0.98 or so. I think > that this is because abbreviation is aborted, which can be a problem > with synthetic data + text -- you get a very slow sort either way, That is possible, yes. It's true that the worst regressions are on text, although there are a few on numeric too (albeit not as significant). > where most time is spent calling strcoll(), and cache > characteristics matter much less. Those cases seemingly take much > longer overall, so this theory makes sense. Unfortunately, > abbreviated keys for text that is not C locale text was basically > disabled across the board today due to a glibc problem. :-( Yeah. Bummer :-( > > Whenever I see that the patch is exactly as fast as the master > branch, I am skeptical. I am particularly skeptical of all i5 > results (including 10M cases), because the patch seems to be almost > perfectly matched to the master branch for CREATE INDEX cases (which > are the best cases for the patch on your Xeon server) -- it's much > easier to believe that there was a problem during the test, honestly, > like maintenance_work_mem wasn't set correctly. Those two things are As I mentioned above, I haven't realized work_mem does not matter for CREATE INDEX, and maintenance_work_mem was set to a fixed value for the whole test. And the two machines used different values for this particular configuration value - Xeon used just 256MB, while i5 used 1GB. So while on i5 it was just a single chunk, on Xeon there were multiple batches. Hence the different behavior. > so different that I have a hard time imagining that they'd ever > really draw. I mean, it's possible, but it's more likely to be a > problem with testing. And, queries like "SELECT * FROM > int_test_padding ORDER BY a DESC" return all rows, which adds noise > from all the client overhead. In fact, you often see that adding more No it doesn't add overhead. The script actually does COPY (query) TO '/dev/null' on the server for all queries (except for the CREATE INDEX, obviously), so there should be pretty much no overhead due to transferring rows to the client and so on. > memory helps no case here, so it seem a bit pointless. Maybe they > should be written like "SELECT * FROM (select * from int_test_padding > ORDER BY a DESC OFFSET 1e10) ff" instead. And maybe queries like > "SELECT DISTINCT a FROM int_test ORDER BY a" would be better as > "SELECT COUNT(DISTINCT a) FROM int_test", in order to test the > datum/aggregate case. Just suggestions. I believe the 'copy to /dev/null' achieves the same thing. > > If you really wanted to make the patch look good, a sort with 5GB of > work_mem is the best way, FWIW. The heap data structure used by the > master branch tuplesort.c will handle that very badly. You use no > temp_tablespaces here. I wonder if the patch would do better with > that. Sorting can actually be quite I/O bound with the patch > sometimes, where it's usually CPU/memory bound with the heap, > especially with lots of work_mem. More importantly, it would be more > informative if the temp_tablespace was not affected by I/O from > Postgres' heap. I'll consider testing that. However, I don't think there was any significant I/O on the machines - particularly not on the Xeon, which has 16GB of RAM. So the temp files should fit into that quite easily. The i5 machine has only 8GB of RAM, but it has 6 SSD drives in raid0. So I doubt it was I/O bound. > > I also like seeing a sample of "trace_sort = on" output. I don't > expect you to carefully collect that in every case, but it can tell > us a lot about what's really going on when benchmarking. Sure, I can collect that. regards -- Tomas Vondra http://www.2ndQuadrant.com PostgreSQL Development, 24x7 Support, Remote DBA, Training & Services
On Wed, Mar 23, 2016 at 8:05 PM, Tomas Vondra <tomas.vondra@2ndquadrant.com> wrote: > FWIW, maintenance_work_mem was set to 1GB on the i5 machine and 256MB on the > Xeon. Hmm, maybe that's why we see no difference for CREATE INDEX on the i5, > and an improvement on the Xeon. That would explain it. > Not a big deal - it's easy enough to change the config and repeat the > benchmark. Are there any particular replacement_sort_mem values that you > think would be interesting to configure? I would start with replacement_sort_mem=64. i.e., 64KB, effectively disabled > I have to admit I'm a bit afraid we'll introduce a new GUC that only very > few users will know how to set properly, and so most people will run with > the default value or set it to something stupid. I agree. > Are you saying none of the queries triggers the memory context resets? What > queries would trigger that (to test the theory)? They will still do the context resetting and so on just the same, but would use a heap for the first attempt. But replacement_sort_mem=64 would let us know that > But if we care about 9.5 -> 9.6 regressions, then perhaps we should include > that commit into the benchmark, because that's what the users will see? Or > have I misunderstood the second part? I think it's good that you didn't test the March 17 commit of the memory batching to the master branch when testing the master branch. You should continue to do that, because we care about regressions against 9.5 only. The only issue insofar as what code was tested is that replacement_sort_mem was not set to 64 (to effectively disable any use of the heap by the patch). I would like to see if we can get rid of replacement_sort_mem without causing any real regressions, which I think the memory context reset stuff makes possible. There was a new version of my quicksort patch posted after March 17, but don't worry about it -- that's totally cosmetic. Some minor tweaks. > BTW which patch does the memory batching? A quick search through git log did > not return any recent patches mentioning these terms. Commit 0011c0091e886b874e485a46ff2c94222ffbf550. But, like I said, avoid changing what you're testing as master; do not include that. The patch set you were testing is fine. Nothing is missing. > As I mentioned above, I haven't realized work_mem does not matter for CREATE > INDEX, and maintenance_work_mem was set to a fixed value for the whole test. > And the two machines used different values for this particular configuration > value - Xeon used just 256MB, while i5 used 1GB. So while on i5 it was just > a single chunk, on Xeon there were multiple batches. Hence the different > behavior. Makes sense. Obviously this should be avoided, though. > No it doesn't add overhead. The script actually does > > COPY (query) TO '/dev/null' > > on the server for all queries (except for the CREATE INDEX, obviously), so > there should be pretty much no overhead due to transferring rows to the > client and so on. That still adds overhead, because the output functions are still used to create a textual representation of data. This was how Andres tested the improvement to the timestamptz output function committed to 9.6, for example. >> If you really wanted to make the patch look good, a sort with 5GB of >> work_mem is the best way, FWIW. The heap data structure used by the >> master branch tuplesort.c will handle that very badly. You use no >> temp_tablespaces here. I wonder if the patch would do better with >> that. Sorting can actually be quite I/O bound with the patch >> sometimes, where it's usually CPU/memory bound with the heap, >> especially with lots of work_mem. More importantly, it would be more >> informative if the temp_tablespace was not affected by I/O from >> Postgres' heap. > > > I'll consider testing that. However, I don't think there was any significant > I/O on the machines - particularly not on the Xeon, which has 16GB of RAM. > So the temp files should fit into that quite easily. Right, but with a bigger sort, there might well be more I/O. Especially for the merge. It might be that that holds back the patch from doing even better than the master branch does. > The i5 machine has only 8GB of RAM, but it has 6 SSD drives in raid0. So I > doubt it was I/O bound. These patches can sometimes be significantly I/O bound on my laptop, where that didn't happen before. Sounds unlikely here, though. >> I also like seeing a sample of "trace_sort = on" output. I don't >> expect you to carefully collect that in every case, but it can tell >> us a lot about what's really going on when benchmarking. > > > Sure, I can collect that. Just for the interesting cases. Or maybe just dump it all and let me figure it out for myself. trace_sort output shows me how many runs they are, how abbreviation did, how memory was used, and even if the sort was I/O bound at various stages (it dumps some getrusage stats to the log, too). You can usually tell exactly what happened for external sorts, which is very interesting for those one or two cases that you found to be noticeably worse off with the patch. Thanks for testing! -- Peter Geoghegan
On Sun, Mar 20, 2016 at 11:01 PM, Peter Geoghegan <pg@heroku.com> wrote: > Allowing 0 tuple runs in rare cases seems like the simplest solution. > After all, mergeprereadone() is expressly prepared for 0 tuple runs. > It says "ensure that we have at least one tuple, if any are to be > had". There is no reason to assume that it says this only because it > imagines that no tuples might be found *only after* the first preread > for the merge (by which I mean I don't think that only applies when a > final on-the-fly merge reloads tuples from one particular tape > following running out of tuples of the tape/run in memory). I just realized that there is what amounts to an over-zealous assertion in dumpbatch(): > + * When this edge case hasn't occurred, the first memtuple should not > + * be found to be heapified (nor should any other memtuple). > + */ > + Assert(state->memtupcount == 0 || > + state->memtuples[0].tupindex == HEAP_RUN_NEXT); The problem is that state->memtuples[0].tupindex won't have been *reliably* initialized here. We could make sure that it is for the benefit of this assertion, but I think it would be better to just remove the assertion, which isn't testing very much over and above the similar assertions that appears in the only dumpbatch() caller, dumptuples(). -- Peter Geoghegan
On Thu, Mar 10, 2016 at 6:54 PM, Peter Geoghegan <pg@heroku.com> wrote: > I've used amcheck [2] to test this latest revision -- the tool ought > to not see any problems with any index created with the patch applied. > Reviewers might find it helpful to use amcheck, too. As 9.6 is > stabilized, I anticipate that amcheck will give us a fighting chance > at early detection of any bugs that might have slipped into tuplesort, > or a B-Tree operator class. Since we still don't even have one single > test of the external sort code [3], it's just as well. If we wanted to > test external sorting, maybe we'd do that by adding tests to amcheck, > that are not run by default, much like test_decoding, which tests > logical decoding but is not targeted by "make installcheck"; that > would allow the tests to be fairly comprehensive without being > annoying. Using amcheck neatly side-steps issues with the portability > of "expected" pg_regress output when collatable type sorting is > tested. Note that amcheck V2, which I posted just now features tests for external sorting. The way these work requires discussion. The tests are motivated in part by the recent strxfrm() debacle, as well as by the need to have at least some test coverage for this patch. It's bad that external sorting currently has no test coverage. We should try and do better there as part of this overhaul to tuplesort.c. Thanks -- Peter Geoghegan
On Mon, Mar 28, 2016 at 11:18 PM, Peter Geoghegan <pg@heroku.com> wrote: > Note that amcheck V2, which I posted just now features tests for > external sorting. The way these work requires discussion. The tests > are motivated in part by the recent strxfrm() debacle, as well as by > the need to have at least some test coverage for this patch. It's bad > that external sorting currently has no test coverage. We should try > and do better there as part of this overhaul to tuplesort.c. Test coverage is good! However, I don't see that you've responded to Tomas Vondra's report of regressions. Maybe you're waiting for more data from him, but we're running out of time here. I think what we need to decide is whether these results are bad enough that the patch needs more work on the regressed cases, or whether we're comfortable with some regressions in low-memory configurations for the benefit of higher-memory configurations. I'm kind of on the fence about that, myself. One test that kind of bothers me in particular is the "SELECT DISTINCT a FROM numeric_test ORDER BY a" test on the high_cardinality_random data set. That's a wash at most work_mem values, but at 32MB it's more than 3x slower. That's very strange, and there are a number of other results like that, where one particular work_mem value triggers a large regression. That's worrying. Also, it's pretty clear that the patch has more large wins than it does large losses, but it seems pretty easy to imagine people who haven't tuned any GUCs writing in to say that 9.6 is way slower on their workload, because those people are going to be at work_mem=4MB, maintenance_work_mem=64MB. At those numbers, if Tomas's data is representative, it's not hard to imagine that the number of people who see a significant regression might be quite a bit larger than the number who see a significant speedup. On the whole, I'm tempted to say this needs more work before we commit to it, but I'd like to hear other opinions on that point. -- Robert Haas EnterpriseDB: http://www.enterprisedb.com The Enterprise PostgreSQL Company
On Tue, Mar 29, 2016 at 9:11 AM, Robert Haas <robertmhaas@gmail.com> wrote: > One test that kind of bothers me in particular is the "SELECT DISTINCT > a FROM numeric_test ORDER BY a" test on the high_cardinality_random > data set. That's a wash at most work_mem values, but at 32MB it's > more than 3x slower. That's very strange, and there are a number of > other results like that, where one particular work_mem value triggers > a large regression. That's worrying. That case is totally invalid as a benchmark for this patch. Here is the query plan I get (doesn't matter if I run analyze) when I follow Tomas' high_cardinality_random 10M instructions (including setting work_mem to 32MB): postgres=# explain analyze select distinct a from numeric_test order by a; QUERY PLAN ───────────────────────────────────────────────────────────────────────────────────────────────────────────────────────────────────────Sort (cost=268895.39..270373.10 rows=591082 width=8) (actual time=3907.917..4086.174 rows=999879 loops=1) Sort Key: a Sort Method: external merge Disk: 18536kB -> HashAggregate (cost=206320.50..212231.32 rows=591082 width=8) (actual time=3109.619..3387.599 rows=999879 loops=1) Group Key: a -> Seq Scan on numeric_test (cost=0.00..175844.40 rows=12190440 width=8) (actual time=0.025..601.295 rows=10000000 loops=1)Planning time: 0.088 msExecution time: 4120.656 ms (8 rows) Does that seem like a fair test of this patch? I must also point out an inexplicable differences between the i5 and Xeon in relation to this query. It took about took 10% less time on the patched Xeon 10M case, not ~200% more (line 53 of the summary page in each 10M case). So even if this case did exercise the patch well, it's far from clear that it has even been regressed at all. It's far easier to imagine that there was some problem with the i5 tests. A complete do-over from Tomas would be best, here. He has already acknowledged that the i5 CREATE INDEX results were completely invalid. Pending a do-over from Tomas, I recommend ignoring the i5 tests completely. Also, I should once again point out that many of the work_mem cases actually had internal sorts at the high end, so once the code in the patches simply wasn't exercised at all at the high end (the 1024MB cases, where the numbers might be expected to get really good). If there is ever a regression, it is only really sensible to talk about it while looking at trace_sort output (and, I guess, the query plan). I've asked Tomas for trace_sort output in all relevant cases. There is no point in "flying blind" and speculating what the problem was from a distance. > Also, it's pretty clear that the patch has more large wins than it > does large losses, but it seems pretty easy to imagine people who > haven't tuned any GUCs writing in to say that 9.6 is way slower on > their workload, because those people are going to be at work_mem=4MB, > maintenance_work_mem=64MB. At those numbers, if Tomas's data is > representative, it's not hard to imagine that the number of people who > see a significant regression might be quite a bit larger than the > number who see a significant speedup. I don't think they are representative. Greg Stark characterized the regressions as being fairly limited, mostly at the very low end. And that was *before* all the memory fragmentation stuff made that better. I haven't done any analysis of how much better that made the problem *across the board* yet, but for int4 cases I could make 1MB work_mem queries faster with gigabytes of data on my laptop. I believe I tested various datum sort cases there, like "select count(distinct(foo)) from bar"; those are a very pure test of the patch. -- Peter Geoghegan
On Tue, Mar 29, 2016 at 12:43 PM, Peter Geoghegan <pg@heroku.com> wrote: > A complete do-over from Tomas would be best, here. He has already > acknowledged that the i5 CREATE INDEX results were completely invalid. The following analysis is all based on Xeon numbers, which as I've said we should focus on pending a do-over from Tomas. Especially important here is the larget set -- the 10M numbers from results-xeon-10m.ods. I think that abbreviation distorts things here. We also see distortion from "padding" cases. Rather a lot of "padding" is used, FWIW. From Tomas' script: INSERT INTO numeric_test_padding SELECT a, repeat(md5(a::text),10) FROM data_float ORDER BY a; This makes the tests have TOAST overhead. Some important observations on results-xeon-10m: * There are almost no regressions for types that don't use abbreviation. There might be one exception when there is both padding and presorted input -- the 32MB high_cardinality_almost_asc/high_cardinality_sorted/unique_sorted "SELECT * FROM int_test_padding ORDER BY a", which takes 26% - 35% longer (those are all basically the same cases). But it's a big win in the high_cardinality_random, unique_random, and even unique_almost_asc categories, or when DESC order was requested in all categories (I note that there is certainly an emphasis on pre-sorted cases in the choice of categories). Other than that, no regressions from non-abbreviated types. * No CREATE INDEX case is ever appreciably regressed, even with maintenance_work_mem at 8MB, 1/4 of its default value of 64MB. (Maybe we lose 1% - 3% with the other (results-xeon-1m.ods) cases, where maintenance_work_mem is close to or actually high enough to get an internal sort). It's a bit odd that "CREATE INDEX x ON text_test_padding (a)" is about a wash for high_cardinality_almost_asc, but I think that's just because we're super I/O bound for this presorted case, but cannot make up for it with quicksort's "bubble sort best case" precheck for presortedness, so replacement selection does better in a way that might even result in a clean draw. CREATE INDEX looks very good in general. I think abbreviation might abort in one or two cases for text, but the picture for the patch is still solid. * "Padding" can really distort low-end cases, that become more about moving big tuples around than actual sorting. If you really want to see how high_cardinality_almost_asc queries like "SELECT * FROM text_test_padding ORDER BY a" are testing the wrong thing, consider the best and worst case for the master branch with any amount of work_mem. The 10 million tuple high_cardinality_almost_asc case takes 40.16 seconds, 39.95 seconds, 40.98 seconds, and 41.28 seconds, and 42.1 seconds for respective work_mems of 8MB, 32MB, 128MB, 512MB, and 1024MB. This is a very narrow case because it totally deemphasizes comparison cost and emphasizes moving tuples around, involves abbreviation of text with a merge phase that cannot use abbreviation that only the patch has due to RS best-case on master. The case is seriously short changed by the memory batching refund thing in practice. When is *high cardinality text* (not dates or something) ever likely to be found in pre-sorted order for 10 million tuples in the real world? Besides, we just stopped trusting strxfrm(), so the case would probably be a wash now at worst. * The more plausible padding + presorted + abbreviation case that is sometimes regressed is "SELECT * FROM numeric_test_padding ORDER BY a". But that's regressed a lot less than the aforementioned "SELECT * FROM text_test_padding ORDER BY a" case, and only at the low end. It is sometimes faster where the original case I mentioned is slower. * Client overhead may distort things in the case of queries like "SELECT * FROM foo ORDER BY bar". This could be worse for the patch, which does relatively more computation during the final on-the-fly merge phase (which is great when you can overlap that with I/O; perhaps not when you get more icache misses with other computation). Aside from just adding a lot of noise, this could unfairly make the patch look a lot worse than master. Now, I'm not saying all of this doesn't matter. But these are all fairly narrow, pathological cases, often more about moving big tuples around (in memory and on disk) than about sorting. These regressions are well worth it. I don't think I can do any more than I already have to fix these cases; it may be impossible. It's a very difficult thing to come up with an algorithm that's unambiguously better in every possible case. I bent over backwards to fix low-end regressions already. In memory rich environments with lots of I/O bandwidth, I've seen this patch make CREATE INDEX ~2.5x faster for int4, on a logged table. More importantly, the patch makes setting maintenance_work_mem easy. Users' intuition for how sizing it ought to work now becomes more or less correct: In general, for each individual utility command bound by maintenance_work_mem, more memory is better. That's the primary value in having tuple sorting be cache oblivious for us; the smooth cost function of sorting makes tuning relatively easy, and gives us a plausible path towards managing local memory for sorting and hashing dynamically for the entire system. I see no other way for us to get there. -- Peter Geoghegan
Hi, On 03/29/2016 09:43 PM, Peter Geoghegan wrote: > On Tue, Mar 29, 2016 at 9:11 AM, Robert Haas <robertmhaas@gmail.com> wrote: >> One test that kind of bothers me in particular is the "SELECT DISTINCT >> a FROM numeric_test ORDER BY a" test on the high_cardinality_random >> data set. That's a wash at most work_mem values, but at 32MB it's >> more than 3x slower. That's very strange, and there are a number of >> other results like that, where one particular work_mem value triggers >> a large regression. That's worrying. > > That case is totally invalid as a benchmark for this patch. Here is > the query plan I get (doesn't matter if I run analyze) when I follow > Tomas' high_cardinality_random 10M instructions (including setting > work_mem to 32MB): > > postgres=# explain analyze select distinct a from numeric_test order by a; > QUERY > PLAN > ─────────────────────────────────────────────────────────────────────────────────────────────────────────────────────────────────────── > Sort (cost=268895.39..270373.10 rows=591082 width=8) (actual > time=3907.917..4086.174 rows=999879 loops=1) > Sort Key: a > Sort Method: external merge Disk: 18536kB > -> HashAggregate (cost=206320.50..212231.32 rows=591082 width=8) > (actual time=3109.619..3387.599 rows=999879 loops=1) > Group Key: a > -> Seq Scan on numeric_test (cost=0.00..175844.40 > rows=12190440 width=8) (actual time=0.025..601.295 rows=10000000 > loops=1) > Planning time: 0.088 ms > Execution time: 4120.656 ms > (8 rows) > > Does that seem like a fair test of this patch? And why not? I mean, why should it be acceptable to slow down? > > I must also point out an inexplicable differences between the i5 and > Xeon in relation to this query. It took about took 10% less time on > the patched Xeon 10M case, not ~200% more (line 53 of the summary page > in each 10M case). So even if this case did exercise the patch well, > it's far from clear that it has even been regressed at all. It's far > easier to imagine that there was some problem with the i5 tests. That may be easily due to differences between the CPUs and configuration. For example the Xeon uses a way older CPU with different amounts of CPU cache, and it's also a multi-socket system. And so on. > A complete do-over from Tomas would be best, here. He has already > acknowledged that the i5 CREATE INDEX results were completely invalid. > Pending a do-over from Tomas, I recommend ignoring the i5 tests > completely. Also, I should once again point out that many of the > work_mem cases actually had internal sorts at the high end, so once > the code in the patches simply wasn't exercised at all at the high end > (the 1024MB cases, where the numbers might be expected to get really > good). > > If there is ever a regression, it is only really sensible to talk > about it while looking at trace_sort output (and, I guess, the query > plan). I've asked Tomas for trace_sort output in all relevant cases. > There is no point in "flying blind" and speculating what the problem > was from a distance. The updated benchmarks are currently running. I'm out of office until Friday, and I'd like to process the results over the weekend. FWIW I'll have results for these cases: 1) unpatched (a414d96a) 2) patched, default settings 3) patched, replacement_sort_mem=64 Also, I'll have trace_sort=on output for all the queries, so we can investigate further. > >> Also, it's pretty clear that the patch has more large wins than it >> does large losses, but it seems pretty easy to imagine people who >> haven't tuned any GUCs writing in to say that 9.6 is way slower on >> their workload, because those people are going to be at >> work_mem=4MB, maintenance_work_mem=64MB. At those numbers, if >> Tomas's data is representative, it's not hard to imagine that the >> number of people who see a significant regression might be quite a >> bit larger than the number who see a significant speedup. Yeah. That was one of the goals of the benchmark, to come up with some tuning recommendations. On some systems significantly increasing memory GUCs may not be possible, though - say, on very small systems with very limited amounts of RAM. > > I don't think they are representative. Greg Stark characterized the > regressions as being fairly limited, mostly at the very low end. And > that was *before* all the memory fragmentation stuff made that > better. I haven't done any analysis of how much better that made the > problem *across the board* yet, but for int4 cases I could make 1MB > work_mem queries faster with gigabytes of data on my laptop. I > believe I tested various datum sort cases there, like "select > count(distinct(foo)) from bar"; those are a very pure test of the > patch. > Well, I'd guess those conclusions may be a bit subjective. regards -- Tomas Vondra http://www.2ndQuadrant.com PostgreSQL Development, 24x7 Support, Remote DBA, Training & Services
On Tue, Mar 29, 2016 at 6:02 PM, Tomas Vondra <tomas.vondra@2ndquadrant.com> wrote: > And why not? I mean, why should it be acceptable to slow down? My point was that over 80% of execution time was spent in the HashAggregate, which outputs tuples to the sort. That, and the huge i5/Xeon inconsistency (in the extent to which this is regressed -- it's not at all, or it's regressed a lot) makes me suspicious that there is something else going on. Possibly involving the scheduling of I/O. > That may be easily due to differences between the CPUs and configuration. > For example the Xeon uses a way older CPU with different amounts of CPU > cache, and it's also a multi-socket system. And so on. We're talking about a huge relative difference with that HashAggregate plan, though. I don't think that those relative differences are explained by differing CPU characteristics. But I guess we'll find out soon enough. >> If there is ever a regression, it is only really sensible to talk >> about it while looking at trace_sort output (and, I guess, the query >> plan). I've asked Tomas for trace_sort output in all relevant cases. >> There is no point in "flying blind" and speculating what the problem >> was from a distance. > > > The updated benchmarks are currently running. I'm out of office until > Friday, and I'd like to process the results over the weekend. FWIW I'll have > results for these cases: > > 1) unpatched (a414d96a) > 2) patched, default settings > 3) patched, replacement_sort_mem=64 > > Also, I'll have trace_sort=on output for all the queries, so we can > investigate further. Thanks! That will tell us a lot more. > Yeah. That was one of the goals of the benchmark, to come up with some > tuning recommendations. On some systems significantly increasing memory GUCs > may not be possible, though - say, on very small systems with very limited > amounts of RAM. Fortunately, such systems will probably mostly use external sorts for CREATE INDEX cases, and there seems to be very little if any downside there, at least according to your similarly, varied tests of CREATE INDEX. >> I don't think they are representative. Greg Stark characterized the >> regressions as being fairly limited, mostly at the very low end. And >> that was *before* all the memory fragmentation stuff made that >> better. I haven't done any analysis of how much better that made the >> problem *across the board* yet, but for int4 cases I could make 1MB >> work_mem queries faster with gigabytes of data on my laptop. I >> believe I tested various datum sort cases there, like "select >> count(distinct(foo)) from bar"; those are a very pure test of the >> patch. >> > > Well, I'd guess those conclusions may be a bit subjective. I think that the conclusion that we should do something or not do something based on this information is subjective. OTOH, whether and to what extent these tests are representative of real user workloads seems much less subjective. This is not a criticism of the test cases you came up with, which rightly emphasized possibly regressed cases. I think everyone already understood that the picture was very positive at the high end, in memory rich environments. -- Peter Geoghegan
On Tue, Mar 29, 2016 at 6:02 PM, Tomas Vondra <tomas.vondra@2ndquadrant.com> wrote: > That may be easily due to differences between the CPUs and configuration. > For example the Xeon uses a way older CPU with different amounts of CPU > cache, and it's also a multi-socket system. And so on. So, having searched past threads I guess this was your Xeon E5450, which has a 12MB cache. I also see that you have an Intel Core i5-2500K Processor, which has 6MB of L2 cache. This hardware is mid-end, and the CPUs were discontinued in 2010 and 2013 respectively. Now, the i5 has a smaller L2 cache, so if anything I'd expect it to do worse than the Xeon, not better. But leaving that aside, I think there is an issue that we don't want to lose sight of. Which is: In most of the regressions we were discussing today, perhaps the entire heap structure can fit in L2 cache. This would be true for stuff like int4 CREATE INDEX builds, where a significant fraction of memory is used for IndexTuples, which most or all comparisons don't have to read in memory. This is the case with a CPU that was discontinued by the manufacturer just over 5 years ago. I think this is why "padding" cases can make the patch look not much better and occasionally worse at the low end: Those keep the number of memtuples as a fraction of work_mem very low, and so mask the problems with the replacement selection heap. When Greg Stark benchmarked the patch at the low end, to identify regressions, he did find some slight regressions at the lowest work_mem settings with many many passes, but they were quite small [1]. Greg also did some good analysis of the performance characteristics of external sorting today [2] that I recommend reading if you missed. It's possible that those regressions have since been fixed, because Greg did not apply/test the memory batching patch that became commit 0011c0091e886b as part of this. It seems likely that it's at least partially fixed, and it might even be better than master overall, now. Anyway, what I liked about Greg's approach to finding regressions at the low end was that when testing, he used the cheapest possible VM available on Google's cloud platform. When testing the low end, he had low end hardware to go with the low end work_mem settings. This gave the patch the benefit of using quicksort to make good use of what I assume is a far smaller L2 cache; certainly nothing like 6MB or 12MB. I think Greg might have used a home server to test my patch in [1], actually, but I understand that it too was suitably low-end. It's perfectly valid to bring economics into this; typically, an external sort occurs only because memory isn't infinitely affordable, or it isn't worth provisioning enough memory to be totally confident that you can do every sort internally. With external sorting, the constant factors are what researchers generally spend most of the time worrying about. Knuth spends a lot of time discussing how the characteristics of actual magnetic tape drives changed throughout the 1970s in TAOCP Volume III. It's quite valid to ask if anyone would actually want to have an 8MB work_mem setting on a machine that has 12MB of L2 cache, cache that an external sort gets all to itself. Is that actually a practical setup that anyone would want to use? [1] http://www.postgresql.org/message-id/CAM-w4HOwt0C7ZndowHUuraw+xi+BhY5a6J008XoSq=R9z7H8rg@mail.gmail.com [2] http://www.postgresql.org/message-id/CAM-w4HM4XW3u5kVEuUrr+L+KX3WZ=5JKk0A=DJjzypkB-Hyu4w@mail.gmail.com -- Peter Geoghegan
On Wed, Mar 30, 2016 at 7:23 AM, Peter Geoghegan <pg@heroku.com> wrote: > Anyway, what I liked about Greg's approach to finding regressions at > the low end was that when testing, he used the cheapest possible VM > available on Google's cloud platform. When testing the low end, he had > low end hardware to go with the low end work_mem settings. This gave > the patch the benefit of using quicksort to make good use of what I > assume is a far smaller L2 cache; certainly nothing like 6MB or 12MB. > I think Greg might have used a home server to test my patch in [1], > actually, but I understand that it too was suitably low-end. I'm sorry I was intending to run those benchmarks again this past week but haven't gotten around to it. But my plan was to run them on a good server I borrowed, an i7 with 8MB cache. I can still go ahead with that but I can also try running it on the home server again too if you want (and AMD N36L with 1MB cache). But even for the smaller machines I don't think we should really be caring about regressions in the 4-8MB work_mem range. Earlier in the fuzzer work I was surprised to find out it can take tens of megabytes to compile a single regular expression (iirc it was about 30MB for a 64-bit machine) before you get errors. It seems surprising to me that a single operator would consume more memory than an ORDER BY clause. I was leaning towards suggesting we just bump up the default work_mem to 8MB or 16MB. -- greg
On Wed, Mar 30, 2016 at 4:22 AM, Greg Stark <stark@mit.edu> wrote: > I'm sorry I was intending to run those benchmarks again this past week > but haven't gotten around to it. But my plan was to run them on a good > server I borrowed, an i7 with 8MB cache. I can still go ahead with > that but I can also try running it on the home server again too if you > want (and AMD N36L with 1MB cache). I don't want to suggest that people not test the very low end on very high end hardware. That's fine, as long as it's put in context. Considerations about the economics of cache sizes and work_mem settings are crucial to testing the patch objectively. If everything fits in cache anyway, then you almost eliminate the advantages quicksort has, but you should be using an internal sort for anyway. I think that this is just common sense. I would like to see a low-end benchmark for low-end work_mem settings too, though. Maybe you could repeat the benchmark I linked to, but with a recent version of the patch, including commit 0011c0091e886b. Compare that to the master branch just before 0011c0091e886b went in. I'm curious about how the more recent memory context resetting stuff that made it into 0011c0091e886b left us regression-wise. Tomas tested that, of course, but I have some concerns about how representative his numbers are at the low end. > But even for the smaller machines I don't think we should really be > caring about regressions in the 4-8MB work_mem range. Earlier in the > fuzzer work I was surprised to find out it can take tens of megabytes > to compile a single regular expression (iirc it was about 30MB for a > 64-bit machine) before you get errors. It seems surprising to me that > a single operator would consume more memory than an ORDER BY clause. I > was leaning towards suggesting we just bump up the default work_mem to > 8MB or 16MB. Today, it costs less than USD $40 for a new Raspberry Pi 2, which has 1GB of memory. I couldn't figure out exactly how much CPU cache that model has, put I'm pretty sure it's no more than 256KB. Memory just isn't that expensive; memory bandwidth is expensive. I agree that we could easily justify increasing work_mem to 8MB, or even 16MB. It seems almost silly to point it out, but: Increasing sort performance has the effect of decreasing the duration of sorts, which could effectively decrease memory use on the system. Increasing the memory available to sorts could decrease the overall use of memory. Being really frugal with memory is expensive, maybe even if your primary concern is the expense of memory usage, which it probably isn't these days. -- Peter Geoghegan
On Thu, Feb 4, 2016 at 3:14 AM, Peter Geoghegan <pg@heroku.com> wrote: > Nyberg et al may have said it best in 1994, in the Alphasort Paper [1]: This paper is available from http://www.vldb.org/journal/VLDBJ4/P603.pdf (previously link is now dead) > The paper also has very good analysis of the economics of sorting: > > "Even for surprisingly large sorts, it is economical to perform the > sort in one pass." I suggest taking a look at "Figure 2. Replacement-selection sort vs. QuickSort" in the paper. It confirms what I said recently about cache size. The diagram is annotated: "The tournament tree of replacement-selection sort at left has bad cache behavior, unless the entire tournament fits in cache". I think we're well justified in giving no weight at all to cases where the *entire* tournament tree (heap) fits in cache, because it's not economical to use a cpu-cache-sized work_mem setting. It simply makes no sense. I understand the reluctance to give up on replacement selection. The authors of this paper were themselves reluctant to do so. As they put it: """ We were reluctant to abandon replacement-selection sort, because it has stability and it generates long runs. Our first approach was to improve replacement-selection sort's cache locality. Standard replacement-selection sort has terrible cache behavior, unless the tournament fits in cache. The cache thrashes on the bottom levels of the tournament. If you think of the tournament as a tree, each replacement-selection step traverses a path from a pseudo-random leaf of the tree to the root. The upper parts of the tree may be cache resident, but the bulk of the tree is not. We investigated a replacement-selection sort that clusters tournament nodes so that most parent-child node pairs are contained in the same cache line. This technique reduces cache misses by a factor of two or three. Nevertheless, replacement-selection sort is still less attractive than QuickSort because: 1. The cache behavior demonstrates less locality than QuickSorts. Even when QuickSort runs did not fit entirely in cache, the average compare-exchange time did not increase significantly. 2. Tournament sort is more CPU-intensive than QuickSort. Knuth calculated a 2:1 ratio for the programs he wrote. We observed a 2.5:1 speed advantage for QuickSort over the best tournament sort we wrote. The key to achieving high execution speeds on fast processors is to minimize the number of references that cannot be serviced by the on-board cache (4MB in the case of the DEC 7000 AXP). As mentioned before, QuickSort's memory access patterns are sequential and, thus, have good cache behavior """ This paper is co-authored by Jim Gray, a Turing award laureate, as well as some other very notable researchers. The paper appeared in "Readings in Database Systems, 4th edition", which was edited by by Joseph Hellerstein and Michael Stonebraker. These days, the cheapest consumer level CPUs have 4MB caches (in 1994, that was exceptional), so if this analysis wasn't totally justified in 1994, when the paper was written, it is today. I've spent a lot of time analyzing this problem. I've been looking at external sorting in detail for almost a year now. I've done my best to avoid any low-end regressions. I am very confident that I cannot do any better than I already have there, though. If various very influential figures in the database research community could not do better, then I have doubts that we can. I started with the intuition that we should still use replacement selection myself, but that just isn't well supported by benchmarking cases with sensible work_mem:cache size ratios. -- Peter Geoghegan
Hi, On 03/30/2016 04:53 AM, Peter Geoghegan wrote: > On Tue, Mar 29, 2016 at 6:02 PM, Tomas Vondra > <tomas.vondra@2ndquadrant.com> wrote: ... >>> If there is ever a regression, it is only really sensible to talk >>> about it while looking at trace_sort output (and, I guess, the query >>> plan). I've asked Tomas for trace_sort output in all relevant cases. >>> There is no point in "flying blind" and speculating what the problem >>> was from a distance. >> >> >> The updated benchmarks are currently running. I'm out of office until >> Friday, and I'd like to process the results over the weekend. FWIW I'll have >> results for these cases: >> >> 1) unpatched (a414d96a) >> 2) patched, default settings >> 3) patched, replacement_sort_mem=64 >> >> Also, I'll have trace_sort=on output for all the queries, so we can >> investigate further. > > Thanks! That will tell us a lot more. So, I do have the results from both machines - I've attached the basic comparison spreadsheets, the complete summary is available here: https://github.com/tvondra/sort-benchmark The database log also includes the logs for trace_sort=on for each query (use the timestamp logged for each query in the spreadsheet to locate the right section of the log). The benchmark was slightly modified, based on the previous feedback: * fix the maintenance_work_mem thinko (affects CREATE INDEX cases) * use "SELECT * FROM (... OFFSET 1e10)" pattern instead of the original approach (copy to /dev/null) * change the data generation for "low cardinality" data sets (by mistake it generated mostly the same stuff as "high cardinality") I have not collected explain plans. I guess we'll need explain analyze in most cases anyway, and collecting those would increase the duration of the benchmark. So I plan to collect this info for the interesting cases on request. While it might look like I'm somehow opposed to this patch series, that's mostly because we tend to look only at the few cases that behave poorly. So let me be clear: I do think the patch seems to be a significant performance improvement for most of the queries, and I'm OK with accepting a few regressions (particularly if we agree those are pathological cases, unlikely to happen in real-world workloads). It's quite rare that a patch is a universal win without regressions, so it's important to consider how likely those regressions are and what's the net effect of the patch - and the patch seems to be a significant improvement in most cases (and regressions limited to pathological or rare corner cases). I don't think those are reasons not to push this into 9.6. Following is a rudimentary analysis of the results, a bit about how the benchmark was constructed (and it's representativeness). rudimentary analysis -------------------- I haven't done any thorough investigation of the results yet, but in general it seems the results from both machines are quite similar - the numbers are different, but the speedup/slowdown patterns are mostly the same (with some exceptions that I'd guess are due to HW differences). The slowdown/speedup patterns (red/green cells in the spreadheets) are also similar to those collected originally. Some timings are much lower, presumably thanks to using the "OFFSET 1e10" pattern, but the patterns are the same. CREATE INDEX statements are an obvious exception, of course, due to the thinko in the previous benchmark. The one thing that surprised me a bit is that replacement_sort_mem=64 actually often made the results considerably worse in many cases. A common pattern is that the slowdown "spreads" to nearby cells - the are many queries where the 8MB case is 1:1 with master and 32MB is 1.5:1 (i.e. takes 1.5x more time), and setting replacement_sort_mem=64 just slows down the 8MB case. In general, replacement_sort_mem=64 seems to only affect the 8MB case, and in most cases it results in 100% slowdown (so 2x as long queries). That being said, I do think the results are quite impressive - there are far many queries with significant speedups (usually ~2x or more) than slowdowns (and less significant than speedups). I mostly agree with Peter that we probably don't need to worry about the slowdown cases with low work_mem settings - if you do sorts with millions of rows, you really need to give the database enough RAM. But there are multiple slowdown cases with work_mem=128MB, and I'd dare to say 128MB is not quite low-end work_mem value. So perhaps we should look at least at those cases. It's also interesting that setting replacement_sort_mem=64 makes this much worse - i.e. the number of slowdowns with higher work_mem values increases, and the difference is often quite huge. So I'm really not sure what to do with this GUC ... L2/L3 cache ----------- I think we're overly optimistic when it comes to the size of the CPU cache - while it's certainly true that modern CPUs have quite a bit of it (the modern Xeon E5 have up to ~45MB per socket), there are two important factors here: 1) The cache is shared by all cores on the socket (and on average there's ~2-3 MB/s per physical core), and thus by all processes running on the CPU. It's possible to run a single process on the CPU (thus getting all the cache), but that's a bit expensive 1-core CPU. 2) The cache is shared by all nodes in the query plan, and we do have executor that interleaves the nodes (so while an implementation of the node may be very efficient when executed in isolation, that may not be true when executed as part of a larger plan). The sort may be immune to this to some degree, though. I'm not sure how much this is considered in the 1994 VLDB paper, but I'd be very careful about making claims about how much CPU cache is available today (even on the best server CPUs). benchmark discussion -------------------- 1) representativeness Let me explain how I constructed the benchmark - I simply compiled a list of queries executing sorts, and ran them on synthetic datasets with different characteristics (cardinality and initial ordering). And I've done that with different work_mem values, to see how that affects the behavior. I've done it this way for a few reasons - firstly, I'm extremely lazy and did not want to study the internals of the patch as I'm not too much into sorting details. Secondly, I did not want to tailor the benchmark too tightly to the patch - it's quite possible some of the queries are not executing the modified code at all, in which case they should be unaffected (no slowdown, no speedup). So while the benchmark might certainly include additional queries or data sets with different characteristics, I'd dare to claim it's not entirely misguided. Some of the tested combinations may certainly be seen as implausible or pathological, although intentional and not constructed on purpose. I'm perfectly fine with identifying such cases and ignoring them. 2) TOAST overhead Peter also mentioned that some of the cases have quite a bit of padding, and that the TOAST overhead distorts the results. It's true there's quite a bit of padding (~320B), but I don't quite see why this would makes the results bogus - I've intentionally constructed it like this to see how the sort behaves with wide rows, because: * many BI queries actually fetch quite a lot of columns, and while 320B may seem a bit high, it's not that difficult to reach with a few NUMERIC columns * we're getting parallel aggregate in 9.6, which relies on serializing the aggregate state (and the combine phase may then need to do a sort again) Moreover, while there certainly is TOAST overhead, I don't quite see why it should change with the patch (as the padding columns are not used as a sort key). Perhaps the patch results in "moving the tuples around more" (deemphasizing comparison), but I don't see why that shouldn't be an important metric in general - memory bandwidth seems to be a quite important bottleneck these days. Of course, if this only affects the pathological cases, we may ignore that. regards -- Tomas Vondra http://www.2ndQuadrant.com PostgreSQL Development, 24x7 Support, Remote DBA, Training & Services
Attachment
On Sat, Apr 2, 2016 at 3:31 PM, Tomas Vondra <tomas.vondra@2ndquadrant.com> wrote: > So let me be clear: I do think the patch seems to be a significant > performance improvement for most of the queries, and I'm OK with accepting a > few regressions (particularly if we agree those are pathological cases, > unlikely to happen in real-world workloads). The ultra-short version of this is: 8MB: 0.98 32MB: 0.79 128MB: 0.63 512MB: 0.51 1GB: 0.42 These are the averages across all queries across all data sets for the run-time for the patch versus master (not patched 64 which I think is the replacement_sort_mem=64MB which appears to not be a win). So even in the less successful cases on average quicksort is faster than replacement selection. But selecting just the cases where 8MB is significantly slower than master it does look like the "padding" data sets are endemic. On the one hand that's a very realistic use-case where I think a lot of users find themselves. I know in my days as a web developer I typically threw a lot of columns into my queries and through a lot of joins and order by and then left it to the application to pick through the recordsets that were returned for the columns that were of interest. The tuples being sorted were probably huge. On the other hand perhaps this is something better tackled by the planner. If the planner can arrange sorts to happen when the rows are narrower that would be a a bigger win than trying to move a lot of data around like this. (In the extreme if it were possible to replace unnecessary columns by the tid and then refetching them later though that's obviously more than a little tricky to do effectively.) There are also some weird cases in this list where there's a significant regression at 32MB but not at 8MB. I would like to see 16MB and perhaps 12MB and 24MB. They would help understand if these are just quirks or there's a consistent pattern.
On Sat, Apr 2, 2016 at 3:20 PM, Greg Stark <stark@mit.edu> wrote: > There are also some weird cases in this list where there's a > significant regression at 32MB but not at 8MB. I would like to see > 16MB and perhaps 12MB and 24MB. They would help understand if these > are just quirks or there's a consistent pattern. I'll need to drill down to trace_sort output to see what happened there. -- Peter Geoghegan
On Sat, Apr 2, 2016 at 3:20 PM, Greg Stark <stark@mit.edu> wrote: > These are the averages across all queries across all data sets for the > run-time for the patch versus master (not patched 64 which I think is > the replacement_sort_mem=64MB which appears to not be a win). So even > in the less successful cases on average quicksort is faster than > replacement selection. It's actually replacement_sort_mem=64 (64KB -- effectively disabled). So where that case does better or worse, which can only be when work_mem=8MB in practice, that's respectively good or bad for replacement selection. So, typically RS does better when there are presorted inputs with a positive (not inverse/DESC) correlation, and there is little work_mem. As I've said, this is where the CPU cache is large enough to fit the entire memtuples heap. "Padded" cases are mostly bad because they make the memtuples heap relatively small in each case. So with work_mem=32MB, you get a memtuples heap structure similar to work_mem=8MB. The padding pushes things out a bit further, which favors master. -- Peter Geoghegan
On Sat, Apr 2, 2016 at 7:31 AM, Tomas Vondra <tomas.vondra@2ndquadrant.com> wrote: > So, I do have the results from both machines - I've attached the basic > comparison spreadsheets, the complete summary is available here: > > https://github.com/tvondra/sort-benchmark > > The database log also includes the logs for trace_sort=on for each query > (use the timestamp logged for each query in the spreadsheet to locate the > right section of the log). Thanks! Each row in these spreadsheets shows what looks like a multimodal distribution for the patch (if you focus on the actual run times, not the ratios). IOW, you can clearly see the regressions are only where master has its best case, and the patch its worst case; as the work_mem increases for each benchmark case for the patch, by far the largest improvement is usually seen as we cross the CPU cache threshold. Master gets noticeably slower as work_mem goes from 8MB to 32MB, but the patch gets far far faster. Things continue to improve for patched in absolute terms and especially relative to master following further increases in work_mem, but not nearly as dramatically as that first increment (unless we have lots of padding, which makes the memtuples heap itself much smaller, so it happens one step later). Master shows a slow decline at and past 32MB of work_mem. If the test hardware had a larger L3 cache, we might expect to notice a second big drop, but this hardware doesn't have the enormous L3 cache sizes of new Xeon processors (e.g. 32MB, 45MB). > While it might look like I'm somehow opposed to this patch series, that's > mostly because we tend to look only at the few cases that behave poorly. > > So let me be clear: I do think the patch seems to be a significant > performance improvement for most of the queries, and I'm OK with accepting a > few regressions (particularly if we agree those are pathological cases, > unlikely to happen in real-world workloads). > > It's quite rare that a patch is a universal win without regressions, so it's > important to consider how likely those regressions are and what's the net > effect of the patch - and the patch seems to be a significant improvement in > most cases (and regressions limited to pathological or rare corner cases). > > I don't think those are reasons not to push this into 9.6. I didn't think that you opposed the patch. In fact, you did the right thing by focussing on the low-end regressions, as I've said. I was probably too concerned about Robert failing to consider that they were not representative, particularly with regard to how small the memtuples heap could be relative to the CPU cache; blame it on how close I've become to this problem. I'm pretty confident that Robert can be convinced that these do not matter enough to not commit the patch. In any case, I'm pretty confident that I cannot fix any remaining regressions. > I haven't done any thorough investigation of the results yet, but in general > it seems the results from both machines are quite similar - the numbers are > different, but the speedup/slowdown patterns are mostly the same (with some > exceptions that I'd guess are due to HW differences). I agree. What we clearly see is the advantages of quicksort being cache oblivious, especially relative to master's use of a heap. That advantage becomes pronounced at slightly different points in each case, but the overall picture is the same. This pattern demonstrates why a cache oblivious algorithm is so useful in general -- we don't have to care about tuning for that. As important as this is for serial sorts, it's even more important for parallel sorts, where parallel workers compete for memory bandwidth, and where it's practically impossible to build a cost model for CPU cache size + memory use + nworkers. > The slowdown/speedup patterns (red/green cells in the spreadheets) are also > similar to those collected originally. Some timings are much lower, > presumably thanks to using the "OFFSET 1e10" pattern, but the patterns are > the same. I think it's notable that this made things more predictable, and made the benefits clearer. > The one thing that surprised me a bit is that > > replacement_sort_mem=64 > > actually often made the results considerably worse in many cases. A common > pattern is that the slowdown "spreads" to nearby cells - the are many > queries where the 8MB case is 1:1 with master and 32MB is 1.5:1 (i.e. takes > 1.5x more time), and setting replacement_sort_mem=64 just slows down the 8MB > case. > > In general, replacement_sort_mem=64 seems to only affect the 8MB case, and > in most cases it results in 100% slowdown (so 2x as long queries). To be clear, for the benefit of other people: replacement_sort_mem=64 makes the patch never use a replacement selection heap, even at the lowest tested work_mem setting of 8MB. This is exactly what I expected. When replacement_sort_mem is the proposed default of 16MB, it literally has zero impact on how the patch behaves where work_mem > replacement_sort_mem. So, since the only case where work_mem <= replacement_sort_mem is when work_mem = 8MB, that's the only case where any change can be seen in either direction. I thought it was important to see that (but more so when we have cheap hardware with little CPU cache). > That being said, I do think the results are quite impressive - there are far > many queries with significant speedups (usually ~2x or more) than slowdowns > (and less significant than speedups). > > I mostly agree with Peter that we probably don't need to worry about the > slowdown cases with low work_mem settings - if you do sorts with millions of > rows, you really need to give the database enough RAM. Cool. > But there are multiple slowdown cases with work_mem=128MB, and I'd dare to > say 128MB is not quite low-end work_mem value. So perhaps we should look at > least at those cases. > > It's also interesting that setting replacement_sort_mem=64 makes this much > worse - i.e. the number of slowdowns with higher work_mem values increases, > and the difference is often quite huge. > > So I'm really not sure what to do with this GUC ... I think it mostly depends on how systems that might actually need replacement_sort_mem do with and without it. I mean, cases that need it because work_mem=8MB is generally reasonable, because low-end hardware is in use. That's why I asked Greg to use cheap hardware at least once. It matters more if work_mem=8MB is regressed when you have a CPU cache size of 1MB (and there is no competition for the cache). > L2/L3 cache > ----------- > > I think we're overly optimistic when it comes to the size of the CPU cache - > while it's certainly true that modern CPUs have quite a bit of it (the > modern Xeon E5 have up to ~45MB per socket), there are two important factors > here: > I'm not sure how much this is considered in the 1994 VLDB paper, but I'd be > very careful about making claims about how much CPU cache is available today > (even on the best server CPUs). I agree. That's why it's so important that we use CPU cache effectively. > benchmark discussion > -------------------- > > 1) representativeness > I've done it this way for a few reasons - firstly, I'm extremely lazy and > did not want to study the internals of the patch as I'm not too much into > sorting details. Secondly, I did not want to tailor the benchmark too > tightly to the patch - it's quite possible some of the queries are not > executing the modified code at all, in which case they should be unaffected > (no slowdown, no speedup). That's right -- a couple of cases do not exercise the patch because the sort is an internal sort. I think that this isn't too hard to figure out now, though. I get why you did things this way. I appreciate your help. > Some of the tested combinations may certainly be seen as implausible or > pathological, although intentional and not constructed on purpose. I'm > perfectly fine with identifying such cases and ignoring them. Me too. Or, if not ignoring them, only giving a very small weight to them. > 2) TOAST overhead > Moreover, while there certainly is TOAST overhead, I don't quite see why it > should change with the patch (as the padding columns are not used as a sort > key). Perhaps the patch results in "moving the tuples around more" > (deemphasizing comparison), but I don't see why that shouldn't be an > important metric in general - memory bandwidth seems to be a quite important > bottleneck these days. Of course, if this only affects the pathological > cases, we may ignore that. That's fair. I probably shouldn't have mentioned TOAST at all -- what's actually important to keep in mind about padding cases, as already mentioned, is that they can make the 32MB cases behave like the 8MB cases. The memtuples heap is left relatively small for the 32MB case too, and so can remain cache resident. Replacement selection therefore almost accidentally gets fewer heap cache misses for a little longer, but it's still the same pattern. Cache misses come to dominate a bit later. -- Peter Geoghegan
On Sat, Apr 2, 2016 at 3:22 PM, Peter Geoghegan <pg@heroku.com> wrote: > On Sat, Apr 2, 2016 at 3:20 PM, Greg Stark <stark@mit.edu> wrote: >> There are also some weird cases in this list where there's a >> significant regression at 32MB but not at 8MB. I would like to see >> 16MB and perhaps 12MB and 24MB. They would help understand if these >> are just quirks or there's a consistent pattern. > > I'll need to drill down to trace_sort output to see what happened there. I looked into this. I too noticed that queries like "SELECT a FROM int_test UNION SELECT a FROM int_test_padding" looked strangely faster for 128MB + high_cardinality_almost_asc + i5 for master branch. This made the patch look relatively bad for the test with those exact properties only; the patch was faster with both lower and higher work_mem settings than 128MB. There was a weird spike in performance for the master branch only. Having drilled down to trace_sort output, I think I know roughly why. I see output like this: 1459308434.753 2016-03-30 05:27:14 CEST STATEMENT: SELECT * FROM (SELECT a FROM int_test UNION SELECT a FROM int_test_padding OFFSET 1e10) ff; I think that this is invalid, because the query was intended as this: SELECT * FROM (SELECT * FROM (SELECT a FROM int_test UNION SELECT a FROM int_test_padding) gg OFFSET 1e10) ff; This would have controlled for client overhead, per my request to Tomas, without altering the "underlying query" that you see in the final spreadsheet. I don't have an exact explanation for why you'd see this spike at 128MB for the master branch but not the other at the moment, but it seems like that one test is basically invalid, and should be discarded. I suspect that the patch didn't see its own similar spike due to my changes to cost_sort(), which reflected that sorts don't need to do so much expensive random I/O. This is the only case that I saw that was not more or less consistent with my expectations, which is good. -- Peter Geoghegan
Hi, So, let me sum this up, the way I understand the current status. 1) overall, the patch seems to be a clear performance improvement There's far more "green" cells than "red" ones in the spreadsheets, and the patch often shaves off 30-75% of the sort duration. Improvements are pretty much all over the board, for all data sets (low/high/unique cardinality, initial ordering) and data types. 2) it's unlikely we can improve the performance further The regressions are limited to low work_mem settings, which we believe are not representative (or at least not as much as the higher work_mem values), for two main reasons. Firstly, if you need to sort a lot of data (e.g. 10M, as benchmarked), it's quite reasonable to use larger work_mem values. It'd be a bit backwards to reject a patch that gets you 2-4x speedup with enough memory, on the grounds that it may have negative impact with unreasonably small work_mem values. Secondly, master is faster only if there's enough on-CPU cache for the replacement sort (for the memtuples heap), but the benchmark is not realistic in this respect as it only ran 1 query at a time, so it used the whole cache (6MB for i5, 12MB for Xeon). In reality there will be multiple processes running at the same time (e.g backends when running parallel query), significantly reducing the amount of cache per process, making the replacement sort inefficient and thus eliminating the regressions (by making the master slower). 3) replacement_sort_mem GUC I'm not quite sure what's the plan with this GUC. It was useful for development, but it seems to me it's pretty difficult to tune it in practice (especially if you don't know the internals, which users generally don't). The current patch includes the new GUC right next to work_mem, which seems rather unfortunate - I do expect users to simply mess with assuming "more is better" which seems to be rather poor idea. So I think we should either remove the GUC entirely, or move it to the developer section next to trace_sort (and removing it from the conf). I'm wondering whether 16MB default is not a bit too much, actually. As explained before, that's not the amount of cache we should expect per process, so maybe ~2-4MB would be a better default value? Also, not what I'm re-reading the docs for the GUC, I realize it also depends on how the input data is correlated - that seems like a rather useless criteria for tuning, though, because that varies per sort node, so using that for a GUC value set in postgresql.conf does not seem very wise. Actually even on per-query basis that's rather dubious, as it depends on how the sort node gets data (some nodes preserve ordering, some don't). BTW couldn't we tune the value automatically for each sort, using the pg_stats.correlation for the sort keys, when available (increasing the replacement_sort_mem when correlation is close to 1.0)? Wouldn't that improve at least some of the regressions? regards -- Tomas Vondra http://www.2ndQuadrant.com PostgreSQL Development, 24x7 Support, Remote DBA, Training & Services
Hi Tomas, Overall, I agree with your summary. On Sun, Apr 3, 2016 at 5:24 AM, Tomas Vondra <tomas.vondra@2ndquadrant.com> wrote: > So, let me sum this up, the way I understand the current status. > > > 1) overall, the patch seems to be a clear performance improvement I think that's clear. There are even cases that are over 5x faster, which are representative of some real workloads (e.g., "CREATE INDEX x ON numeric_test (a)" when low_cardinality_almost_asc + maintenance_work_mem=512MB). A lot of the aggregate (datum sort) cases, and heap tuple cases are 3x - 4x faster. > 2) it's unlikely we can improve the performance further I think it's very unlikely that these remaining regressions can be fixed, yes. > Secondly, master is faster only if there's enough on-CPU cache for the > replacement sort (for the memtuples heap), but the benchmark is not > realistic in this respect as it only ran 1 query at a time, so it used the > whole cache (6MB for i5, 12MB for Xeon). > > In reality there will be multiple processes running at the same time (e.g > backends when running parallel query), significantly reducing the amount of > cache per process, making the replacement sort inefficient and thus > eliminating the regressions (by making the master slower). Agreed. And even though the 8MB work_mem cases always have more than enough CPU cache to fit the replacement selection heap, it's still no worse than a mixed picture. The replacement_work_mem=64KB + patch + 8MB (maintenance_)work_mem cases (i.e. replacement selection entirely disabled) don't always do worse; they are often a draw, and sometimes do much better. We *still* win in many cases, sometimes by quite a bit (e.g. "SELECT COUNT(DISTINCT a) FROM int_test" typically loses about 50% of its runtime when patched and RS is disabled at work_mem=8MB). The cases where we lose at work_mem=8MB involve padding and a correlation. The really important case of CREATE INDEX on int4 almost always wins, *even with sorted input* (the almost-but-not-quite-asc-sorted case loses ~1%). We can shave 20% - 30% off the CREATE INDEX int4 cases with just maintenance_work_mem = 8MB. Even in these cases with so much CPU cache relative to work_mem, you need to search for regressed cases to find them, and they are less representative cases. So, while the picture for the work_mem=8MB column alone seems kind of bad, if you consider where the regressions actually occur, you could argue that even that's a draw. > 3) replacement_sort_mem GUC > > I'm not quite sure what's the plan with this GUC. It was useful for > development, but it seems to me it's pretty difficult to tune it in practice > (especially if you don't know the internals, which users generally don't). I agree. > So I think we should either remove the GUC entirely, or move it to the > developer section next to trace_sort (and removing it from the conf). I'll let Robert decide what's best here, but I see your point. Side note: trace_sort actually is documented. It's a bit weird that we have those TRACE_SORT macros at all IMV. I think we should rip those out, and assume every build enables TRACE_SORT, because that's probably true anyway. I do think that replacement selection could be put to good use for CREATE INDEX if the CREATE INDEX utility command had a "presorted" parameter. Specifically, an implementation of the "presorted" idea that I recently sketched [1] could do better than any presorted replacement selection case we've seen so far because it allows the implementation to optimistically create the index on-the-fly (if that isn't possible, throw an error), without a second pass over tuples sorted on tape. Nothing needs to be stored on a tape/temp file *at all*; the only thing that is stored externally is the index itself. But this patch doesn't add that feature, which can be worked on without the user needing to know about replacement_sort_mem in 9.6. So, I'm not in favor of ripping out the replacement selection code, but think it could make sense to effectively disable it entirely for the time being (with some developer feature to turn it back on for testing). In general, I share your misgivings about the new GUC, though. > I'm wondering whether 16MB default is not a bit too much, actually. As > explained before, that's not the amount of cache we should expect per > process, so maybe ~2-4MB would be a better default value? The obvious presorted case is where we have a SERIAL column, but as I mentioned even that isn't helped by RS. Moreover, it will be significantly hurt with a default maintenance_work_mem of 64MB. Your int4 CREATE INDEX cases clearly show this. > BTW couldn't we tune the value automatically for each sort, using the > pg_stats.correlation for the sort keys, when available (increasing the > replacement_sort_mem when correlation is close to 1.0)? Wouldn't that > improve at least some of the regressions? Maybe, but that seems hard. That information isn't conveniently available to the executor/tuplesort, and as we've seen with CREATE INDEX int4 cases, it's far from clear that we'll win even when there definitely is presorted input. Replacement selection needs more than a simple correlation to win, so you'll end up building a cost model with many new problems if this is to work. [1] http://www.postgresql.org/message-id/CAM3SWZRFzg1LUK8FBg_goZ8zL0n7k6q83qQjhOV8NDZioA5TEQ@mail.gmail.com -- Peter Geoghegan
On 04/03/2016 09:41 PM, Peter Geoghegan wrote: > Hi Tomas, ... >> 3) replacement_sort_mem GUC >> >> I'm not quite sure what's the plan with this GUC. It was useful for >> development, but it seems to me it's pretty difficult to tune it in practice >> (especially if you don't know the internals, which users generally don't). > > I agree. > >> So I think we should either remove the GUC entirely, or move it to the >> developer section next to trace_sort (and removing it from the conf). > > I'll let Robert decide what's best here, but I see your point. > > Side note: trace_sort actually is documented. It's a bit weird that we > have those TRACE_SORT macros at all IMV. I think we should rip those > out, and assume every build enables TRACE_SORT, because that's > probably true anyway. What do you mean by documented? I thought this might be a good place is: http://www.postgresql.org/docs/devel/static/runtime-config-developer.html which is where trace_sort is documented. > > I do think that replacement selection could be put to good use for > CREATE INDEX if the CREATE INDEX utility command had a "presorted" > parameter. Specifically, an implementation of the "presorted" idea > that I recently sketched [1] could do better than any presorted > replacement selection case we've seen so far because it allows the > implementation to optimistically create the index on-the-fly (if that > isn't possible, throw an error), without a second pass over tuples > sorted on tape. Nothing needs to be stored on a tape/temp file *at > all*; the only thing that is stored externally is the index itself. > But this patch doesn't add that feature, which can be worked on > without the user needing to know about replacement_sort_mem in 9.6. > > So, I'm not in favor of ripping out the replacement selection code, > but think it could make sense to effectively disable it entirely for > the time being (with some developer feature to turn it back on for > testing). In general, I share your misgivings about the new GUC, > though. OK. > >> I'm wondering whether 16MB default is not a bit too much, actually. As >> explained before, that's not the amount of cache we should expect per >> process, so maybe ~2-4MB would be a better default value? > > The obvious presorted case is where we have a SERIAL column, but as I > mentioned even that isn't helped by RS. Moreover, it will be > significantly hurt with a default maintenance_work_mem of 64MB. Your > int4 CREATE INDEX cases clearly show this. > >> BTW couldn't we tune the value automatically for each sort, using the >> pg_stats.correlation for the sort keys, when available (increasing the >> replacement_sort_mem when correlation is close to 1.0)? Wouldn't that >> improve at least some of the regressions? > > Maybe, but that seems hard. That information isn't conveniently > available to the executor/tuplesort, and as we've seen with CREATE > INDEX int4 cases, it's far from clear that we'll win even when there > definitely is presorted input. Replacement selection needs more than a > simple correlation to win, so you'll end up building a cost model with > many new problems if this is to work. Sure, that's non-trivial and definitely not a 9.6 material. I'm also wondering whether we need to do choose replacement_sort_mem at planning time, or whether it could be done in the executor based on actually observed data ... regards -- Tomas Vondra http://www.2ndQuadrant.com PostgreSQL Development, 24x7 Support, Remote DBA, Training & Services
<p dir="ltr">I just mean that, as you say, trace_sort is described in the documentation. <p dir="ltr">I don't think we'llend up with any kind of cost model here, so where that would need to happen is only an academic matter. The create indexparameter would only be an option for the DBA. That's about the only case I can see working for replacement selection:where indexes can be created with very little memory quickly, by optimistically starting to write out the startof the final index representation almost immediately, before most of the underlying table has even been read in. <pdir="ltr">--<br /> Peter Geoghegan
On Sun, Apr 3, 2016 at 12:50 AM, Peter Geoghegan <pg@heroku.com> wrote: > 1459308434.753 2016-03-30 05:27:14 CEST STATEMENT: SELECT * FROM > (SELECT a FROM int_test UNION SELECT a FROM int_test_padding OFFSET > 1e10) ff; > > I think that this is invalid, because the query was intended as this: > > SELECT * FROM (SELECT * FROM (SELECT a FROM int_test UNION SELECT a > FROM int_test_padding) gg OFFSET 1e10) ff; ISTM OFFSET binds more loosely than UNION so these should be equivalent. -- greg
On Sun, Apr 3, 2016 at 4:08 PM, Greg Stark <stark@mit.edu> wrote: >> SELECT * FROM (SELECT * FROM (SELECT a FROM int_test UNION SELECT a >> FROM int_test_padding) gg OFFSET 1e10) ff; > > ISTM OFFSET binds more loosely than UNION so these should be equivalent. Not exactly: postgres=# explain analyze select i from fff union select i from ggg offset 1e10; QUERY PLAN ---------------------------------------------------------------------------------------------------------------------------------------Limit (cost=357771.51..357771.51 rows=1 width=4) (actual time=2989.378..2989.378 rows=0 loops=1) -> Unique (cost=345771.50..357771.51 rows=2400002 width=4) (actual time=2031.044..2930.903 rows=1500001 loops=1) -> Sort (cost=345771.50..351771.51 rows=2400002 width=4) (actual time=2031.042..2543.167 rows=2400002 loops=1) Sort Key: fff.i Sort Method: external merge Disk: 32840kB -> Append (cost=0.00..58620.04 rows=2400002 width=4) (actual time=0.048..435.408 rows=2400002 loops=1) -> Seq Scan on fff (cost=0.00..14425.01 rows=1000001 width=4) (actual time=0.048..100.435 rows=1000001 loops=1) -> Seq Scan on ggg (cost=0.00..20195.01 rows=1400001 width=4) (actual time=0.042..138.991 rows=1400001 loops=1)Planning time: 0.123 msExecution time: 2999.564 ms (10 rows) postgres=# explain analyze select * from (select i from fff union select i from ggg) fg offset 1e10; QUERY PLAN ---------------------------------------------------------------------------------------------------------------------------------------Limit (cost=381771.53..381771.53 rows=1 width=4) (actual time=2982.519..2982.519 rows=0 loops=1) -> Unique (cost=345771.50..357771.51 rows=2400002 width=4) (actual time=2009.176..2922.874 rows=1500001 loops=1) -> Sort (cost=345771.50..351771.51 rows=2400002 width=4) (actual time=2009.174..2522.761 rows=2400002 loops=1) Sort Key: fff.i Sort Method: external merge Disk: 32840kB -> Append (cost=0.00..58620.04 rows=2400002 width=4) (actual time=0.056..428.934 rows=2400002 loops=1) -> Seq Scan on fff (cost=0.00..14425.01 rows=1000001 width=4) (actual time=0.055..100.806 rows=1000001 loops=1) -> Seq Scan on ggg (cost=0.00..20195.01 rows=1400001 width=4) (actual time=0.042..139.994 rows=1400001 loops=1)Planning time: 0.127 msExecution time: 2993.294 ms (10 rows) The startup and total costs are greater in the latter case, but the costs match at and below the Unique node. Whether or not this was relevant is probably unimportant, though. My habit is to do the offset outside of the subquery. My theory is that the master branch happened to get a HashAggregate for the 128MB case that caused us both confusion, because it looked cheaper than an external sort + unique when the sort required many passes on the master branch only (where my cost_sort() changes that lower the costing of external sorts were not included). This wasn't a low cardinality case, so the HashAggregate may have only won by a small amount. I suppose that this could happen when the HashAggregate was not predicted to use memory > work_mem, but a sort was. Then, as the sort requires fewer merge passes with more work_mem, the master branch starts to agree with the patch on the cheapest plan once again. The trend of the patch being faster continues, after this one hiccup. This is down to the cost_sort() changes, not the tuplesort.c changes. But this was just a quirk, and the trend still seems clear. This theory seems very likely based on this strange query's numbers for i5 master as work_mem increases: Master: 16.711, 9.94, 4.891, 8.32, 4.88 Patch: 17.23, 9.77, 9.78, 4.95, 4.94 ISTM that master's last and third-from-last cases *both* use a HashAggregate, where the patch behaves more consistently. After all, the patch does smooth the cost function of sorting, an independently useful goal to simply making sorting faster. We don't have to be afraid of crossing an arbitrary, fuzzy threshold. -- Peter Geoghegan
Sorry for not responding to this thread again sooner. I was on vacation Thursday-Sunday, and have been playing catch-up since then. On Sun, Apr 3, 2016 at 8:24 AM, Tomas Vondra <tomas.vondra@2ndquadrant.com> wrote: > Secondly, master is faster only if there's enough on-CPU cache for the > replacement sort (for the memtuples heap), but the benchmark is not > realistic in this respect as it only ran 1 query at a time, so it used the > whole cache (6MB for i5, 12MB for Xeon). > > In reality there will be multiple processes running at the same time (e.g > backends when running parallel query), significantly reducing the amount of > cache per process, making the replacement sort inefficient and thus > eliminating the regressions (by making the master slower). Interesting point. > 3) replacement_sort_mem GUC > > I'm not quite sure what's the plan with this GUC. It was useful for > development, but it seems to me it's pretty difficult to tune it in practice > (especially if you don't know the internals, which users generally don't). > > The current patch includes the new GUC right next to work_mem, which seems > rather unfortunate - I do expect users to simply mess with assuming "more is > better" which seems to be rather poor idea. > > So I think we should either remove the GUC entirely, or move it to the > developer section next to trace_sort (and removing it from the conf). I certainly agree that GUCs that aren't easy to tune are bad. I'm wondering whether the fact that this one is hard to tune is something that can be fixed. The comments about "padding" - a term I don't like, because it to me implies a deliberate attempt to game the benchmark when in reality wanting to sort a wide row is entirely reasonable - make me wonder if this should be based on a number of tuples rather than an amount of memory. If considering the row width makes us get the wrong answer, then let's not do that. > BTW couldn't we tune the value automatically for each sort, using the > pg_stats.correlation for the sort keys, when available (increasing the > replacement_sort_mem when correlation is close to 1.0)? Wouldn't that > improve at least some of the regressions? Surely not for 9.6. -- Robert Haas EnterpriseDB: http://www.enterprisedb.com The Enterprise PostgreSQL Company
On Thu, Apr 7, 2016 at 6:55 AM, Robert Haas <robertmhaas@gmail.com> wrote: >> In reality there will be multiple processes running at the same time (e.g >> backends when running parallel query), significantly reducing the amount of >> cache per process, making the replacement sort inefficient and thus >> eliminating the regressions (by making the master slower). > > Interesting point. The effective use of CPU cache is *absolutely* critical here. I think that this patch is valuable primarily because it makes sorting predictable, and only secondarily because it makes it much faster. Having discrete costs that can be modeled fairly accurately has significant practical benefits for DBAs, and for query optimization, especially when parallel worker sorts must be costed. Inefficient use of CPU cache implies a big overall cost for the server, not just one client; my sorting patches are usually tested on single client cases, but the multi-client cases can be a lot more sympathetic (we saw this with abbreviated keys at one point). I wonder how many DBAs are put off by higher work_mem settings due to issues with replacement selection....they are effectively denied the ability to set work_mem appropriately across the board, because of this one weak spot. It really is perverse that there is, in effect, a "Blackjack" cost function for sorts, which runs counter to the general intuition that more memory is better. > I certainly agree that GUCs that aren't easy to tune are bad. I'm > wondering whether the fact that this one is hard to tune is something > that can be fixed. The comments about "padding" - a term I don't > like, because it to me implies a deliberate attempt to game the > benchmark when in reality wanting to sort a wide row is entirely > reasonable - make me wonder if this should be based on a number of > tuples rather than an amount of memory. If considering the row width > makes us get the wrong answer, then let's not do that. That's a good point. While I don't think it will make it easy to tune the GUC, it will make it easier. Although, I think that it should probably still be GUC_UNIT_KB. That should just be something that my useselection() function compares to the overall size of memtuples alone when we must initially spill, not the value of work_mem/maintenance_work_mem. The degree of padding isn't entirely irrelevant, because not all comparisons will be resolved at the stup.datum1 level, but it's still clearly an improvement to not have wide tuples mess with things. Would you like me to revise the patch along those lines? Or, do you prefer units of tuples? Tuples are basically equivalent, but make it way less obvious what the relationship with CPU cache might be. If I revise the patch along these lines, I should also reduce the default replacement_sort_mem to produce roughly equivalent behavior for non-padded cases. -- Peter Geoghegan
On Mon, Mar 21, 2016 at 2:01 AM, Peter Geoghegan <pg@heroku.com> wrote: > On Thu, Mar 17, 2016 at 1:13 PM, Robert Haas <robertmhaas@gmail.com> wrote: >> OK, I have now committed 0001 > > I attach a revision of the external quicksort patch and supplementary > small patches, rebased on top of the master branch. I spent some time today reading through the new 0001 and in general I think it looks pretty good. But I think that there is some stuff in there that logically seems to me to deserve to be separate patches. In particular: 1. Changing cost_sort to consider disk access as 90% sequential, 10% random rather than 75% sequential, 25% random. As far as I can recall from the thread, zero test results have been posted to demonstrate that this is a good idea. It also seems completely unprincipled. If the cost of sorts decreases as a result of this patch, it is because we've reduced the CPU cost, not the I/O cost. The changes we're talking about here make I/O more random, not less random, because we will now have more tapes, not fewer; which means merges will have to seek the disk head more frequently, not less frequently. Now, it's tempting to say that this patch should result in some change to the cost model: if the patch doesn't make sorting faster, we shouldn't commit it at all, and if it does, then surely the cost model should change accordingly. But the question for the cost model isn't whether the change to the model somehow reflects the increase in execution speed. It's whether we get better query plans with the change than without. I don't think there's been a degree of review of that aspect of this patch on list that would give me confidence to commit a change like this. 2. Restricting the maximum number of tapes to 500. This seems like a sound change and I don't object to it in theory. But I've seen no benchmark results which demonstrate that this is a good idea, and it is quite separate from the core purpose of the patch. Since time is short, I recommend we remove both of these things from the patch and you can resubmit them as separate patches later. As far as I can see, neither of them is so tied into the rest of the patch that the main part of the patch can't be committed without those changes. -- Robert Haas EnterpriseDB: http://www.enterprisedb.com The Enterprise PostgreSQL Company
On Thu, Apr 7, 2016 at 1:17 PM, Peter Geoghegan <pg@heroku.com> wrote: >> I certainly agree that GUCs that aren't easy to tune are bad. I'm >> wondering whether the fact that this one is hard to tune is something >> that can be fixed. The comments about "padding" - a term I don't >> like, because it to me implies a deliberate attempt to game the >> benchmark when in reality wanting to sort a wide row is entirely >> reasonable - make me wonder if this should be based on a number of >> tuples rather than an amount of memory. If considering the row width >> makes us get the wrong answer, then let's not do that. > > That's a good point. While I don't think it will make it easy to tune > the GUC, it will make it easier. Although, I think that it should > probably still be GUC_UNIT_KB. That should just be something that my > useselection() function compares to the overall size of memtuples > alone when we must initially spill, not the value of > work_mem/maintenance_work_mem. The degree of padding isn't entirely > irrelevant, because not all comparisons will be resolved at the > stup.datum1 level, but it's still clearly an improvement to not have > wide tuples mess with things. > > Would you like me to revise the patch along those lines? Or, do you > prefer units of tuples? Tuples are basically equivalent, but make it > way less obvious what the relationship with CPU cache might be. If I > revise the patch along these lines, I should also reduce the default > replacement_sort_mem to produce roughly equivalent behavior for > non-padded cases. I prefer units of tuples, with the GUC itself therefore being unitless. I suggest we call the parameter replacement_sort_threshold and document that (1) the ideal value may depend on the amount of CPU cache available to running processes, with more cache implying higher values; and (2) the ideal value may depend somewhat on the input data, with more correlation implying higher values. And then pick some value that you think is likely to work well for most people and call it good. If you could prepare a new patch with those changes and also making the changes requested in my other email, I will try to commit that before the deadline. Thanks. -- Robert Haas EnterpriseDB: http://www.enterprisedb.com The Enterprise PostgreSQL Company
On Thu, Apr 7, 2016 at 11:05 AM, Robert Haas <robertmhaas@gmail.com> wrote: > I spent some time today reading through the new 0001 and in general I > think it looks pretty good. Cool. > 1. Changing cost_sort to consider disk access as 90% sequential, 10% > random rather than 75% sequential, 25% random. As far as I can recall > from the thread, zero test results have been posted to demonstrate > that this is a good idea. It also seems completely unprincipled. I think that it's less unprincipled than the existing behavior, which imagines that I/O is a significant cost overall, something that is demonstrably wrong (there is an XXX comment about the existing disk access costings). Still, I agree that there is no logical reason to connect it to the bulk of what I want to do here, except that maybe it would be good if we were more optimistic about the cost of external sorting now. cost_sort() knows nothing about cache efficiency, of course, so naturally we cannot teach it to weigh cache efficiency less heavily. I guess I was worried that the smaller run sizes would put cost_sort() off external sorts even more, even as they became far cheaper. > 2. Restricting the maximum number of tapes to 500. This seems like a > sound change and I don't object to it in theory. But I've seen no > benchmark results which demonstrate that this is a good idea, and it > is quite separate from the core purpose of the patch. Ditto. This is something that could be done separately. We've often pondered if it made any sense at all (e.g. commit message of c65ab0bfa97b71bceae6402498910f4074996279), and I'm sure that it doesn't, but the memory refund stuff in the already memory management patch at least refunds the cost for the final on-the-fly merge (iff state->tuples). > Since time is short, I recommend we remove both of these things from > the patch and you can resubmit them as separate patches later. As far > as I can see, neither of them is so tied into the rest of the patch > that the main part of the patch can't be committed without those > changes. I agree to all this. Now that you've indicated where you stand on replacement_sort_mem, I have all the information I need to produce a new revision. I'll go do that. Thanks -- Peter Geoghegan
On Thu, Apr 7, 2016 at 11:10 AM, Robert Haas <robertmhaas@gmail.com> wrote: > I prefer units of tuples, with the GUC itself therefore being > unitless. I suggest we call the parameter replacement_sort_threshold > and document that (1) the ideal value may depend on the amount of CPU > cache available to running processes, with more cache implying higher > values; and (2) the ideal value may depend somewhat on the input data, > with more correlation implying higher values. And then pick some > value that you think is likely to work well for most people and call > it good. I really don't want to bikeshed about this, but I must ask: if the name of the GUC must include the word "threshold", shouldn't it be called quicksort_threshold? My dictionary defines threshold as "any place or point of entering or beginning". But this GUC does not govern where replacement selection begins; it governs where it ends. How do you feel about replacement_sort_tuples? We already use the word "tuple" in the names of GUCs. -- Peter Geoghegan
On Thu, Apr 7, 2016 at 11:10 AM, Robert Haas <robertmhaas@gmail.com> wrote: > I prefer units of tuples, with the GUC itself therefore being > unitless. I suggest we call the parameter replacement_sort_threshold > and document that (1) the ideal value may depend on the amount of CPU > cache available to running processes, with more cache implying higher > values; and (2) the ideal value may depend somewhat on the input data, > with more correlation implying higher values. And then pick some > value that you think is likely to work well for most people and call > it good. > > If you could prepare a new patch with those changes and also making > the changes requested in my other email, I will try to commit that > before the deadline. Thanks. Attached revision of patch series: * Breaks out the parts you don't want to commit right now, as agreed. These separate patches in the rebased patch series are included here for completeness, but will probably be submitted separately to 9.7. I do still think you should commit 0002-* alongside 0001-*, though, because it's useful to be able to enable the memory context dumps on dev builds to debug external sorting. I won't insist on it, but that is my recommendation. * Fixes "over-zealous assertion" that I pointed out recently. * Replaces replacement_sort_mem GUC with replacement_sort_tuples GUC, since, as discussed, effective cut-off points for using replacement selection for the first run are easier to derive from the size of memtuples (the might-be heap) than from work_mem/maintenance_work_mem (the fraction of all tuplesort memory used that is used for memtuples could be very low in cases with what Tomas called "padding"). Since you didn't get back to me on the name of the GUC, I just ran with the name replacement_sort_tuples, but that's something I'm totally unattached to. Feel free to change it to whatever you prefer, including your original suggestion of replacement_sort_threshold if you still think that works. The new default value that I came up with for replacement_sort_tuples is 150,000 tuples, which is intended as a rough generic break-even point. Note that trace_sort reports how many tuples were in the heap should replacement selection actually be chosen for the first run. 150,000 seems like a high enough generic delta between an out-of-order tuple, and its optimal in-order position; if *that* amount of buffer space to "juggle" tuples isn't enough, it seems unlikely that *anything* will be (anything that is less than 1/2 of the total number of input tuples, at least). Note that I use the term "cache oblivious" in the documentation now, per your suggestion that CPU cache characteristics be addressed. We have traditionally avoided using jargon like that, but I think it works well here. The reader is not required to know the definition. Dropping that term provides bread-crumbs for advance users to put all this together in more detail, which I believe has value. It suggests that increasing work_mem or maintenance_work_mem can have almost no downside provided you don't need that memory for anything else, which is true. I will be glad to see this through. Thanks for your help with this, Robert. -- Peter Geoghegan